AI technology: how to use it in your data projects?
Table of Contents
ToggleDigital transformation, Big Data, Automation… In a world where digital technology plays a bigger role, companies are collecting and storing astronomical amounts of data. However, exploiting it correctly is a major challenge.
AI technologies are currently considered the rising star of data analysis due to their almost limitless potential. But as the saying goes, “Power is nothing without control”. Harnessing data to drive operational meaning requires both a structured approach and informed technology choices.
Why is data preparation so important? Which AI technology should be preferred? Why is it essential to use dedicated artificial intelligence platforms? Find out how to take your data projects to the next level.
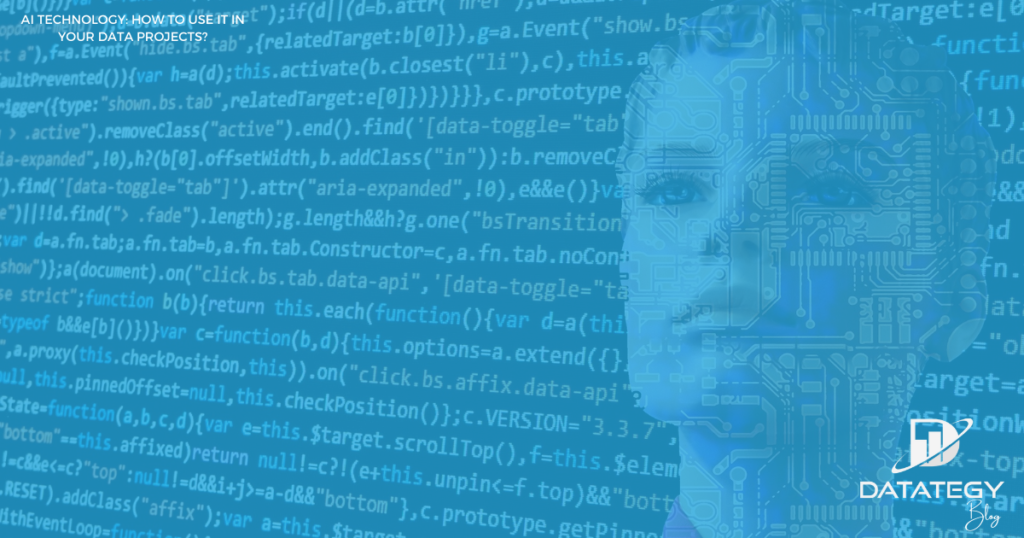
AI technology: the importance of data preparation.
While AI is used in many application today, it is important to keep in mind that the term “Artificial Intelligence” does not (yet) go together with “Total Automation”, regardless of the technology used.
For every data project that you have in mind, a large portion will be dedicated to data preparation, a process that is mainly done manually. A recent study by Cognilytica showed that data preparation accounts for an average of 80% of the time spent on AI projects.
The reason for this is based on three points:
- Qualitative and quantitative standards to be respected
It is imperative to have a sufficient volume of data available to properly feed the AI technology.
In the same way, the quality of the information must also be checked to avoid starting a project on the wrong basis.
It is not uncommon that the volume and quality of usable data are overestimated by the project stakeholders. The preparation phase is therefore crucial as it acts as a safeguard.
- Strong consistency issues
With the multiplication of formats and the permanent growth of dematerialised storage systems, it is common for data to be altered during its lifetime, or even from the moment it is created.
Beyond quality, it is therefore the overall consistency of the information that must be checked: are all data of the same nature in the right format? Have duplicates been identified? Is it possible to standardise information of the same type from different sources?
- The necessary visualization of data
To begin to verify the two points discussed above, it is necessary to be able to visualize the data in a concrete and synthetic form, using data visualization.
They allow a trained eye to quickly spot information and irregularities thanks to the graphic representations, whereas the analysis of raw data would be much more complex and time-consuming.
As we have seen, data preparation is not a simple routine and takes time to complete. But once everything is ready, which artificial intelligence technology should be used for analysis?
Machine learning, the AI technology to choose for your data projects
Today, Artificial Intelligence, in the broadest sense of the word, encompasses several application sub-domains: Machine Learning, Deep Learning, Neural Network, Natural Language Processing (NLP)…
However, in the context of a data project, one AI technology stands out: Machine Learning. This approach has proven itself for predictive analysis, and continues to gain power with the growing volume of data globally (more occurrences and therefore a better learning curve).
But what are the main advantages of this artificial intelligence technology?
- Firstly, it is the almost infinite learning capacity of Machine Learning that sets it apart from other AI models. Over time and with the data presented to it, the system gains experience and knowledge. It can then use this added (and accrued) value to make better decisions or present more accurate predictions/trends that it may not have been able to identify before.
- The other great strength of Machine Learning is its ability to quickly analyse large volumes of information and then identify recurring trends and patterns that are difficult for a human to spot.
- It should also be noted that Machine Learning is an AI technology that can evolve on its own, without human intervention (with considerable time savings). Once it is provided with data, the algorithm is able to operate autonomously, but also and above all to adapt to new and unforeseen events in record time (an excellent example being anti-virus software which uses it to detect new infection risks).
- Finally, it is an artificial intelligence system capable of efficiently processing large amounts of data with multidimensional structures.
Why should you opt for an artificial intelligence platform?
The use of “Big Data” over the past decade has continued to grow: companies around the world now have the material to use artificial intelligence to optimize their decision-making. However, the use of AI technology within a data project is often a complex and time-consuming exercise, including for data science professionals. Exploiting its full potential can therefore be particularly difficult. In this context, a dedicated artificial intelligence solution can make the difference. AI platforms offer a shared view of data processing, powerful preparation tools and numerous dedicated “ready-to-use” algorithms capable of providing short-term results, gain time and increase RoIs.
Best of all, AI platforms are typically packaged as “toolboxes”: many individual building blocks can be selected, assembled, and modified at will by users to create custom workflows.
At Datategy, our papAI platform also makes artificial intelligence accessible to the widest possible audience, thanks to a low-code approach and easy-to-understand visual interfaces. These facilitate the appropriation and democratization of AI for businesses. Moreover, the SaaS model is particularly relevant today to facilitate the connection of an AI platform to the various data sources owned by the organization.
Finally, solutions dedicated to artificial intelligence such as papAI give companies the possibility to industrialize the management of their data projects for specific use cases: duplication of assets, repurposing of workflows without code… the possibilities are numerous. A clever mix of methodology and tools, the use of AI technologies in your data projects requires rigor and patience.
Fortunately, AI platforms provide excellent support for data scientists, as they do not have to develop specific solutions for each new project. Thus, they can spend more time “making the data talk” than creating the required infrastructure: industrialisation is underway!
Interested in discovering papAI?
Our commercial team is at your disposal for any question