Transform Defense Logs & Rapport into Situational Awareness with AI...
Read MoreData projects: the main challenges
for the Chief Data Officer
Table of Contents
Toggle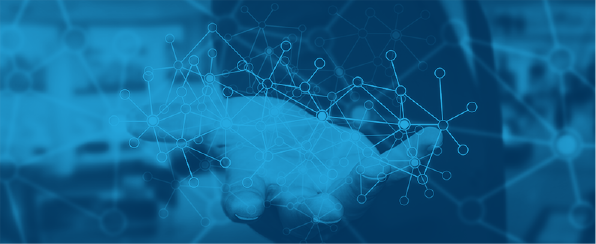
According to Gartner, 85% of data projects end in failure. This is mainly due to the quality of the data. Ensuring good data quality to make it usable is therefore the main task of the Chief Data Officers (CDO).
However, 31% of the CDOs surveyed by IBM believe that the increasing complexity of data, as well as the compartmentalisation between departments and people in the company, are real obstacles and hinder the use of data to build relevant models.
These are not the only challenges they face. When it comes to Artificial Intelligence projects, CDOs again face many obstacles:
- 37% of CDOs say that the lack of expertise and knowledge in AI is a barrier to its adoption.
- 26% consider that they lack dedicated tools, which prevents them from developing projects.
In this article, we take a closer look at these barriers and how to overcome them.
Barrier #1 - Lack of strategy in data governance
It is no secret that without optimal governance and quality of data, any process aimed at exploiting it and extracting value from it, is useless. By data governance we mean all the organisations and procedures put in place to manage the collection, organisation and use of data. The aim is to provide employees with the best possible conditions for analysing and using data.
However, this governance is still complicated for many organisations. CDOs are confronted with a lack of data consistency from one site to another, or even from one business line to another. They are also faced with “dark data”, data that is ignored either because of shadow IT or because no one even knows it exists. It is therefore necessary to make an inventory of all this data in order to know whether or not it is being exploited, how and why.
The SNCF has handled this issue very well: the company has set up Data Owners who monitor indicators linked to data quality. In the event of a quality discrepancy, they warn and implement an action plan to resolve the problem. At the same time, a Data Quality expert on the business side is responsible for building dashboards to help the business solve quality problems more easily.
Barrier #2 - Lack of collaboration with the trades
According to a study by McKinsey (“Notes from the frontier: modelling the impact of AI on the world economy”), artificial intelligence could add $13 trillion to global GDP by 2030. So the benefits are clear. While the CDO and their teams are convinced, it is still difficult for employees in the various branches of a company to understand the value that AI brings. It is this lack of collaboration between the business and the CDO that can slow down the development of projects.
AI requires a lot of “test and learn”, an agile approach and strong cooperation between business teams and data scientists. It is necessary to get employees on board to capitalise on the technical and analytical know-how of some, and the precise knowledge of business processes of others.
To do this, training and evangelisation of business teams are key: employees must be reconciled with AI, who sometimes see it as a threat to their functions. This stage enables them to discover how artificial intelligence will facilitate their missions, their decision-making, make them more efficient, better target their actions, and possibly relieve them of tedious tasks.
Barrier #3 - Lack of dedicated human resources
A study conducted in 2019 by IDC reveals that half of AI projects are doomed to failure, mainly due to a lack of competent resources. Data profiles such as Data Scientists, CDOs, Data Analysts, Data Architects… are in demand by companies and are still too few in number. Dedicated training courses are developing, but qualified profiles are spoilt for choice in terms of employers.
Another skill that can be lacking and lead projects to failure is managers. Indeed, it is not enough to surround oneself with technical profiles to successfully carry out a mission of data valorisation. Project management profiles are also key, in order to understand the project, the strategy and to define priorities, deadlines, costs and ensure the steering. Many projects end in failure, not because of a lack of technical skills, but because of a lack of management skills.
Barrier #4 - The difficulty of industrialising Artificial Intelligence models
CDOs are also faced with the challenge of making the most of AI models and making them profitable. Without the right tools and a real strategy, putting AI projects into production can be complex. According to a study conducted by Algorithmia, 50% of companies spend between 8 and 90 days deploying a single AI model. This is due to the difficulty of replicating basic models, a lack of team involvement, or the difficulty of deploying algorithms at scale.
At the same time, problems specific to the production launch sometimes emerge without having been detected beforehand and cause the deployment to fail. Linked to the lack of human resources, companies do not always have the internal skills to solve these problems or to make the deployment a success. Finally, there is often a lack of strategy, methodology and industrialisation of artificial intelligence models.
The lack of collaboration and understanding of the issues between the business and data teams can also have an impact on the industrialisation of models: as the project does not meet expectations, the business does not wish to use the resources made available and developed by the data teams. Evangelisation and communication work is therefore necessary to avoid wasting time and energy deploying useless processes.
However, certain production difficulties can be anticipated. Major technical pitfalls likely to impact deployment will be overcome if the AI analysis platform is interfaced with the company’s internal tools (BI for example) from the outset or if it is already integrated into the cloud where the data is stored.
CDOs must therefore overcome these various obstacles to be able to take advantage of all the benefits offered by data. Before thinking about “mass” artificial intelligence, it is necessary to work on the quality of the data, the collection, the strategy and industrialisation of projects, the collaboration between the business and the data teams, and finally the recruitment of the necessary resources.
Recommended articles
Why AIOps Is Key to Cyber Threat Detection in Defense?
Why AIOps Is Key to Cyber Threat Detection in Defense?...
Read MoreHow AI Transforms Predictive Maintenance in Defense Equipment
How AI Transforms Predictive Maintenance in Defense Equipment In a...
Read More