Understanding Multimodal AI Complexity Businesses commonly use the term “multimodal”...
Read MoreAIOps advanced IT operations management
Table of Contents
ToggleOrganizations throughout the world are increasingly looking to automation technologies as a means of improving operational efficiency, This indicates that tech leaders are becoming always more interested in MLOps and AIOps. One emerging approach, AIOps, promises to apply artificial intelligence to fexing IT problems.
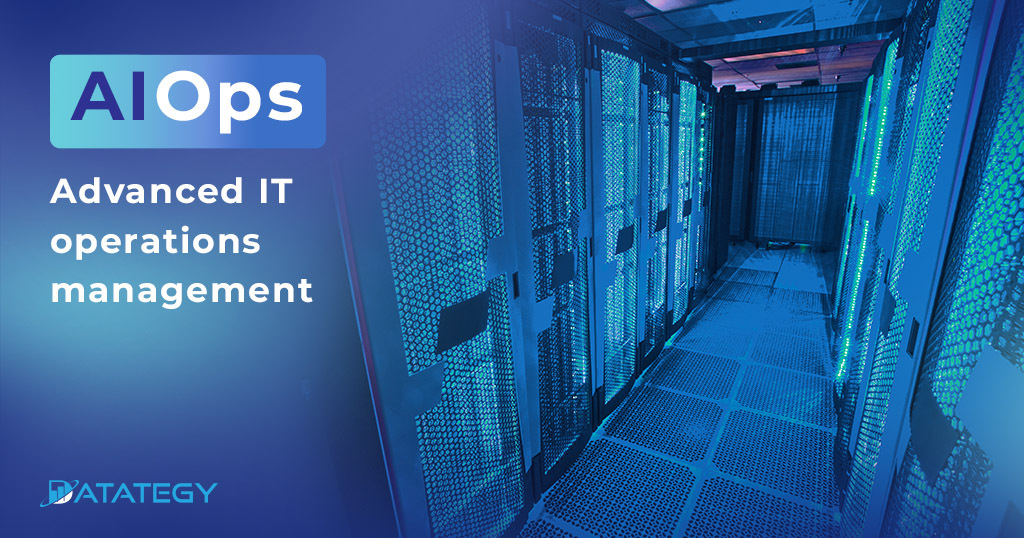
What's AIOps ?
Conceptualized by Gartner in 2017, the notion of AIOps (for artificial intelligence operations systems) refers to monitoring tools that combine artificial intelligence (AI) and Ops, IT operations management. In other words, AIOps puts AI technology at the service of IT production. This field covers various dimensions of Ops, from monitoring to application maintenance of information systems.
How AIOps platfrom works ?
Data collection and aggregation: AIOps begin by collecting and aggregating data from multiple sources, such as IT infrastructure applications, components, and monitoring tools.
Data analysis: It involves analyzing data in real-time at the ingestion point, It also includes analyzing historical data stored on your systems.
This aims to identify significant patterns and events related to network and system availability issues and performance.
Reports and diagnosis: After detecting the issues, AIOps platforms initiate actions immediately based on the gained insights. They can even diagnose issues without requiring human intervention, It helps address the root causes and report them to concerned IT teams for rapid response.
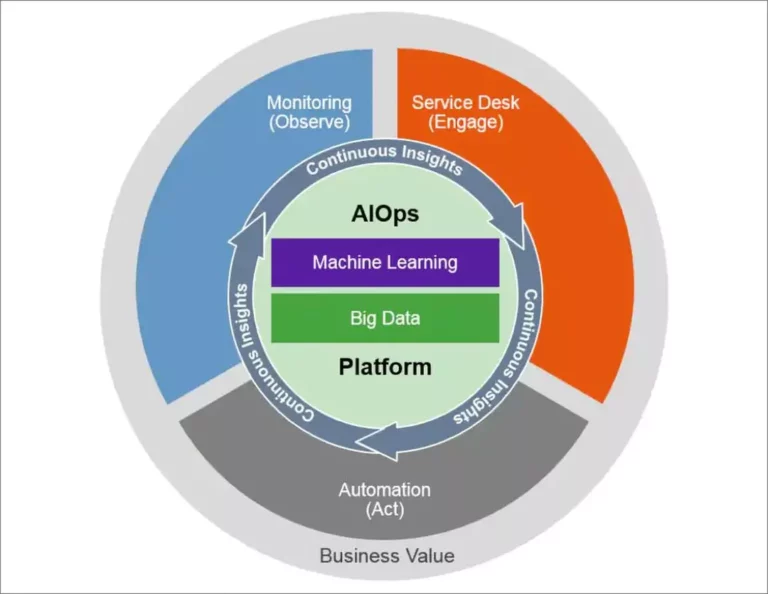
What's the difference betwen MLops and AIOps ?
While MLOps and AIOps are entirely separate disciplines involving various technologies and procedures, machine learning and artificial intelligence play a significant role in aiding businesses in achieving operational efficiency. Most importantly, they accomplish distinct objectives.
By automating incident management and machine diagnostics using machine learning, AIOps improves the effectiveness of IT operations.
Putting models into production more quickly makes it simpler to bridge the gap between data operations and infrastructure teams. Putting machine learning models into production is known as MLOps. MLOps doesn’t specifically refer to a machine learning capability, unlike AIOps.
What are the main use cases for AIOps ?
Identify the origin of an anomaly: In today’s multicloud or hybrid environments, it’s extremely difficult to identify the underlying event that caused the incident, The main problem with root cause analysis is gathering logs, metrics and traces that occur in the same time frame across the stack. AIOps helps shed light on the origins of anomalies. An AIOps solution also helps show the timeline of the incident from the moment it occurred.
Improved capacity planning and resource utilization: With AI-assisted, data-driven mapping, you can deploy workloads to the right combination of servers, instances and machines, If a specific combination hasn’t worked, you can adjust it in real time and continue to make changes in real time as well until it works as expected, without manual intervention.
Ability to correlate events: AIOps can play a role that allows you to aggregate related telemetry information – logs, metrics and traces.It allows you to look at associated telemetry information from various tools, all together, on the same dashboard at the same time, which will give you a clear view of what’s going on in the system and help identify the root cause fairly quickly.
papAI , A solution for IT problems
AIOps is a practical and necessary choice among a range of next-generation computing solutions, and papAI is a solution that addresses your needs.
For example, model performance slippage is a recurring problem in production machine learning environments. Companies are realizing that, unlike regular software, machine learning systems require robust risk mitigation processes.
papAI provides alert systems that identify bias and model drift. It also includes counterfactual explanations, an important emerging technique that provides hypothetical scenario analysis for model interpretability, you can book a personalized demonstration of the “papAI” platform features here.
Conclusion
The global AIOps market has grown from $4.58 billion in 2021 to $5.81 billion in 2022 at a compound annual growth rate (CAGR) of 26.76%. The AIOps market is expected to grow to $15.10 billion in 2026 at a compound annual growth rate (CAGR) of 26.95% (source AIOps Global Market Report 2022).
Interested in discovering papAI ?
Our commercial team is at your disposal for any question.
How Do AI Predictive Models Estimate Car Prices?
How Do AI Predictive Models Estimate Car Prices? Valuation methods...
Read More