How RAG Transforms Sales and Marketing Performance In sales and...
Read MoreThe Delivery Imperative: Making AI Accessible to All
Table of Contents
ToggleDid you know that 80% of data and AI projects never make it past the MVP stage? Despite the hype around artificial intelligence, most initiatives struggle to move from proof of concept to real-world impact. The reasons vary lack of alignment between teams, unclear business value, or solutions that never reach operational scale.
At Datategy, we believe successful delivery is where the true value of AI is realized. It’s not just about building a smart algorithm; it’s about making sure it solves the right problem for the right people in the right way.
In this interview, Hermann, our Head of Project Delivery, shares how his team ensures that every project at Datategy isn’t just delivered but adopted, scaled, and impactful. From aligning cross-functional teams to managing expectations and measuring success, Hermann opens up about what it really takes to move beyond MVP and make AI operational.
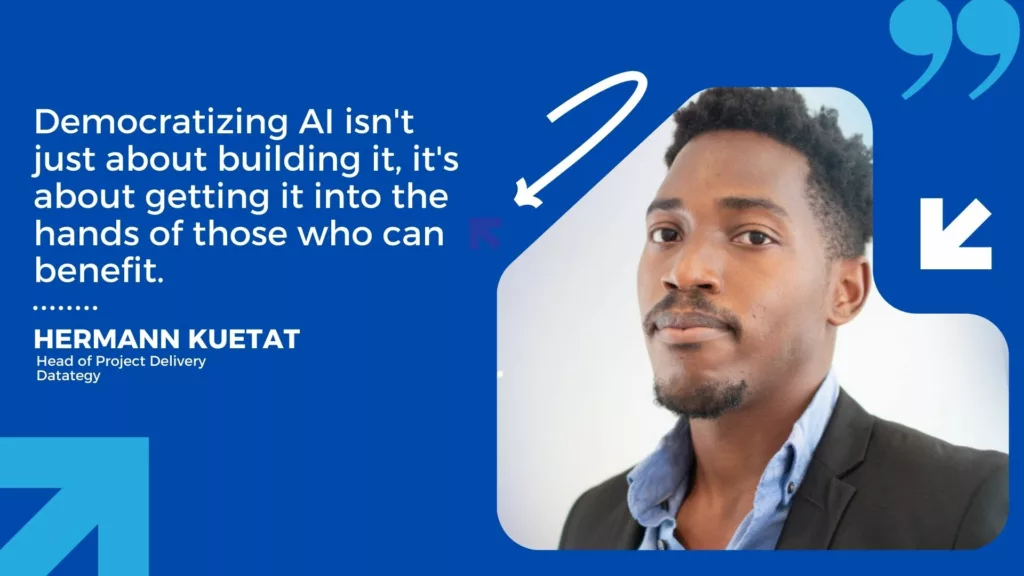
Could you introduce yourself?
Hello, my name is Hermann.
I’ve always been driven by a desire to solve real-world problems. That’s what led me to study engineering in the energy sector. But during my final internship, I had a real eye-opener: I discovered how powerful data could be not just in one industry, but across the board. The idea that data could be used to generate insights, guide decisions, and ultimately improve performance fascinated me. So I made a shift. I trained to become a data expert and started my career as a data analyst in the energy field, then spent several years as a data engineer in banking.
Today, I’m the Head of Project Delivery at Datategy. My mission is to ensure that every AI project we deliver doesn’t just work technically, but also brings real, measurable value to our clients. I see myself as a key enabler of Datategy’s vision of democratized AI. That vision becomes reality with each successful delivery, we’re not just building software; we’re empowering people to make better decisions with AI.
How is the delivery team structured and what are its main tasks?
The delivery team is built for agility. We have project managers, data scientists, and solution engineers who work together as dedicated squads, depending on the size and complexity of the project.
Our tasks span from the initial scoping phase to deployment, training, and continuous support. What makes us strong is our ability to adapt our approach to the client’s level of maturity, whether they’re just beginning with AI or already managing complex use cases.
What are your priority objectives for the next 12 months?
We’re scaling up, so one key goal is to streamline and standardize our delivery processes without losing the custom-fit approach our clients appreciate. At the same time, I want to strengthen the feedback loop between the delivery and product teams.
Real-life projects (RATP, Alstom, Renault Group, Société des Grands Projets, etc.), give us the best insights into how our platform should evolve. Another big focus is building internal knowledge and mentoring newcomers, we want to grow as a team while staying aligned with our core values: excellence, pragmatism, and client-centricity.
How does the delivery team interact with Datategy's product, tech, and sales teams?
It’s a close and ongoing collaboration, a constant feedback loop that shapes our direction. With the sales team, we’re involved early—often during pre-sales—to meticulously assess client requirements and ensure the proposed AI solutions are not only innovative but also practically achievable and deeply aligned with the client’s specific business objectives and technical infrastructure.
This early engagement allows us to proactively identify potential roadblocks and set realistic expectations from the outset. On the tech and product side, our feedback is essential; it’s grounded in real-world application and client interaction. We’re the ones who witness firsthand how features perform in diverse operational environments, understand the specific challenges clients encounter during implementation and ongoing use, and recognize the aspects of our platform that truly resonate and deliver significant value.
This continuous, direct interaction with the deployed technology and end-users ensures we’re not just iteratively improving both our service offerings and the underlying AI platform, but also strategically evolving them based on tangible insights and client success metrics. One of the most valuable aspects was learning the full lifecycle of an AI implementation product—from data ingestion and processing, to building pipelines for training and testing, through to model deployment.
What are the key stages in a typical project managed by the delivery team?
It starts with a kickoff where we define the goals, success metrics, and timeline. Then we move into solution design and iterative implementation, often in short sprints to deliver value quickly.
Training and onboarding come next we don’t just hand over a tool, we ensure users are confident using it. Finally, we assess the impact and, if needed, help the client scale to other use cases or teams.
How do you measure the success of a project delivered to a customer?
Success isn’t solely about adhering to timelines and budgetary constraints, though those are undeniably critical indicators of efficient execution. True success, in our view, hinges on the tangible impact and sustained utilization of the delivered solution within the client’s operational context.
Did our AI implementation empower individuals to make demonstrably faster and more insightful decisions, leading to measurable improvements in their workflows? Were end-users able to seamlessly integrate the platform into their daily routines, adopting it with genuine confidence and realizing its intended benefits without significant friction or resistance? When we can confidently answer yes to these questions, that’s a clear indication of a successful project.
Furthermore, our commitment extends beyond the initial deployment; we proactively engage with our clients a few months post-implementation to rigorously measure the long-term impact of our solutions, tracking key performance indicators and gathering qualitative feedback to ensure sustained value generation and identify areas for continuous improvement.
What tools or methodologies do you use to monitor the progress and quality of deliveries?
How do you manage customer expectations vs. internal constraints (technical, deadlines, etc.)?
Interested in discovering papAI?
Our AI expert team is at your disposal for any questions
The Delivery Imperative: Making AI Accessible to All
The Delivery Imperative: Making AI Accessible to All Did you...
Read MorepapAI Academy : Uzo C Okoye
papAI Academy : Uzo C Okoye papAI Academy is a...
Read MoreHow Law Firms Use RAG to Boost Legal Research
How Law Firms Use RAG to Boost Legal Research RAG...
Read More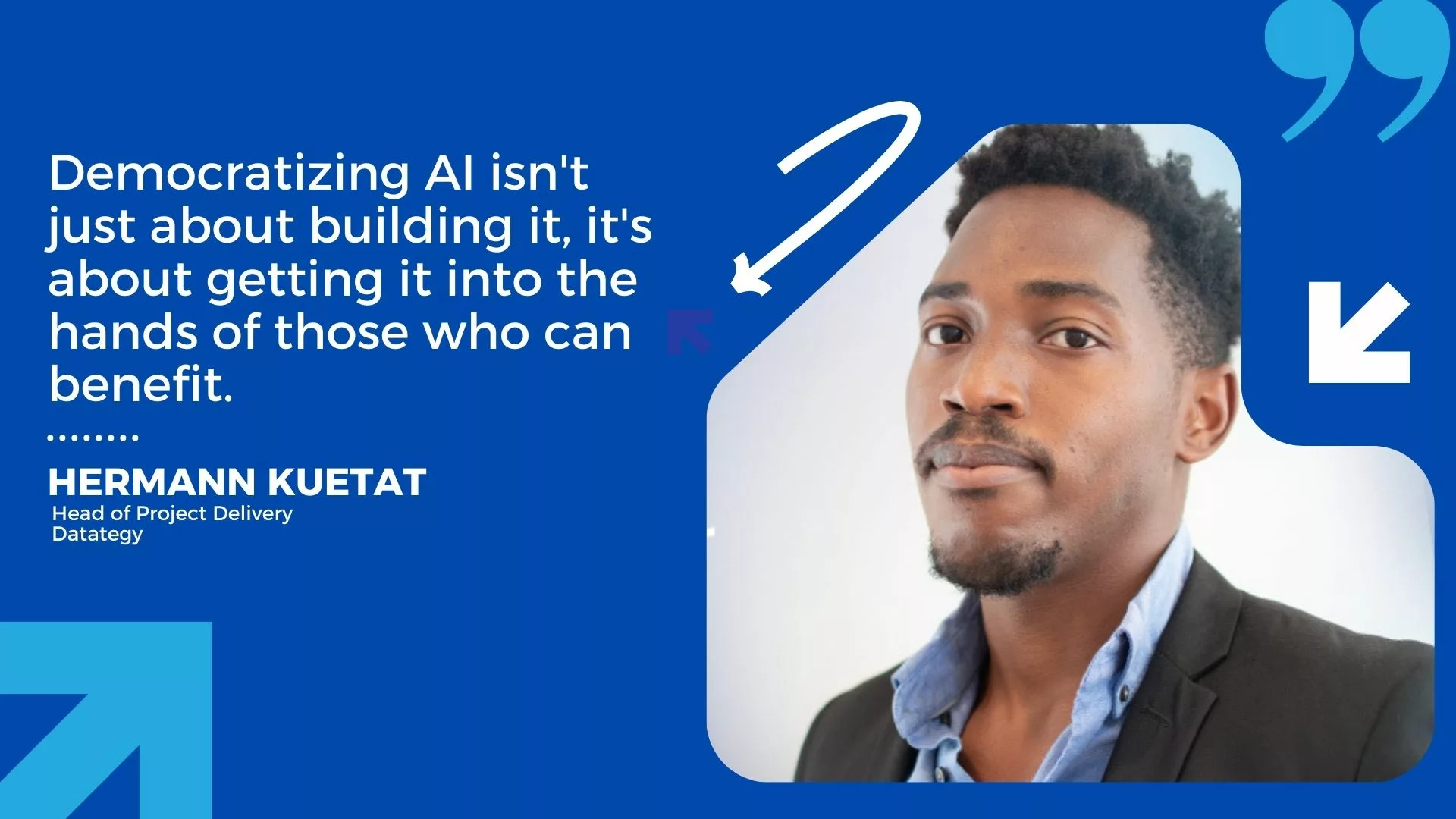
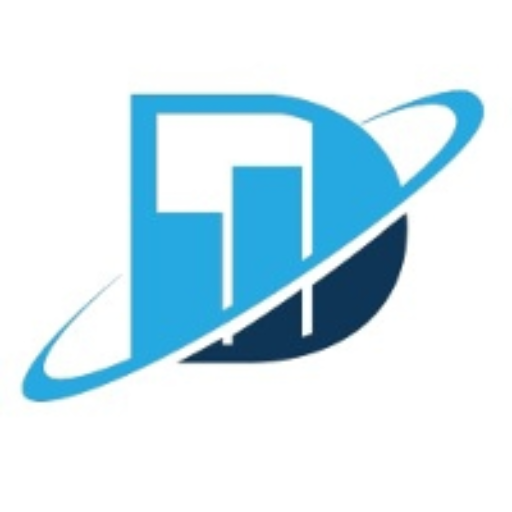