Understanding Multimodal AI Complexity Businesses commonly use the term “multimodal”...
Read MoreRevolutionizing Drug Discovery: Integrating papAI Solution into the Drug Discovery Pipeline
Table of Contents
ToggleDrug discovery is a difficult, drawn-out process that needs a large time and financial commitment. Researchers have historically tested tens of thousands of chemicals to find one that has the desired therapeutic effect. This process is known as trial and error. Yet current developments in artificial intelligence (AI) have completely transformed the drug discovery process, making it more rapid, effective, and accurate than ever before. According to a report by Accenture, AI can potentially reduce the time and cost of developing a new drug by up to 70%.
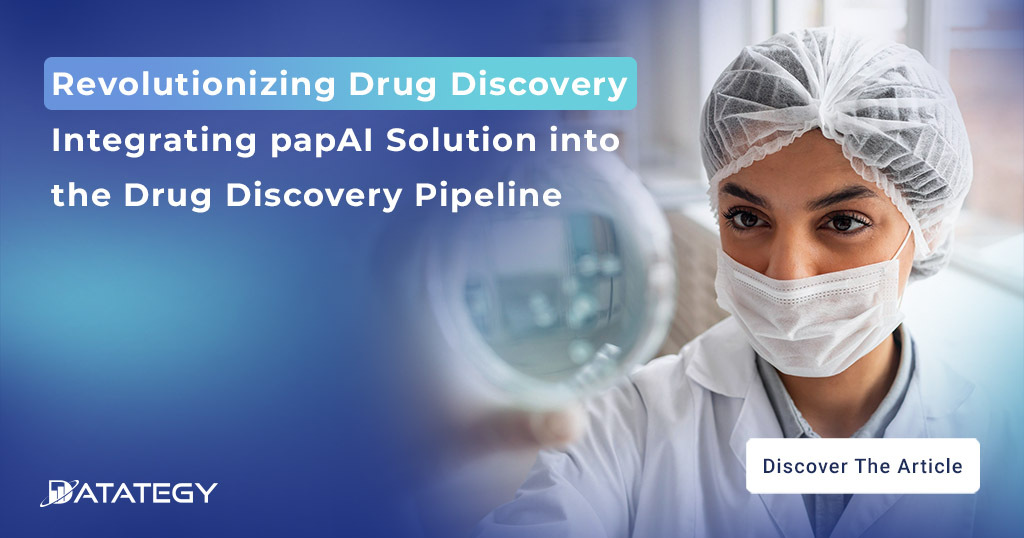
To find new drug candidates and forecast their effectiveness and safety, artificial intelligence (AI) systems can evaluate enormous volumes of data, including chemical structures, biological interactions, and clinical data. In this article, we will examine the many applications of AI in drug discovery and analyze its advantages and challenges, and the implementation of papAI solution in this process.
The evolution of drug discovery
In the past, finding new therapeutics required both fundamental study to identify potential treatments and the extraction of components from natural assets. In general, the pace of progress was laborious, irritating, and slow. Sometimes discoveries were made as a result of unanticipated occurrences and observations (such as penicillin). Small molecules are produced by chemical synthesis, which still accounts for 90% of all medications on the market today.
Biologics ( biological therapeutics ) are bigger, more complicated biological treatments that have been discovered as a result of scientific and technical advancements during the 1990s. Biologics are created or separated from live creatures as opposed to chemically synthesized tiny molecules. Examples of biologics include allergenics, antisense medicines, blood and blood components, recombinant therapeutic DNA and proteins, and vaccinations.
This infographic provides details of all the major developments in the field of drug discovery:
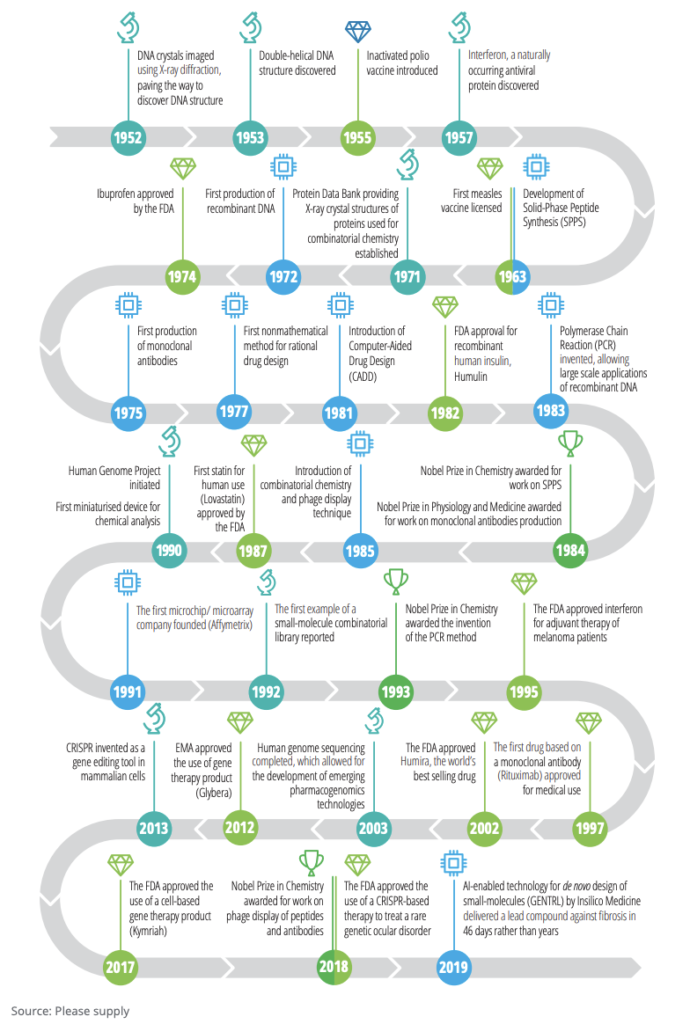
What are the benefits of using AI in this area?
1- Faster identification of potential drug candidate
By examining a variety of data sets, including chemical structures, biological interactions, and clinical data, AI can significantly speed up the process of discovering potential drug candidates. In the past, researchers have tested hundreds or even millions of chemicals in the lab using high-throughput screening (HTS) techniques to find possible therapeutic candidates. Finding a viable molecule requires years of expensive and time-consuming research.
However, with AI, researchers can scan huge data sets much more quickly and identify new medication possibilities. For example, massive databases of chemicals, their chemical characteristics, and information on their biological activity and toxicity may be used to train machine learning algorithms. These algorithms can then spot trends and forecast the chance that a specific molecule will be beneficial in treating a specific ailment.
In a study published in the journal Nature in 2018, researchers used a deep learning algorithm to identify potential drug candidates for Alzheimer’s disease. The algorithm was able to identify a number of compounds that were effective in treating Alzheimer’s in mice, including one compound that has now entered clinical trials. The researchers estimated that it would have taken 30 years and cost $2.5 billion to identify these compounds using traditional methods.
2- More precise predictions of drug efficacy and safety
AI can assist researchers in prioritizing the most promising compounds for additional testing, decreasing the chance of expensive clinical trial failures by offering more accurate forecasts of medication effectiveness and safety. This can not only save time and money but also hasten the delivery of patients’ beneficial medicines.
AI can also assist in locating potential drug adverse effects that might not be noticeable through conventional testing procedures. For instance, patient data analysis using machine learning algorithms can be utilized to find genetic or other characteristics that may raise the likelihood of negative medication responses. This can help researchers create treatments that are more individualized and less likely to cause negative effects.
3- Developing new drug targets
To find new potential therapeutic targets, researchers may use AI to evaluate enormous data sets from many different sources, such as genomes, proteomics, and metabolomics data. For instance, massive databases of genetic data may be used to train machine learning algorithms to find genes linked to certain diseases. The biochemical processes that are affected by the disease may then be predicted using these algorithms, along with proteins or receptors that might be useful as therapeutic targets.
AI may also be used to assess the results of high-throughput screening experiments to find drugs that alter the activity of particular proteins or metabolic pathways. This may make it possible for researchers to discover new, as yet undiscovered, targets.
According to a report by McKinsey & Company, AI can help to identify new drug targets up to four times faster than traditional methods. The report estimates that AI has the potential to reduce the time and cost of drug discovery by up to 50%.
Challenges and Limitations of AI in Drug Discovery
The difficulty of understanding data from complicated models is one of the main issues with employing AI in drug research. The ability to grasp how these patterns connect to particular biological processes can be difficult, despite the fact that machine learning algorithms are adept at spotting patterns in vast datasets. This might make it challenging to rank prospective medication candidates or choose which targets have the greatest promise for further investigation.
The necessity for high-quality data is another issue with applying AI in drug research. Large volumes of data are used by AI systems to train and improve their models. Nevertheless, the accuracy of the resultant forecasts depends heavily on the quality of this data. Inaccurate conclusions can be drawn from inadequate, inaccurate, or biased data, which can impede the creation of efficient treatments.
Furthermore, the quality of AI systems depends on the data they are trained on. An AI model may not be accurate in predicting pharmacological effectiveness or safety in a variety of patient groups if the data used to train it is not representative of the patient population. This may reduce the value of AI in creating individualized medical strategies that are effective for all patients.
How to choose the best AI solution for your data project?
In this white paper, we provide an overview of AI solutions on the market. We give you concrete guidelines to choose the solution that reinforces the collaboration between your teams.
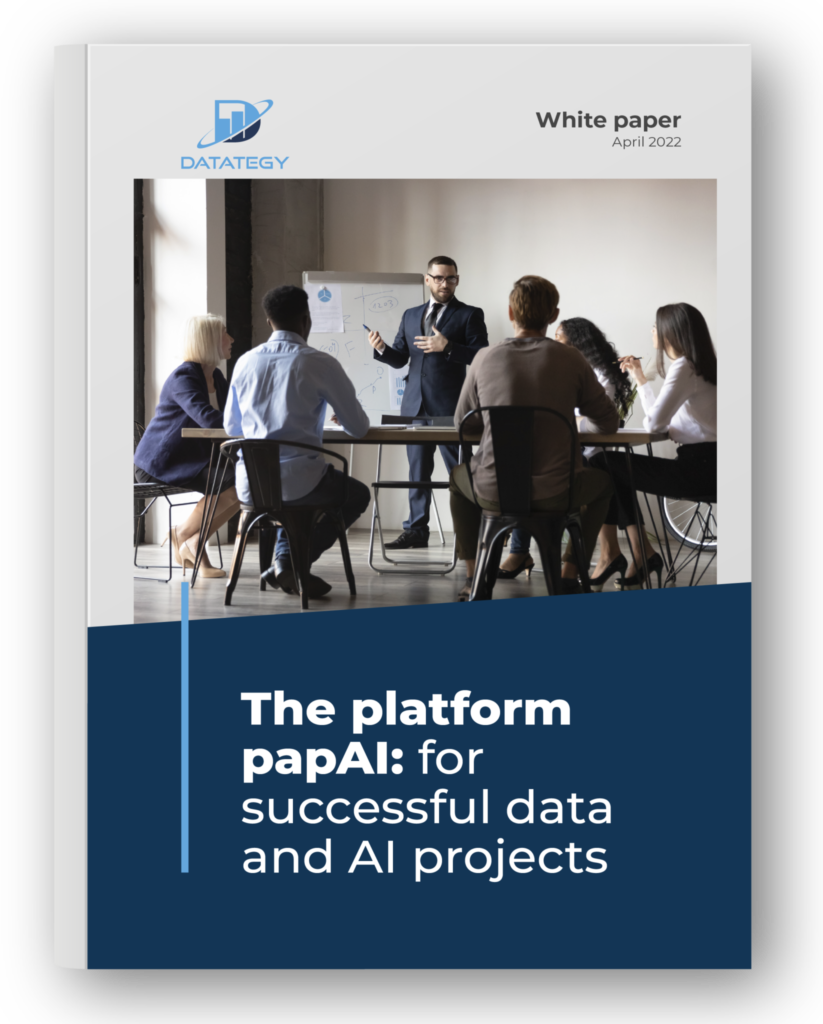
Why does using the papAI solution make a difference?
AI eliminates subjective bias and does not rely on predetermined targets
Target identification in the preclinical phases is made quicker and more precise by using AI. AI can assess all of the available data to identify the objectives that are most likely to be successful. papAI solution contains a number of measures to assess the fairness level of the model’s prediction in order to reduce the effect of gender and age bias
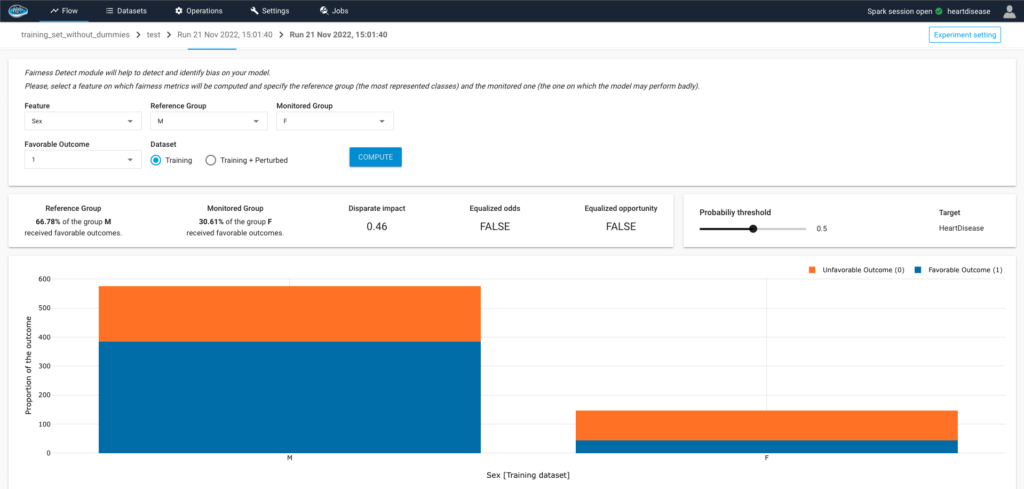
Extract meaningful information from a large dataset
Data collections for drug discovery are huge, dynamic, and varied. In addition to saving time and money for academics and pharmaceutical firms, AI can mine this data and extract critical insights that will help patients receive medicines more quickly. Moreover, with papAI’s Explainable AI, we can understand easily how a trained model works and the factors that had a major impact on the prediction’s decision-making. One such interpretable tool is the Partial Dependency plot (PDP), which helps us visualise this variance.
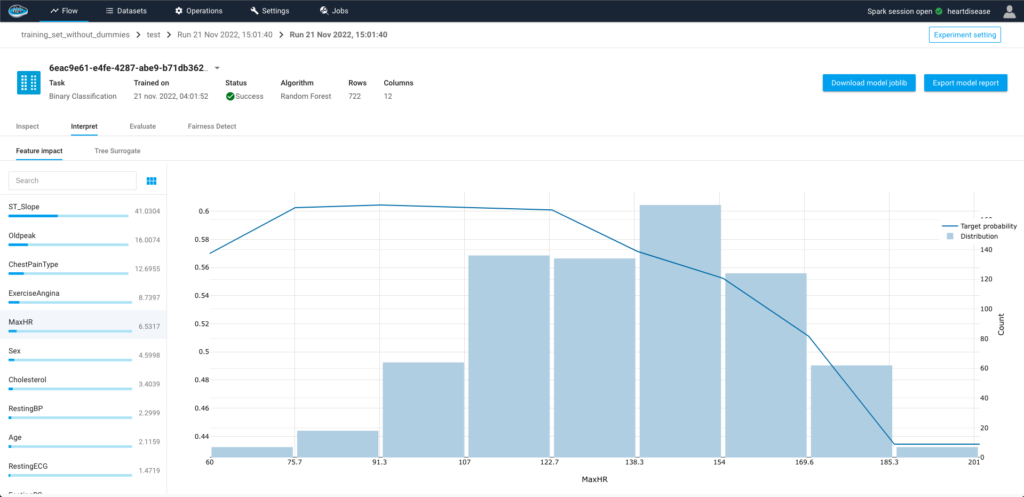
Higher predictive power reduces the risk of false positives
AI can find significant interactions in a medication screen. This reduces the possibility of sending an ineffective medicine to clinical trials by accident. For instance, one of the primary reasons why cancer treatments don’t reach the market is a lack of preclinical predictive modeling. Preprocessing methods that appropriately organize the dataset and remove class imbalances that have a significant impact on the final prediction are integrated into papAI in order to ensure that the danger of discovering false positives is reduced.
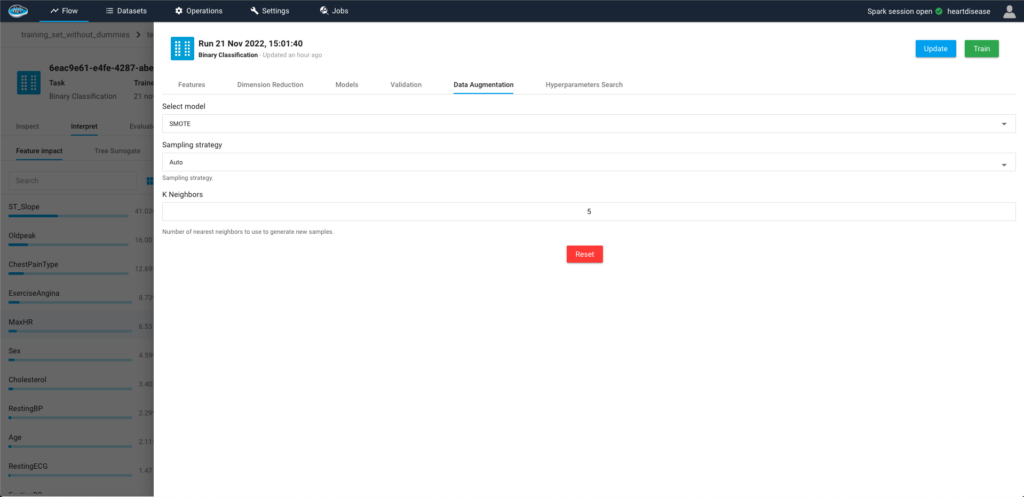
Speed up the drug discovery and development process
AI boosts efficiency across many different stages of the drug development process. Preclinically, AI improves drug target identification and predictive modeling. AI also speeds up molecule design and testing, and drug screening. papAI has been developed to help data, medical or business experts to build an intuitive and visual data science flow and fully understand and challenge the results.
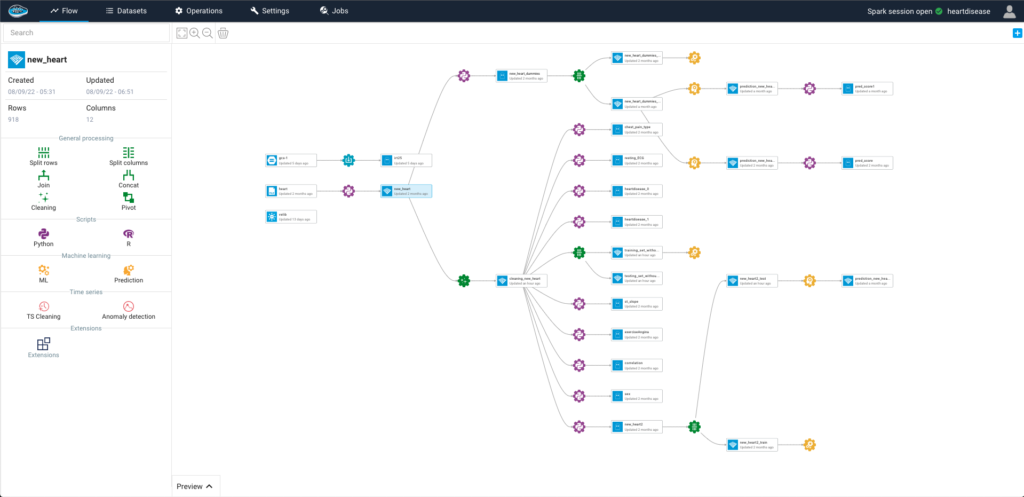
Create your own AI-based tool with the papAI solution to optimise your drug discovery process
In conclusion, AI has the potential to revolutionize the drug development process by enhancing the accuracy of therapeutic effectiveness and safety forecasts, speeding up the identification of promising drug candidates, and discovering novel drug targets. To fully harness the potential of this technology, it is necessary to recognize and overcome the challenges and restrictions of using AI in drug research.
One solution to these challenges is to use papAI solution to create your own AI-based tool for drug discovery. papAI solution helps to explain and make transparent the results through a process of data mining, cleaning, and visualization that can accelerate the deployment of AI projects and drug discovery and reduce the time and cost of bringing new therapies to market.
Book your demo now. Our team of experts can help you create a custom AI-based tool that meets the unique needs of your organization and helps you stay at the forefront of drug discovery innovation.
Interested in discovering papAI?
Our commercial team is at your disposal for any questions
How Do AI Predictive Models Estimate Car Prices?
How Do AI Predictive Models Estimate Car Prices? Valuation methods...
Read More“DATATEGY EARLY CAREERS PROGRAM” With Tommy GUYOT
“DATATEGY EARLY CAREERS PROGRAM” With Tommy GUYOT Hello! My name...
Read More