Why AIOps Is Key to Cyber Threat Detection in Defense?...
Read MoreMachine Learning (ML) in Insurance: Uncovering Patterns and Insights for Improved Decision-making
Table of Contents
ToggleSince risk management is the foundation of the insurance sector, successful and profitable decision-making is essential. With the development of machine learning (ML), insurers now have a potent tool at their disposal to sift through massive volumes of data, find patterns, recognize trends, and draw up insightful conclusions.
Find out how papAI can improve the deployment of AI projects in the Insurance Sector here.
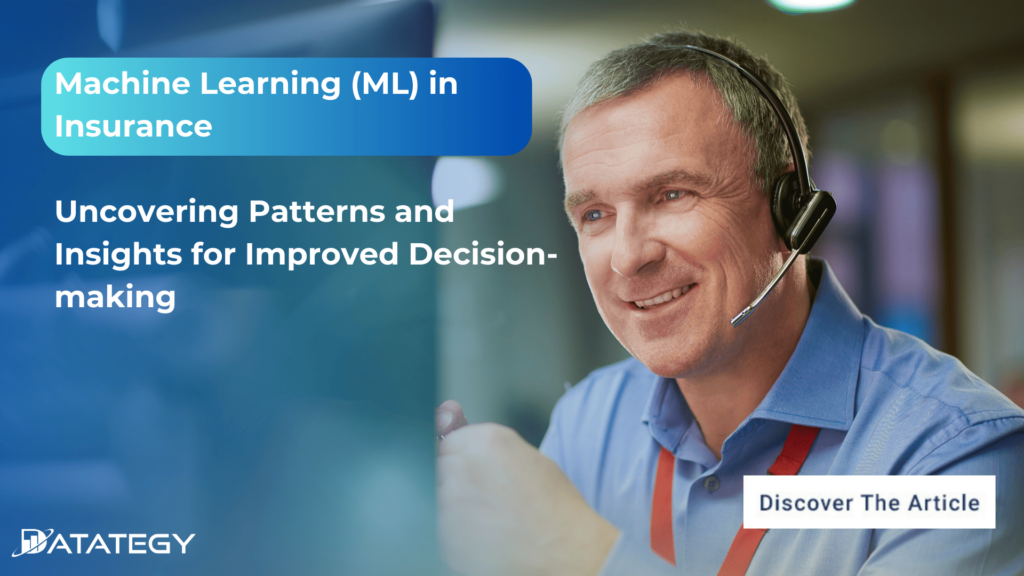
In this article, we will explore the application of machine learning in insurance and how it revolutionizes decision-making processes for business teams.
Overview of AI Adoption in the Insurance Sector
Due to the fact that it remains the largest insurance market in terms of premiums and was the first to implement new technologies and analytics, North America represents the largest portion of the sector.
The North American insurance analytics market is expected to be dominated by the US and Canada in terms of revenue generation. The majority of insurance analytics solution providers, including large enterprises, have a direct or indirect presence in this region through fulfillment agents, distributors, and resellers.
Due to its increasing technology adoption rate, the APAC region is expected to witness the highest growth in the global insurance analytics market between 2020 and 2023.
The insurance analytics market in the APAC region is expected to be driven by rapid economic development, globalization, digitalization, and the increasing use of cloud-based technologies.
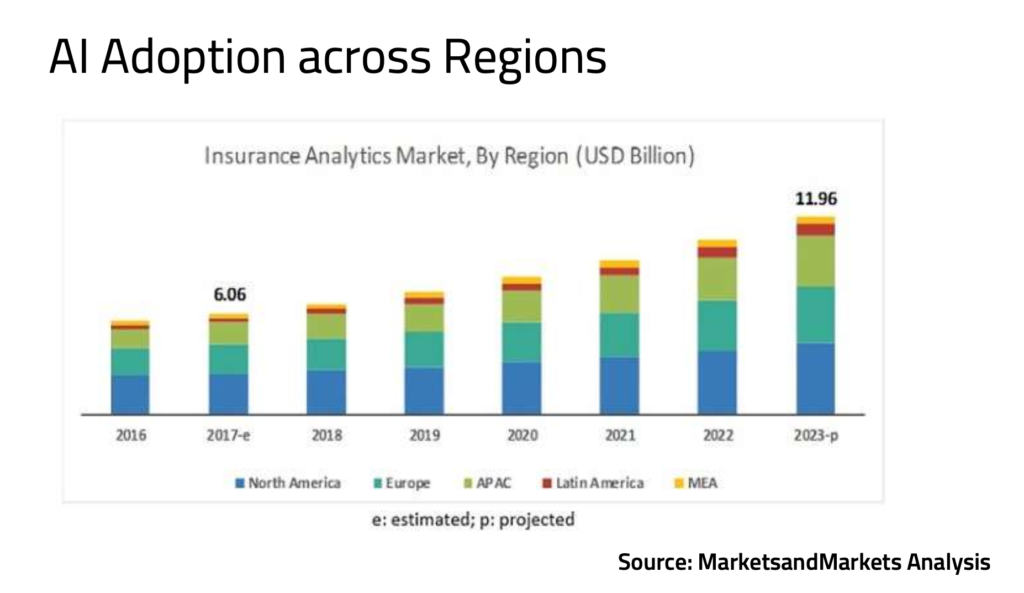
Understanding Machine Learning in Insurance
Due to technological improvements, the insurance industry has seen a substantial transition in recent years. The development of machine learning, a branch of artificial intelligence, has revolutionized the insurance industry by allowing insurers to make wise decisions by properly utilizing their data. Machine learning algorithms can anticipate future results, find anomalies, and automate numerous insurance procedures by learning from prior data through training.
Machine learning algorithms are created to learn from data and generate predictions or take action. These algorithms analyze historical data in the insurance industry to find patterns, correlations, and trends that human analysts might overlook. They can get information that helps with pricing plans, claims management, fraud detection, and improving the client experience.
What are the advantages of machine learning in insurance?
1- Improving Fraud Detection and Prevention
Machine learning is proven to be a great ally in this issue as fraud identification and prevention are significant difficulties the insurance business faces. It costs insurers billions of dollars annually since traditional techniques of fraud detection frequently fail to catch sophisticated fraudulent actions. According to a study by McKinsey, insurers leveraging AI for fraud detection reported a significant increase in detection accuracy, with some achieving detection rates as high as 90%.
Large-scale data analysis, revealing hidden patterns, and spotting abnormalities that can point to fraud are all skills at that machine learning algorithms excel at. These algorithms can identify suspect patterns and behaviors by continually learning from prior claim data and flagging claims for additional inquiry. By identifying fraudulent claims as early as possible, this proactive method enables insurers to reduce losses. Machine learning algorithms are also particularly successful at remaining one step ahead of fraudsters since they can adjust and develop new fraud strategies.
2- Enhancing Risk Assessment
A crucial component of the insurance sector is improving risk assessment, and machine learning is playing a key role in this area. With the use of cutting-edge algorithms and data analytics, machine learning gives insurers the ability to comprehend risks more thoroughly and provide more precise estimates. Traditional methods of risk assessment frequently rely on scant historical data and subjective interpretation, which can produce discrepancies and errors. On the other hand, machine learning algorithms can evaluate enormous volumes of data from several sources, revealing hidden patterns and recognizing intricate risk indicators. Insurance companies can foresee future trends, make better judgments, and streamline their underwriting procedures thanks to this thorough study.
Machine learning also automates risk assessment, reducing processes and boosting effectiveness. Insurance companies may increase the accuracy of their pricing, customize coverage choices, and make better risk management decisions by strengthening risk assessment with machine learning. By enabling insurers to make more accurate and data-driven judgments, machine learning’s revolutionary potential is altering the insurance industry. Learn how machine learning may transform the way risk is assessed in the insurance sector.
3- Optimize Pricing
In the insurance sector, pricing optimization is a crucial component, and machine learning is showing to be a game-changer in this area. Pricing decisions in the past have frequently been made based on historical data and broad market patterns, which has resulted in inconsistent and incorrect pricing. But now that machine learning has become a reality, insurers can use cutting-edge algorithms to analyze massive quantities of data and determine prices with more accuracy.
According to a study by McKinsey & Company, a 1% improvement in pricing can lead to an average increase of 11.1% in operating profit. With the help of machine learning algorithms, insurers may better understand risk variables and how they affect pricing by seeing patterns, correlations, and hidden insights in data. Machine learning algorithms can produce more precise and individualized pricing modes by using a variety of data sources, including as policyholder information, historical claims data, market trends, and external variables.
Machine Learning in Insurance: Challenges and Opportunities
Data Availability and Quality
For machine learning algorithms to deliver reliable results, high-quality data is necessary. Insurance companies are required to make sure that their data is accurate, organized, and properly labeled. Additionally, it might be difficult to acquire pertinent external data sources and incorporate them into the models.
Ethics and Privacy Issues:
Privacy and ethical issues are crucial since machine learning uses a lot of personal data. To secure sensitive information, insurers are required to implement strong security protocols and adhere to data protection laws. Building client trust through algorithmic decision-making requires transparency as well.
Management of Change and Skills Gap:
Adopting machine learning needs qualified people who are conversant in data science and insurance. Significant obstacles include the need for cultural transformation inside organizations and the skill gap in the sector. In order to fully utilize machine learning capabilities, insurers must make training investments and foster a data-driven culture.
Demystifying AI: A Comprehensive Guide to Key Concepts and Terminology
This guide will cover the essential terminology that every beginner needs to know. Whether you are a student, a business owner, or simply someone who is interested in AI, this guide will provide you with a solid foundation in AI terminology to help you better understand this exciting field.
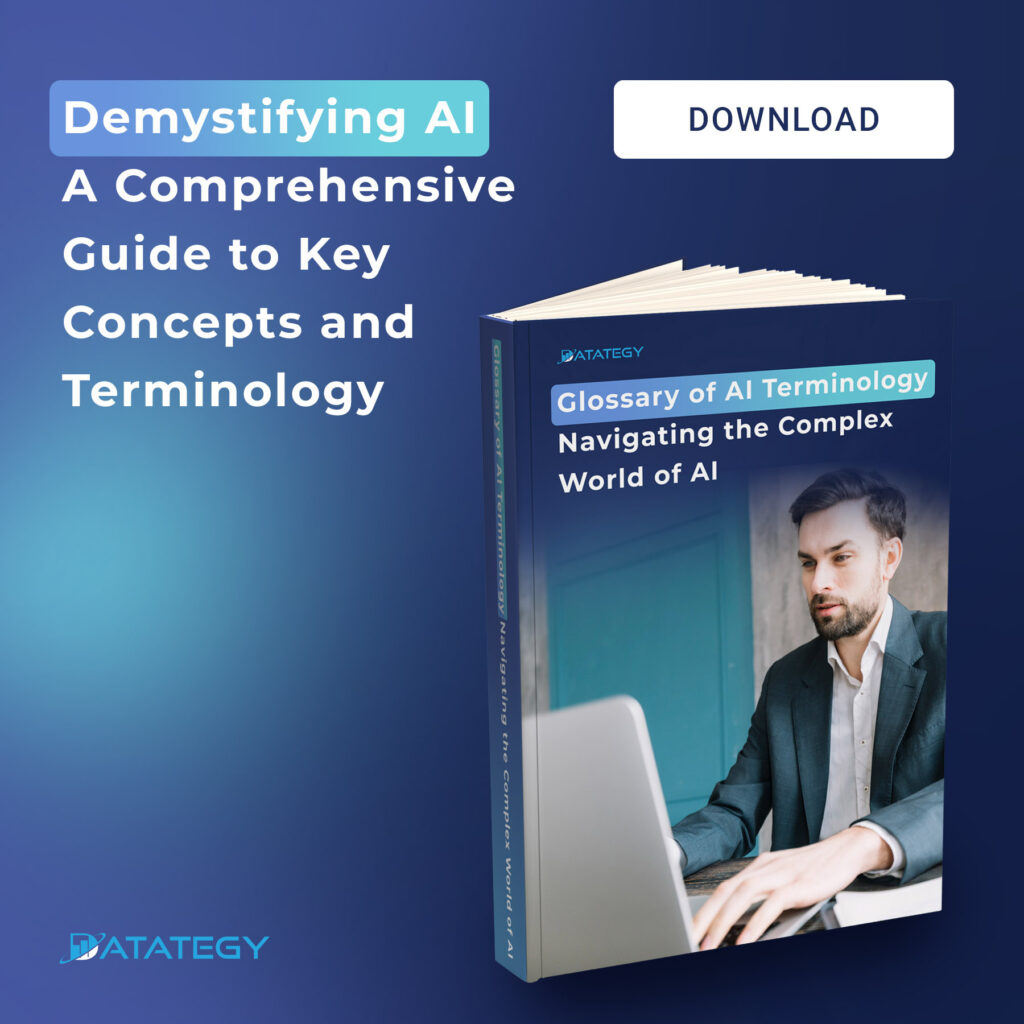
Case Study: How Can papAI Solution Improve Prediction of Customer Churn?
1- Initial Dataset Presentation
The goal of this use case is to create a machine learning model that employs 16 differentiating characteristics (features 0 through 15) to forecast client attrition in the insurance sector. Insurance firms should develop proactive measures to reduce customer attrition and improve long-term client relationships by precisely identifying prospective churners in advance.
The objective of the study is to draw attention to the importance of using data-driven methodologies to forecast customer attrition and its consequences for the expansion and profitability of the insurance sector.
We use one-hot encoding, which handles categorical variables by generating binary columns that reflect the categories, to get the data ready for analysis. Instead of randomly giving numerical values to categories, it produces binary columns that expressly state whether a certain category is present or absent in each row. This aids in avoiding
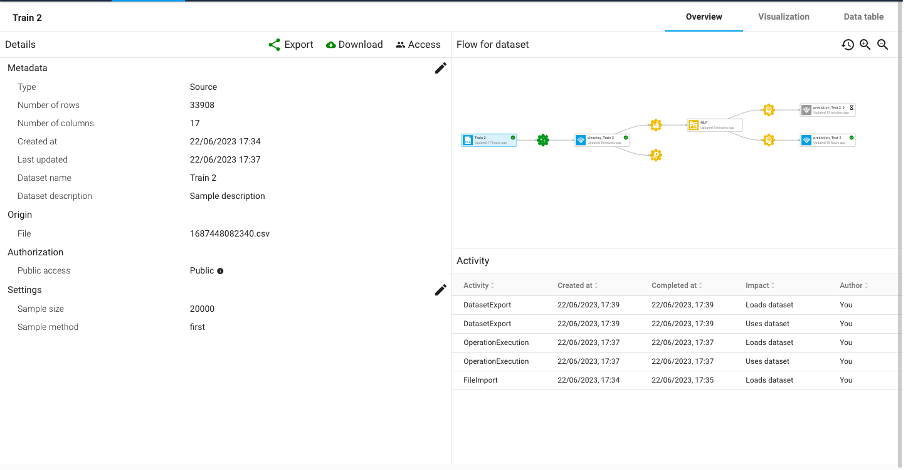
2. Data Preparation and Analysis
We use one-hot encoding, which handles categorical variables by generating binary columns that reflect the categories, to get the data ready for analysis. Instead of randomly giving numerical values to categories, it produces binary columns that expressly state whether a certain category is present or absent in each row. This helps keep the model from being misled by unexpected ordinality assumptions.
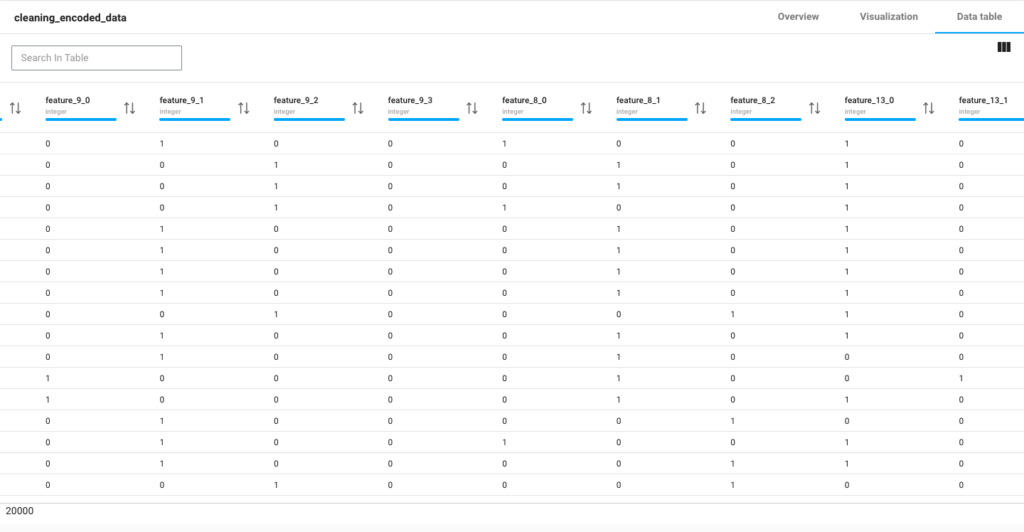
3- Model Training
Building and testing our Insurance Churn prediction model throughout the training phase required the use of many models from the papAI Solution. For categorization jobs, papAI offers a broad variety of machine learning algorithms and methods.
Using the training data derived from the stratified split, we first trained a number of models. These models comprised well-known classifiers including support vector machines, decision trees, logistic regression, and random forests. The input characteristics and matching binary target variables were used to train each model. In order to solve the issue of class imbalance, we also applied the data augmentation technique SMOTE (Synthetic Minority Over-sampling Technique), which creates synthetic samples for the minority class in order to equalize the distribution of classes.
The data augmentation techniques available in papAI included random over-sampling, SMOTE (Synthetic Minority Over-sampling Technique), ADASYN (Adaptive Synthetic Sampling), Borderline SMOTE, SWIM maha (Synthetic With Interpolation of Minority using Mahalanobis distance), and SWIM RBF (Synthetic With Interpolation of Minority using Radial Basis Function).
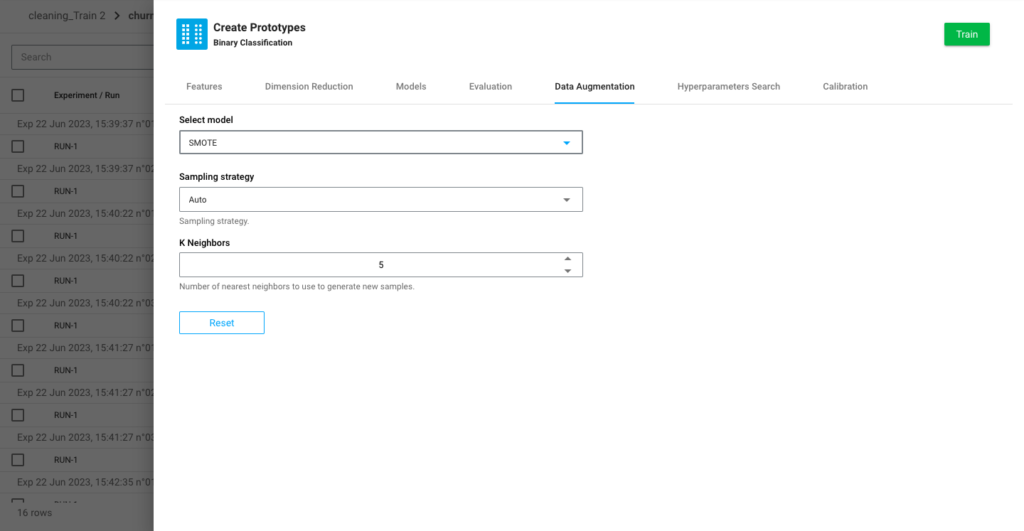
We chose the model with the best performance based on the evaluation’s findings. By choosing the model with the best performance, we can be certain that our solution will be deployed with the maximum level of accuracy in predicting whether or not the insurance business would lose a client.
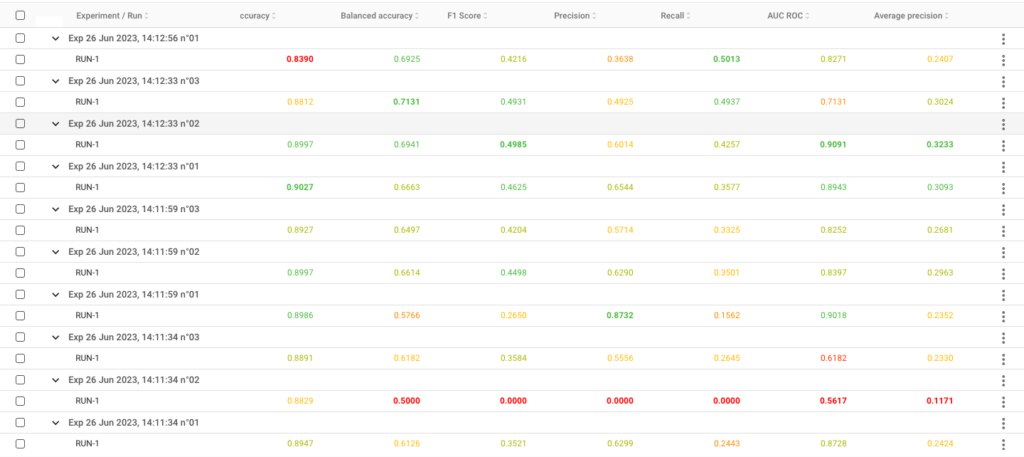
4- Explain the Predicted Outcome
After deploying the model chosen and making the prediction on the test set we can use the counterfactuals artifact proposed by papAI solution which allows us to choose a row and manipulate the different features to see how the model will react depending on each variable as you can see in the figure below, we changed the value of some feature and turned the prediction from 54,79% false to 89.97 true.
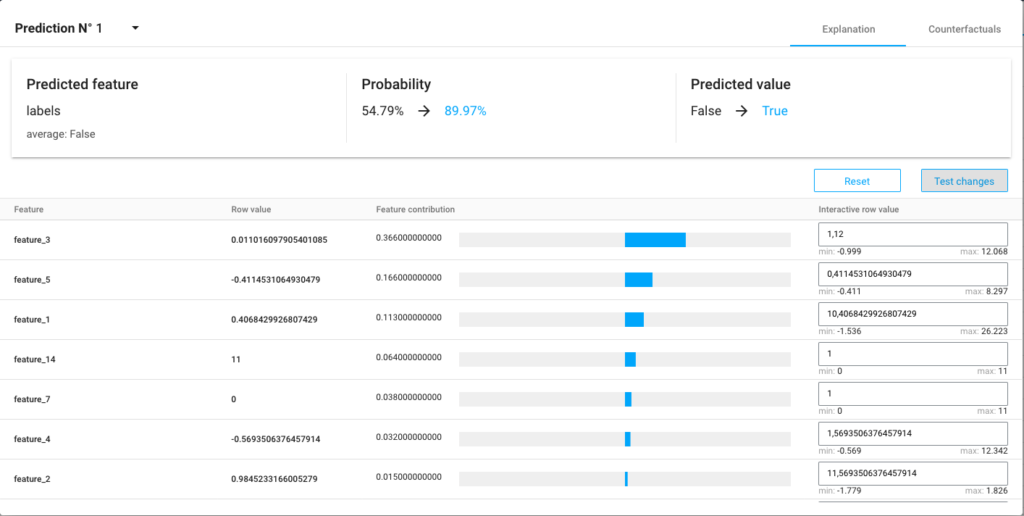
We can identify the elements that have the most effects on the model’s performance by carefully studying the feature impact. The importance of the variables in the learning process is highlighted by the fact that those with greater percentages have a considerable impact on the model’s prediction. Adjustments and future optimizations should be primarily focused on these important factors.
In contrast, factors with smaller percentages might be thought of as having a somewhat lesser effect on the performance of the model. This may be a result of their intricate interactions with other variables in the dataset or the fact that they are less useful.
Create your own AI-based tool with papAI solution to deploy, monitor and maintain your AI projects in Insurance Industry
You may utilize AI to make your company’s AI initiatives more comprehensible with the help of papAI solution. Using papAI, you have the option to create your own AI-based tool that is tailored to your specific needs. Modern machine learning methods will significantly improve the accuracy and efficiency of your prediction processes.
Through a process of data mining, cleansing, and visualization that can hasten the deployment of AI projects, papAI solution aids in explaining and making transparent the outcomes. Reserve your demo today. Our team of specialists can assist you in developing a special AI-based product that is tailored to the requirements of your company.
Interested in discovering papAI?
Our commercial team is at your disposal for any questions
How AI Transforms Predictive Maintenance in Defense Equipment
How AI Transforms Predictive Maintenance in Defense Equipment In a...
Read MoreHow to Scale AI Without Breaking Your Infrastructure in 2025
How to Scale AI Without Breaking Your Infrastructure in 2025...
Read More“DATATEGY EARLY CAREERS PROGRAM” With Noé Vartanian
“DATATEGY EARLY CAREERS PROGRAM” With Noé Vartanian Hello all, my...
Read More