Why AIOps Is Key to Cyber Threat Detection in Defense?...
Read MoreUnlocking the Power of Scalable AI: Why is papAI a Must-have for Your Organization?
Table of Contents
ToggleSince the dawn of time, companies have been continuously looking for ways to improve their productivity and remain competitive. The use of artificial intelligence (AI) in companies in various sectors (such as healthcare, industry, energy, and hospitality) is becoming increasingly important.
Companies are using AI to make sense of the increasing amount of data they generate and to leverage it to gain insights that will help them grow their businesses. However, as AI becomes increasingly complex, companies now face new challenges in managing and implementing it properly. Scalable AI systems such as papAI have been developed to address this need.
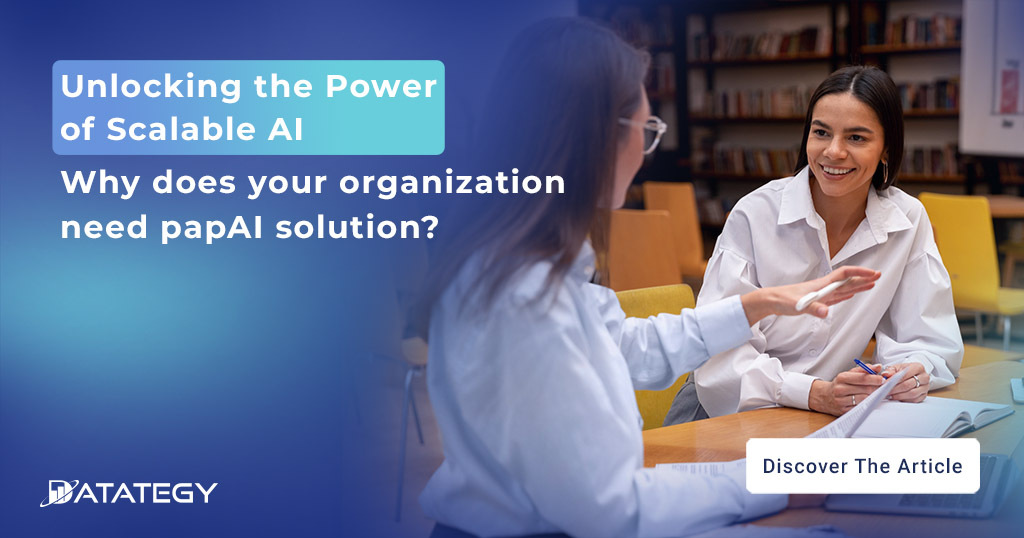
Organizations should prioritize scalable AI for a variety of reasons. It primarily enables businesses to handle and analyze enormous volumes of data rapidly and effectively. This is crucial for organizations that must be able to quickly extract insights from the ever-growing volumes of data they are working with. papAI can rapidly scale up or down processing resources as needed by using cloud computing, providing top performance and cost-effectiveness.
The state of infrastructure today
Learn more in this most recent Fivetran research, which was conducted in July 2022 and polled 500 senior IT and data science experts.
For a range of competencies, organizations have operational systems in place
When reviewing the capacities that businesses have operational systems in place for, the three most likely categories are supply chain management, manufacturing and maintenance, and product lifecycle management. The fact that businesses have these systems in place is good, but it’s important to understand how they are utilizing the data they are collecting through them.
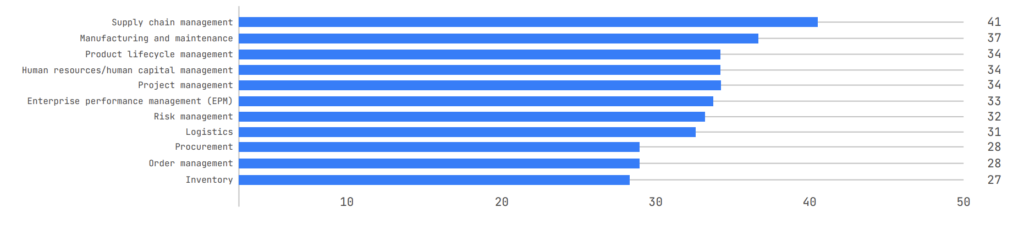
Nearly every organization are gathering and using data from their operating systems
Organizations must gather data, and many of them appear to be doing so well. However, just because a company is using all of its data doesn’t guarantee they are doing it efficiently; in fact, some may be just getting started. If used effectively, data may be a crucial tool for organizational activities like forecasting and decision-making. Therefore, it is heartening to see that data is first being collected and used, but how?
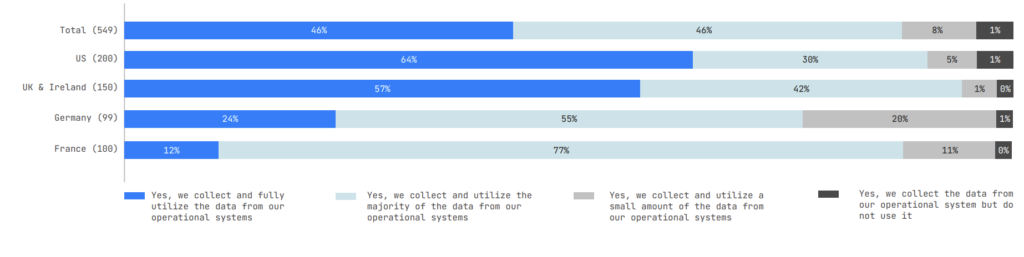
Sector differences exist in the extent to which organizations are using and collecting data from their operational systems
Businesses in the public sector are the least likely to effectively utilize data from their operational systems due to a number of variables. A lack of internal talents, a tight budget, a lack of time, or even old infrastructure might be impediments. For other people, using this data might not be their primary priority. However, when it is obvious, as in the media and industrial sectors, they likely have access to large amounts of data, which, of course, is only valuable if it is presented in a clear and meaningful way.
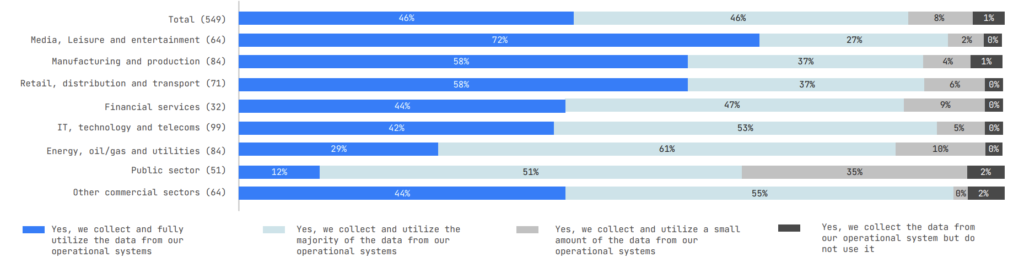
What does the term scaling AI mean?
AI use cases or pilot projects have been successful for several organizations. To scale AI, which requires utilizing technology throughout the organization and leveraging its potential, they are experiencing difficulty. A scalable AI system has to cope with data that is produced in real-time, frequently in the millions of records each day. This contrasts with an AI-based POC or Pilot project, which only interacts with a small piece of data. This necessitates a shift in an organization’s operational paradigm, several top-down and bottom-up actions, the adoption of a new culture, and a significant financial investment.
What advantages does adopting papAI as a scalable AI solution offer?
- Managing growth: One of the main reasons companies need a scalable AI platform is to manage growth. As a company gets bigger, so do the amount of data that has to be processed, the number of users that need to use the platform, and the complexity of the problems that need to be handled. A scalable AI platform could be able to support this growth without needing to completely rethink or reconstruct everything.
- Cost-effectiveness: An AI platform that is scalable can be less expensive than one that isn’t. Building a platform that is scalable from the beginning can be more expensive than constructing one that isn’t, but as the company grows, it may end up being less expensive overall. By minimizing the cost of redesigning or rebuilding the platform as the business grows, it helps the organization save money.
- Performance improvement: Scalable AI platforms have the potential to make AI models perform better. As data amount and problem complexity increase, the performance of the models may suffer. A scalable platform could control this increase in data and complexity without compromising performance
- Better decision: Scalable AI platforms enable organizations to examine more data and provide more accurate projections, allowing them to make better decisions. This might lead to improved client satisfaction, cost savings, and increased production.
How papAI platform helps you to scale your AI solution?
1- Make data preprocessing simpler
papAI platform can facilitate data preparation in your company by providing tools and techniques that make it easier to work with larger datasets and more types of datasets. This can include features such as:
- Data integration: papAI can help you integrate data from multiple sources because it’s an intuitive AI platform that allows you to quickly and easily integrate your data at any point during the prototyping of your predictive model, such as structured data from a SQL database and unstructured data from social media or other sources. This can make it easier to work with data from different sources and get a more complete picture of the data.
- Data cleaning: papAI can also help to clean and preprocess data, removing errors, outliers, and missing values. This can help to improve the quality of the data and make it more suitable for analysis, also for your own personalized use and visualization, you have a great latitude to customize your code design via Python.
- Data transformation: papAI platform help to transform data into the format required for analysis. For example, it can convert text data into numerical values, or group data into categories.
- Data Visualization: papAI can also provide data visualization tools that make it easier to understand and explore the data through a 2D, 3D view and geographical plots. Compare several models for better visualization and easily access the visualization models of your collaborators. This can help to identify patterns and trends in the data, which can inform decision-making.
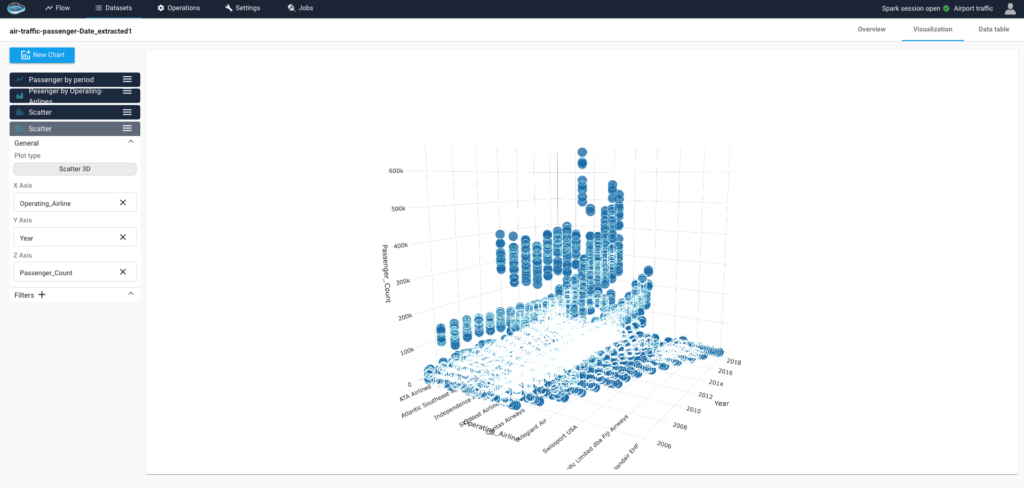
-Example of Data visualization on papAI platform Scatter 3d-
2- Help experimentation with model training and scaling
Scaling experiments and model training may be made easier with the help of papAI platform because it offers a centralized and automated method of doing so. The platform can automate the tedious and time-consuming processes of model selection, hyperparameter tweaking, and data preparation by using an AutoML engine.
Data scientists and engineers may now concentrate on more challenging and worthwhile activities like feature engineering and model interpretability. A scalable AI as papAI platform further enables users to create unique models using coding languages like Python or Scala. As a result, data scientists and engineers may have more control over the models that are trained and apply their domain-specific knowledge to develop models that are suited to the organization’s particular requirements.
papAI solution makes it simple to deploy models in real-world settings. Once a model has been trained and certified, it may be easily accessed and used by other teams for their own applications by being deployed to the platform’s model management system.
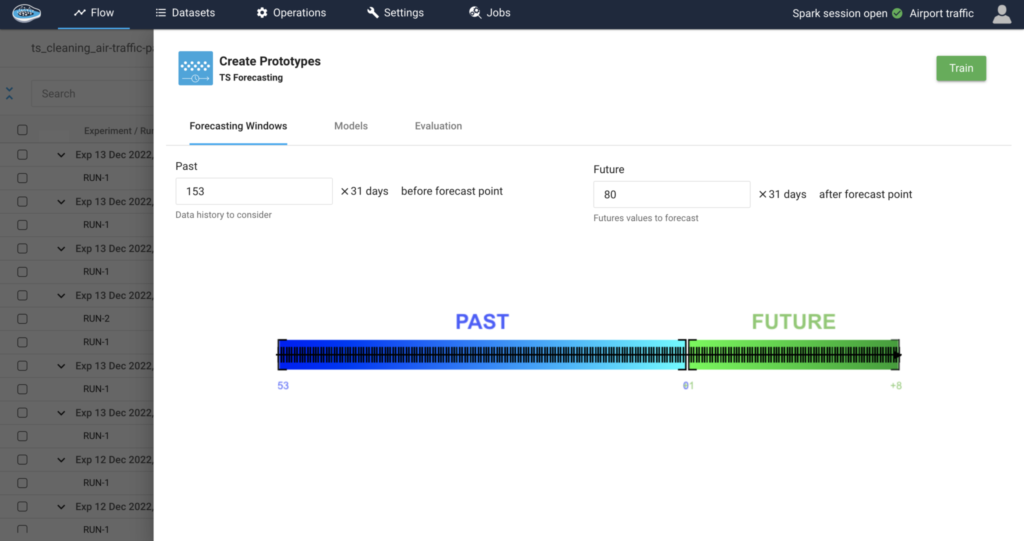
– Example of training data on papAI platform –
3- Monitoring During Deployment and Scaling for Production
papAI platform can facilitate scaling for production and monitoring during AI deployment by utilizing a multi-node architecture and by increasing the speed of scoring models.
- Multi-node architecture: A multi-node design enables the papAI to split the workload over several computers, enabling it to process many requests at once. This is especially crucial in circumstances when there are lots of queries and real-time responses are needed, such as production settings.
- Speed of scoring models: Using technologies like GPU acceleration, which enables quicker processing of complicated models, the speed of scoring models may be greatly boosted. This is especially significant in production settings where quick decision-making depends on reduced latency.
- Monitoring: A scalable AI platform such as papAI makes it simple to keep tabs on the effectiveness of the used models. This involves tracking the models’ predictions and drifts as well as keeping an eye on how much CPU and memory are being used. This makes it possible for teams to recognize and handle any difficulties that can emerge throughout the deployment process immediately.
- Deployment: The deployment of models into production is also made simple by a scalable AI platform. Once a model has been trained and certified, it may be easily accessed and used by other teams for their own applications by being deployed to the platform’s model management system.
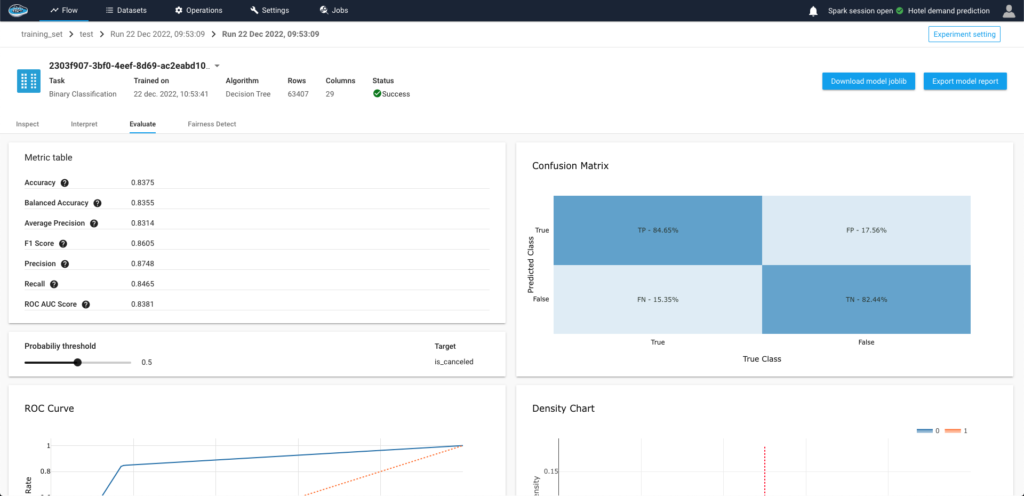
– Example of evaluation of the model on papAI platform –
Conclusion
In conclusion, a scalable AI platform can facilitate scaling experimentation and model training by providing an automated approach to the process, enabling users to create custom models, allowing for parallel experimentation and model training, and making it easy for models to be deployed into production.
Book your demo now. Our team of experts can help you create a custom AI-based tool that meets the unique needs of your organization.
Interested in discovering papAI?
Our commercial team is at your disposal for any questions
How AI Transforms Predictive Maintenance in Defense Equipment
How AI Transforms Predictive Maintenance in Defense Equipment In a...
Read MoreHow to Scale AI Without Breaking Your Infrastructure in 2025
How to Scale AI Without Breaking Your Infrastructure in 2025...
Read More“DATATEGY EARLY CAREERS PROGRAM” With Noé Vartanian
“DATATEGY EARLY CAREERS PROGRAM” With Noé Vartanian Hello all, my...
Read More