Why AIOps Is Key to Cyber Threat Detection in Defense?...
Read MoreTowards a Greener Future: The Role papAI platform in Predicting Energy Consumption
Table of Contents
ToggleIn the modern world, energy consumption is a crucial problem. Finding solutions to optimize energy use is becoming more and more important due to the rising energy demand and the associated rise in energy prices. The application of artificial intelligence (AI) to forecast energy usage offers a viable answer to this issue. Energy management and consumption might be revolutionized by this technology, which would benefit all stakeholders—people, corporations, and governments—by offering insightful information and reducing costs.
Find out how papAI can improve the deployment of AI projects in the Oil and Gas Industry.
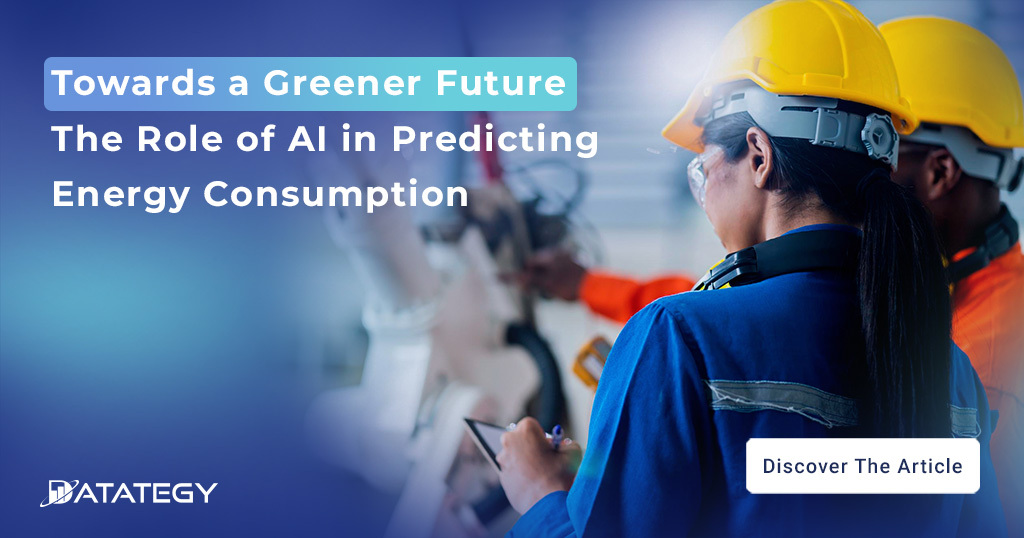
The application of machine learning and other cutting-edge computing techniques to analyze vast volumes of data in order to uncover patterns and correlations that may be utilized to generate predictions about future energy consumption constitutes the use of AI in forecasting energy consumption. These forecasts may be used to guide energy management methods, such as optimizing energy consumption, locating possibilities for energy savings, and predicting future energy demand.
Overview of The Energy Progress
The Energy Progress Report evaluates progress made toward the worldwide goal of ensuring that everyone has access to reasonably priced, dependable, sustainable, and contemporary energy by 2030. The world is still not on schedule to complete the Sustainable Development Goal 7 (SDG 7) objectives by 2030 at the current rate of development. Particularly in the most vulnerable and already trailing nations, progress has been stifled.
Innovative policies and technologies continue to emerge and bring benefits to the energy sector, but the impact of the COVID-19 pandemic set progress back in ways that were not foreseen in 2019. Not only is the world not on track to reach SDG 7 under current and planned policies, but some targets are also even farther away than they were.
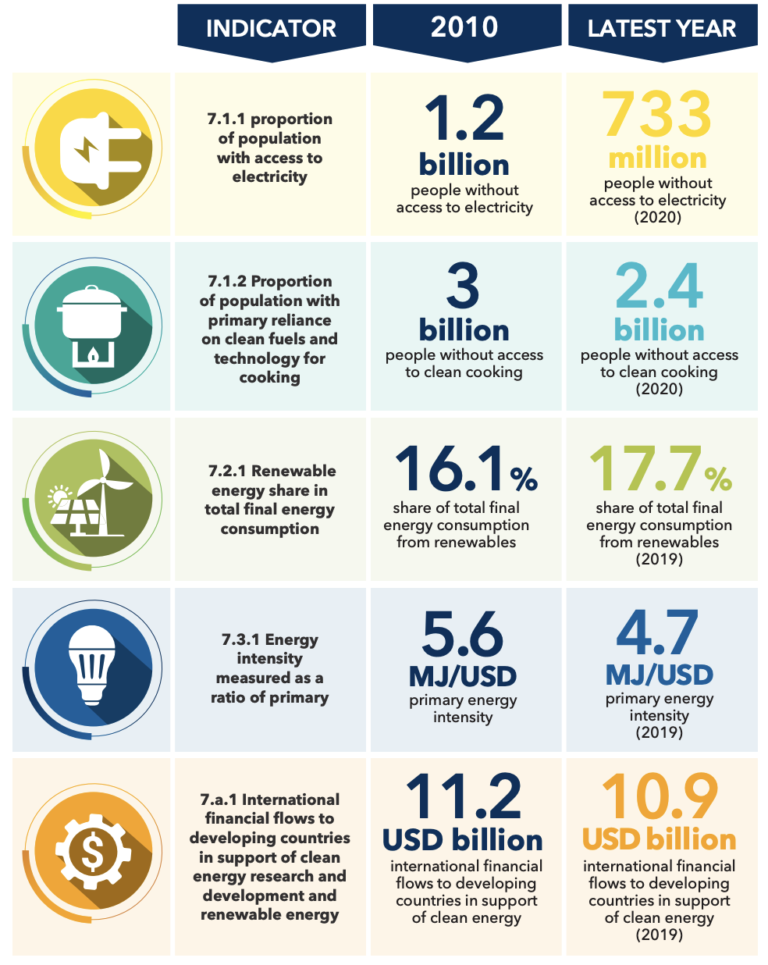
The recent expansion of electricity availability in Africa is being undone. In 2020, the number of individuals without access to electricity grew after dropping during the previous six years.
The perceived risk of lending money to a number of developing nations has drastically grown at the same time, making it more expensive for such nations to acquire debt financing for energy technology and expand access to electricity.
AI Techniques for Energy Consumption Prediction
AI techniques such as machine learning, deep learning, and neural networks can be used to analyze vast amounts of data and identify patterns that can be used to make accurate predictions about future energy consumption. In this section, we will explore these AI techniques and their potential applications in predicting energy consumption:
1- Machine learning
The creation of algorithms that can learn from data and make predictions or judgments based on that data constitutes the field of machine learning, a branch of artificial intelligence. In order to anticipate future energy use, machine learning algorithms can be trained on data regarding past energy usage patterns, weather patterns, occupancy patterns, and other pertinent elements. With the help of this method, it is possible to gain insightful knowledge about the patterns of energy consumption and create more potent energy management plans.
2- Deep learning
Deep learning is a type of machine learning that uses artificial neural networks to analyze data and make predictions. Deep learning algorithms can be used to analyze data from a wide range of sources, including energy consumption patterns, weather patterns, and occupancy patterns, to make predictions about future energy consumption. The use of deep learning in predicting energy consumption has the potential to provide highly accurate predictions, and has been shown to be effective in a range of applications, including demand forecasting and energy management.
3- Neural networks
A form of machine learning algorithm known as a neural network is based on the structure and operation of the human brain. Based on the correlations between many elements, neural networks can be used to assess complex data and forecast future energy use. These predictions have the potential to be quite accurate.
The Benefits of AI in Predicting Energy Consumption
1- Improved Accuracy of Predictions
Increased forecast accuracy is one of the main advantages of utilizing AI to anticipate energy usage. Huge data sets can be analyzed by AI systems to find patterns that can be used to predict future energy use with accuracy. Organizations may be better able to comprehend patterns of energy usage and make more educated decisions about energy management as a result of the projections’ increased accuracy. According to a study by the National Renewable Energy Laboratory, the use of machine learning algorithms can lead to an improvement in prediction accuracy of up to 20% compared to traditional prediction methods.
2- Reduced Energy Costs
The ability to lower energy expenses is a significant advantage of utilizing AI to estimate energy usage. A report by Accenture estimates that the use of AI in the energy sector could result in energy savings of up to 40% by 2035. AI can assist firms in creating more effective energy management strategies and taking action to save energy expenses by giving them more precise forecasts about future energy consumption. AI may be used, for instance, to spot trends in energy use that point out areas where energy consumption can be cut back on and to forecast energy use in the future that can be used to optimize energy use and cut expenses.
3- Improved Energy Efficiency
AI-based energy consumption forecasting can assist businesses in increasing their energy effectiveness. AI can assist organizations in identifying areas where energy usage can be optimized and in taking actions to increase energy efficiency by giving them insights into energy usage patterns. AI may be used, for instance, to spot trends in energy use that point to places where consumption can be cut back on and to forecast future energy use, which can then be used to optimize energy use and boost efficiency.
4- Improved Energy Security
AI-based energy consumption prediction can also help to improve energy security. By providing organizations with accurate predictions about future energy consumption, AI can help organizations to plan and prepare for changes in energy demand, and take steps to ensure that they have a secure and reliable energy supply. This can help to reduce the risk of energy shortages and to ensure that organizations are better able to respond to changes in energy demand.
5- Supports a Cleaner Environment
Energy conservation helps to reduce greenhouse gas emissions, which contribute to global warming and climate change. By reducing energy consumption, individuals and organizations can play a role in reducing their carbon footprint and help to create a cleaner environment for future generations.
Using papAI solution to forecast energy consumption
Context
The study of the consumption trend is important to estimate the necessary quantity to produce and distribute to the population while avoiding having excess or not enough energy which could be constraining. However, through our energy consumption use case, we are going to show you the efficiency of ML models to predict this trend and help energy providers into better organization of their production and all of this through our papAI platform.
1- Importing the dataset
First of all, you need a baseline a data that gathers information about a load of energy consumed. This is why we are using, for this use case, the hourly power consumption from the company PJM that serves the Northeastern states in the US with electricity. In order to use this dataset in the papAI platform, we need to import it from a data source. Thanks to its different connectors, you can import any dataset from different sources, either from your local machine, an external DB, or an API. After selecting the data source where your dataset is located, you just simply select the desired dataset and tune up some settings according to your liking and it will be shown in your project ready-to-use for any operation available.
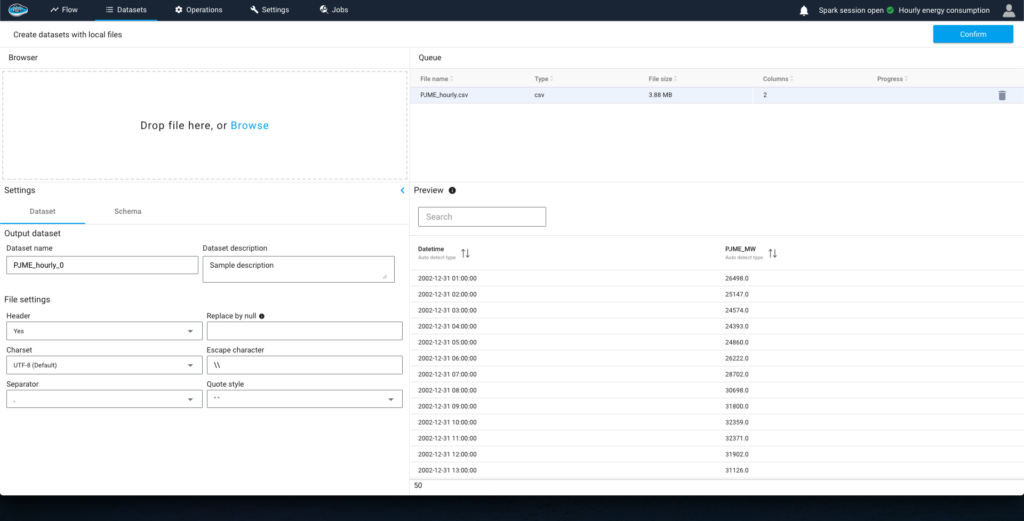
2- Explore and process your dataset
Right before going into the ML part, we need to explore patterns we can detect that can help us determine the baseline for the pipeline to train but also incoherences that we need to fix in order to ensure the best result possible for the model training. In our case, we have a case of seasonality and trend that is followed each year but we are going to select the 2017 period only and predict for 2018. After doing some feature engineering, we obtain this dataset ready to be trained.
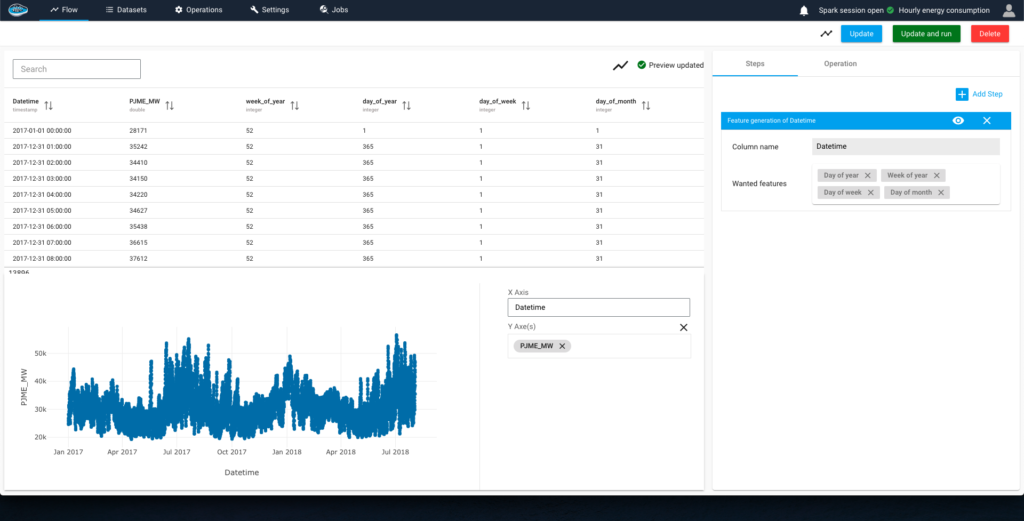
3- Build your own ML pipeline
After preparing the dataset for the training, you can develop your own ML use case to train models and test them out to monitor their performances and choose the most suitable one for your business. In order to train the model, we need to tune up the pipeline thus using the AutoML module that helps you build it easily and effortlessly with our built-in algorithms and multiple training settings. In our case, we have a use case of time-series forecasting where we undergo a sanity check before accessing the experiments to verify the sampling of the DateTime points before running the experiments. After that automatic check, you can test some experiments and tweak up some settings to forecast the desired period.
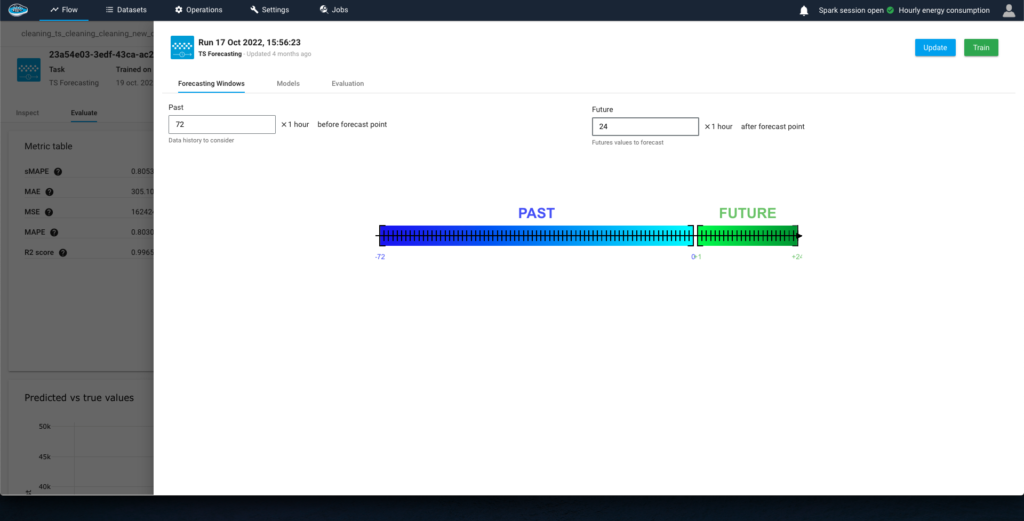
4- Evaluate the forecasting aspects
When the runs are successful, you can check how well the model has been forecasting and if it follows the same pattern. This monitoring can be done in the Evaluation module. Through it, you can check the metrics related to the prediction made by the model, the error ratio and a graph representing the real and predicted data. All of these tools can be useful to make a decision of choosing the right model hence deploying it into a real-life use case.
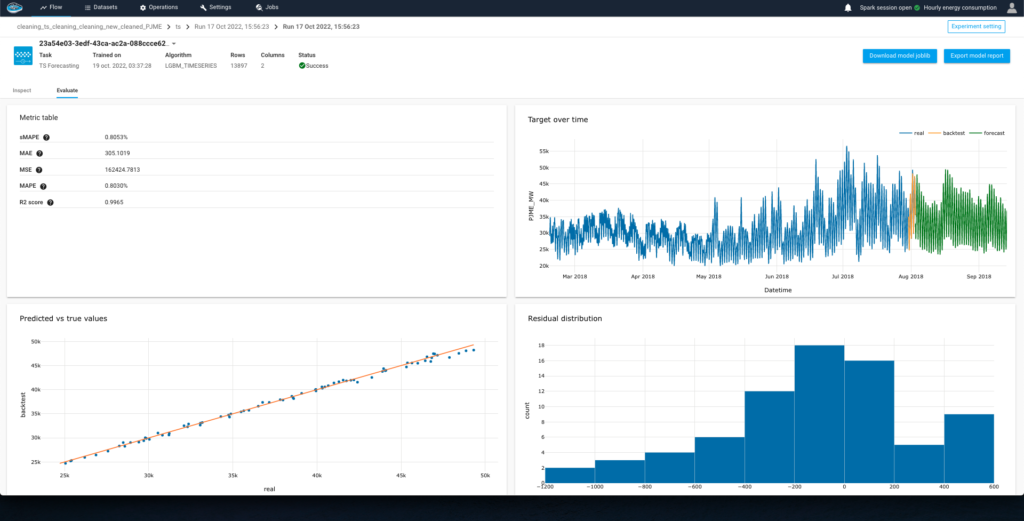
5- Predict values and deploy
When you choose the right model, you can promote it to use its prediction capabilities on any dataset with the same schema and check its performances. When the model is the right fit for you, you can deploy it to use for any external use and answer your business use case.
Conclusion
With the increasing demand for energy and the need to adopt sustainable practices, the use of AI in predicting energy consumption has become even more important. AI-based systems can process vast amounts of data, analyze patterns and trends, and provide organizations with real-time insights into energy usage. This information can then be used to develop effective energy management strategies, reduce energy costs, and improve energy efficiency.
In an era of rapid technological advancement, it is essential for organizations to explore and embrace the benefits of AI. By doing so, they can gain a competitive advantage and ensure that they are well-positioned to navigate the challenges of the future. The use of AI in predicting energy consumption is a vital step towards a more sustainable and efficient energy sector, and organizations should consider exploring this technology further.
Interested in discovering papAI ?
Our commercial team is at your disposal for any question.
How AI Transforms Predictive Maintenance in Defense Equipment
How AI Transforms Predictive Maintenance in Defense Equipment In a...
Read MoreHow to Scale AI Without Breaking Your Infrastructure in 2025
How to Scale AI Without Breaking Your Infrastructure in 2025...
Read More