How to Scale AI Without Breaking Your Infrastructure in 2025...
Read MoreHow RAG Transforms Sales and Marketing Performance
Table of Contents
ToggleIn sales and marketing, it’s easy to get carried away by buzzwords. Every few months, a new framework or model hits the headlines, only to fade away as the next big thing emerges. But Retrieval-Augmented Generation is not just another passing trend. It represents a fundamental shift in the way we think about combining structured knowledge with the power of generative models and artificial intelligence.
The global Retrieval Augmented Generation market size was estimated at USD 1.2 billion in 2024 and is projected to grow at a CAGR of 49.1% from 2025 to 2030, indicating strong market belief in its benefits and potential (Grand View Research).
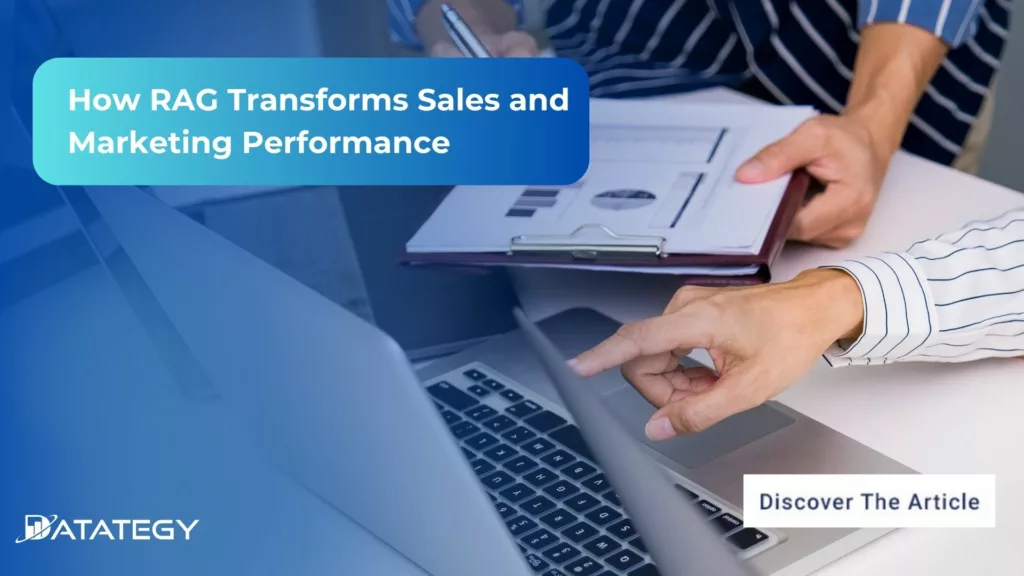
Discover how Retrieval-Augmented Generation (RAG) is revolutionizing sales and marketing teams and their workflows. We’ll pinpoint the specific ways it simplifies crucial processes.
What does RAG mean?
Retrieval-Augmented Generation (RAG) is an advanced architectural paradigm intended to improve large language models’ (LLMs’) performance in knowledge-intensive activities. Unlike purely generative models that rely only on their pre-training parametric knowledge, RAG incorporates an explicit information retrieval method into the text generation process. The process entails the first extraction of relevant material from an external knowledge base, which could include a variety of sources like document corpora, online data, or structured knowledge graphs.
What Are a RAG System's Fundamental Elements?
Knowledge Base
Any RAG system is built on top of the Knowledge Base. All of the information, records, and reference materials are kept there. Without it, the generator would have nothing solid upon which to base its responses, and the retriever would have nothing to search. Consider the knowledge base to be the library of your AI. Anything from product manuals to internal corporate procedures to legal documents, client correspondence, or research papers could be included. The most important thing is that it contains the data that your users are interested in and is arranged such that it is simple to use and access.
It takes more than just throwing a number of files into a folder to create a strong knowledge base. For effective searching, the data must be indexed, cleansed, and organised. This frequently entails dividing texts into more manageable portions, adding metadata to them, and, if dense retrieval is being used, turning them into vector embeddings. When a user asks a query, a well-prepared knowledge base guarantees that the retriever can swiftly and precisely focus on the most pertinent information.
Retrievers Systems
In the RAG framework, the Retriever is the first and likely most important phase. When a user asks a question, it is in charge of extracting the pertinent details from a vast amount of data. The retriever goes out and gathers actual, contextually relevant stuff to back the response rather than depending on the model’s internal knowledge, which may be out-of-date or lacking. Imagine it as an intelligent research assistant whose job it is to search the entire knowledge base and retrieve only the most important information.
The retriever’s ability to comprehend meaning is what gives it its effectiveness. When the language changes, traditional search methods that rely on specific keywords may not be accurate. On the other hand, a competent retriever compares the user’s query with the database’s content using embeddings, which are numerical representations of meaning. This enables it to retrieve data not only from surface-level matches but also from semantic similarities. As a result, the retriever can still make connections even if the enquiry and the document have different language.
Generators
The Generator is the component of the RAG framework that does the communicating; it transforms the information that has been retrieved into a response that makes sense and sounds natural. The main drawback with conventional language models, such as GPT, is that they frequently make stuff up, even when they are already adept at producing fluent text. The generator in a RAG system constructs its response using the real documents and information the retriever gives, rather than merely drawing concepts from its training data. As a result, the output is intelligent in addition to sounding intelligent.
In this configuration, the generator’s strength lies in its capacity to comprehend and integrate fragments of recovered content into a coherent story. Instead of just copying and pasting from the source, it synthesizes. The generator can, for instance, integrate three pages about a topic that the retriever pulls with slightly varied wording to produce a single, legible response that seems coherent and conversational. When responding to complicated or multi-part queries, where knowing the tone and context is just as important as providing accurate information, this is very helpful.
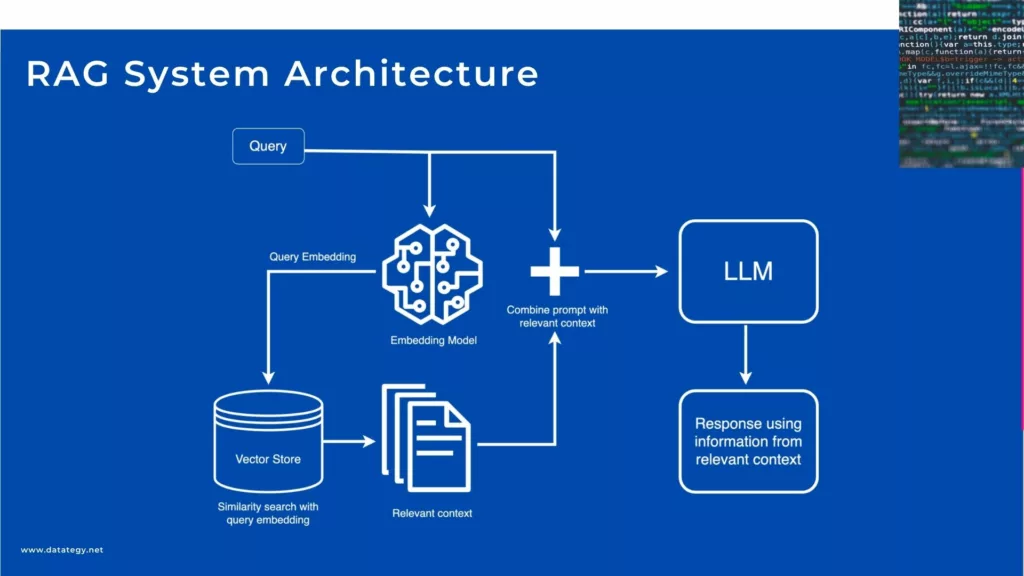
RAG System Architecture
What are the Benefits of Retrieval-Augmented Generation in Sales and Marketing?
Knowledge Standardization
Conflicting knowledge is a major obstacle to sales and marketing teams aligning. Sales teams are frequently forced to rely on antiquated slides or ad hoc notes, whereas marketing may use the most recent product updates, messaging frameworks, or market positioning. By centralising information access into a single, intelligent system that both teams can query in real-time, RAG bridges this gap. They may ask RAG — and receive reliable, accurate responses — rather than searching through folders or asking around.
The sales and marketing teams remain in sync when RAG serves as the one source of knowledge. The same current sources are used for campaign data, objection management, competitive positioning, and product messaging.There are strong knock-on effects from this alignment. The ability of sales to follow up on marketing’s narrative increases the effectiveness of campaigns. Lead handoff gets better. Instead of hearing from two departments, customers only hear one voice.
Enhanced Content Accuracy
In the rapidly evolving digital world of today, incorrect or out-of-date content can drastically harm a brand’s trust. By allowing RAG systems to consult real-time data sources when producing responses or material, retrieval-augmented generation, or RAG, transforms the game. Teams may now access real-time data that represents the market, news, and consumer behaviour instead of being restricted to static training datasets.
For sales and marketing professionals who depend on current information to make product pitches, respond to objections, and establish credibility, this feature is extremely beneficial. RAG guarantees that the AI-driven material used in customer communications, email campaigns, or landing sites is correct and pertinent, regardless of the subject matter—product specifications, competition comparisons, or regulatory changes. No more embarrassing fixes or lost chances because of outdated data.
Personalization at Scale
Without using a lot of manual labour, businesses can now provide highly customised content experiences across a variety of touchpoints through RAG. In order to create responses or materials that fit each customer’s profile and behaviour, the system dynamically pulls the most pertinent data from both internal and external sources.
Consider a sales email that, without requiring a human to draft each version, adapts its tone and content according to the recipient’s industry, past interactions, and current interests. is a landing page that changes its content based on the visitor’s stage of the purchasing process. These kinds of customised interactions are made scalable, consistent, and surprisingly manageable with RAG.
Efficiency and Scalability
Despite frequently having the same or fewer resources, sales and marketing teams are constantly under pressure to generate more campaigns, content, and outcomes. By automating the content creation process while preserving quality and relevancy, RAG offers a significant efficiency benefit. Teams can produce excellent drafts that are inspired by up-to-date data and prepared for rapid review, saving hours spent creating marketing emails, social media posts, or product descriptions.
The advantages of scalability go even beyond. Launching campaigns across several languages, locations, or sectors can be a logistical nightmare when using traditional approaches. By automatically customising outputs for each unique target, RAG streamlines this process and makes it simpler to localise campaigns and modify messaging to suit various markets.
What are the Real-World Applications of RAG in Marketing?
1. Automated Campaign Brief Generation
Campaign briefs are the foundation of any project for marketing teams, but writing them is frequently a tedious, time-consuming procedure. Teams invest hours in obtaining information, coordinating with product teams, consulting previous campaigns, and determining how to condense everything into a well-organized document. This approach becomes much more collaborative and efficient with RAG (Retrieval-Augmented Generation) without compromising quality.
CRMs, content repositories, brand guidelines, and performance dashboards are just a few examples of the internal systems from which RAG smartly pulls real-time data. It can simultaneously retrieve current external sources such as competitor positioning, market data, or pertinent news. The outcome? A system that can produce a comprehensive, contextualised campaign brief that include timeframes, potential channels, objectives, target audience profiles, key messaging, and even content outlines.
2. Content Creation and Summarization
RAG excels in transforming complex, raw data into representations that are easy for audiences to understand by fusing generative AI with retrieval skills. It creates condensed, contextualised material that truly makes sense for your audience by drawing on both structured and unstructured data, including transcripts, product manuals, public databases, and blog archives. Additionally, it is flexible enough to produce many outputs for various forms, ranging from an executive summary to a concise Instagram caption.
This significantly boosts throughput for marketers without sacrificing quality. You don’t always have to start from the beginning. RAG assists you in adapting the material while maintaining tone, accuracy, and brand voice, saving you the trouble of manually rewriting the same message five times for various devices. It enhances creativity rather than diminishes it.
3. Market Research and Trend Analysis
Understanding your market, competitors, and audience is the first step towards effective marketing. However, let’s face it: performing market research is frequently time-consuming, disjointed, and challenging to stay up to date. Numerous hours are spent by marketers reading papers, extracting information from articles, or following the most recent developments in the sector. RAG turns this into a dynamic, on-demand experience.
RAG may create a consolidated, real-time picture of what’s going on in your market by combining data from several live sources, including competition websites, social media, analyst reports, and customer review platforms. It contextualises the data rather than merely displaying it in its raw form. This gives marketers the confidence and speed to act. Teams can obtain real-time insights in a matter of minutes rather than waiting for a formal quarterly study presentation.
4. Customer Support Enhancement
Support systems with RAG capabilities can draw information from product documentation, frequently asked questions, CRM data, and recent interactions to obtain and produce accurate, context-aware responses. This implies that a chatbot or virtual assistant can not only answer questions more quickly but also more intelligently, customising responses according to the context of the user and even changing tone depending on how they feel. This is a treasure trove for marketing teams. Why? because it scales up the protection of consumer trust and brand voice.
RAG can be applied proactively in addition to reactively. Consider being able to obtain a steady flow of anonymised consumer issues and trends, condensed into a format that your marketing team can use. An explainer campaign is a good idea if 20% of users are having trouble understanding a feature. A change in messaging is indicated if there is a misunderstanding over pricing. By bridging the gap between strategy and support, RAG enables marketing teams to create content that, in their own words, truly solves consumer pain areas.
What are the Real-World Applications of RAG in Sales?
1. Sales Enablement
Giving sales representatives the resources, knowledge, and information they require to close deals more successfully is the main goal of sales enablement. But in reality, a lot of teams suffer from out-of-date playbooks, disorganised data, and erratic messaging. When it comes to providing dynamic, real-time support based on both internal and external data, Retrieval-Augmented Generation (RAG) is revolutionary. It turns into a constantly current and available digital co-pilot.
A new salesperson getting ready to make a pitch. They just query the RAG system, “What’s our best use case for a logistics company in Europe?” rather of sifting through folders for hours or hounding colleagues for the most recent case studies. From carefully curated corporate knowledge, it instantly pulls pertinent case studies, product information, and even competition comparisons, and then produces a concise, pitch-ready response. Not only does that save time, but it also increases consistency and confidence.
2. Contract Document Analysis
Any experienced salesperson is aware that the contract phase is frequently when a deal can get stuck. Back-and-forth talks, lengthy, complex legalese, and the desire for speed can all lead to friction and delays. By swiftly and effectively evaluating contract documents, RAG can relieve this bottleneck and enable sales teams to work more quickly without sacrificing accuracy.
RAG is able to scan and evaluate contracts, proposals, and legal documents, extracting the most pertinent provisions and providing the sales representative with a summary of the main elements. RAG offers an easy-to-read summary of the document, whether it be in terms of service, pricing models, or renewal conditions, assisting sales teams in promptly identifying any possible problems or possibilities.
3. Analysis and Reporting
From conversion rates and sales cycles to client acquisition expenses and pipeline velocity, sales teams must monitor a wide range of data in order to assess their success and pinpoint opportunities for development. Reporting has historically been laborious and error-prone. By combining data from many sources and producing customisable, real-time sales reports, RAG streamlines this procedure.
Sales teams can request particular information by integrating RAG with CRM systems, analytics platforms, and performance dashboards. For example, “What is the win rate for deals in the energy sector over the last quarter?” or “Create a report on this month’s top-performing representatives.” RAG saves time and enhances decision-making by processing this data and generating an understandable report.
4. Prospect / Client Communications
Making sure that each client or prospect receives individualised communication is one of the biggest problems facing sales teams. Maintaining individualised outreach can become a logistical challenge when dealing with high lead volumes. RAG, on the other hand, makes this process scalable by creating customised communications for each step of the sales funnel, from the initial touchpoint to continuous nurture sequences, utilising real-time data.
To provide highly customised outreach, RAG gathers information from the CRM system, client records, previous exchanges, and even social media profiles. “Generate an email for a retail industry prospect who downloaded our eBook on eCommerce strategies,” is a question a sales representative may pose to RAG. The AI system will use the customer’s interests and behaviour to create a personalised, pertinent email.
How to Build a RAG System Step-by-Step?
Step 1: Curating and Preparing Your Knowledge Base
Before anything else, you need to decide what information your AI system will rely on. This involves identifying the right documents, databases, and knowledge sources that contain the facts and insights your users will need. These could be internal reports, support articles, regulatory guidelines, or any trusted content relevant to your domain. The goal here is to build a solid, reliable foundation that reflects your organization’s knowledge accurately.
Once the content is selected, it needs to be cleaned and organized. This includes removing duplicates, outdated files, or irrelevant material. Documents are often split into smaller passages or “chunks” to make retrieval more precise. For example, instead of indexing an entire PDF, you break it into sections like paragraphs or bullet points. That way, the retriever can surface only the pieces that match a query no more and no less.
Step 2: Setting Up the Retrieval Layer
The retrieval layer is the bridge between the user’s question and the information buried in your knowledge base. When a user asks something, the retriever’s job is to go fetch the most relevant pieces of content. But for it to work properly, you need to carefully configure how your search works do you use a dense retriever, a sparse one, or a hybrid that combines both? Each approach comes with trade-offs in terms of speed, accuracy, and complexity.
Dense retrieval uses embeddings to match the meaning of a question to the meaning of a document. It’s great for understanding context and nuance, which makes it powerful in conversational AI.On the other hand, sparse retrieval (like traditional keyword-based search) is simpler and faster, but can miss the mark when questions are phrased in unexpected ways. Combining both through a hybrid method often gives the best of both worlds.
Step 3: Fine-Tuning or Prompt Engineering the Generator
With relevant information now flowing in, it’s time to focus on the generator the voice of your RAG system. You have two main paths here: fine-tuning or prompt engineering. Fine-tuning involves training your language model further using examples that are specific to your domain. This helps the model adapt to your tone, terminology, and answer style. It’s great for consistency, especially in high-precision environments like legal or medical settings.
Prompt engineering, by contrast, is faster and doesn’t require retraining the model. Instead, you carefully craft instructions and examples within the prompt to guide how the model responds. This might include formatting rules, the level of detail to include, or what kind of tone to use (formal, casual, instructional, etc.). Prompt engineering gives you flexibility, especially when working with powerful pre-trained models you can’t modify directly.
Step 4: Connecting the Pipeline
Once each component is in place, the next step is to connect everything into a functioning pipeline. This is where the magic happens: the user inputs a question, the retriever pulls relevant data from the knowledge base, and the generator crafts a grounded, readable response. Each part needs to communicate smoothly with the next, so your infrastructure setup matters, especially if you’re deploying at scale or integrating into an existing app or chatbot.
Depending on your stack, you might need APIs, orchestration tools, or middleware to glue everything together. Some teams use frameworks like LangChain or LlamaIndex to simplify these connections, while others build custom flows for tighter control. The important thing is to ensure the hand-off from retriever to generator includes all necessary metadata like source attribution, confidence scores, or user-specific preferences.
Step 5: Testing and Validating Output Quality
Even with the best setup, a RAG system is only as good as the answers it delivers. That’s why testing and validation are critical. At this stage, you need to evaluate how well the system performs across different types of questions and scenarios. Are the answers accurate? Are they consistent? Do they cite the right sources? A mix of manual review and automated testing can help catch issues early and refine your pipeline.
Start by defining what “good” looks like for your specific use case. In a customer service chatbot, for example, clarity and tone might matter more than academic precision. In a legal assistant, every word may need to be backed by a verifiable source. From there, you can build evaluation datasets with real user questions and benchmark how your system responds. This includes testing for edge cases, ambiguous queries, and even potential bias in the responses.
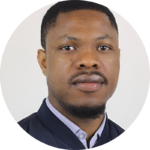
RAG goes beyond fetching information; it transforms static data into actionable intelligence, enabling AI to deliver context-aware, meaningful responses rather than generic outputs.
Kenneth EZUKWOKE
Data Scientist
AI and Machine Learning for Sales: The Key to Unlocking Growth Potential
The development of artificial intelligence (AI) has significantly changed the sales industry, which has witnessed a remarkable evolution throughout time. The way companies approach sales processes and strategies has been completely transformed by AI. In this white paper, we will examine the concept of AI in sales, its historical evolution, and the advantages it provides for companies.
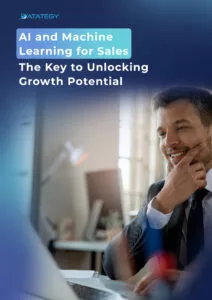
How papAI Helps you to Build RAG Systems ?
papAI is an all-in-one artificial intelligence solution made to optimize and simplify processes in various sectors. It offers strong data analysis, predictive insights, and process automation capabilities.
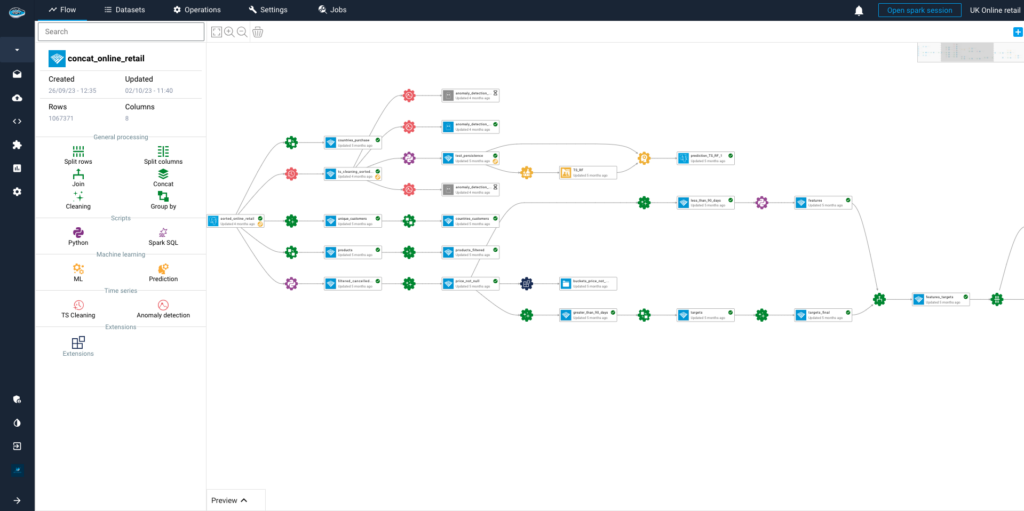
Here’s an in-depth look at the key features and advantages of this innovative solution:
What are the key factors that differentiate Datategy's RAG approach?
Datategy’s approach to building Retrieval-Augmented Generation (RAG) the Hyper-layered Asynchronous Hybrid RAG (HAH-RAG) represents a significant leap in how large-scale legal and enterprise documents are processed, understood, and used to generate high-quality answers. This innovation responds directly to the limitations faced by traditional RAG pipelines, especially when scaling to massive document repositories and maintaining context relevance.
Traditional RAG frameworks often falter when asked to process expansive knowledge bases. They either lose context by overly chunking documents or sacrifice accuracy for speed in their retrieval phase. HAH-RAG avoids this trap by distributing the workload across hyper-layered retrieval tiers. These layers distinguish between high-level topics and granular subcomponents within documents, ensuring that only the most relevant and contextual passages are retrieved.
This intelligent layering helps maintain both global and local document context, allowing the system to be exponentially scaled while preserving precision. For law firms, this means being able to work with massive sets of contracts, case law, or compliance archives without the usual degradation in relevance or response quality.
1- Optimizing Document Retrieval
papAI-RAG combines papAI’s sophisticated capabilities for preprocessing with an improved asynchronous vector database search, enabling a powerful dual-approach system for seamless retrieval. papAI’s modular workflow would handle chunking and indexing while maintaining clear data lineage with its hyper-layered approach that enables multi-granularity search across different data levels.
The key benefits include significantly faster retrieval times (64.5% latency improvement), better data quality through papAI’s preprocessing, and more comprehensive search results through the combined retrieval methods.
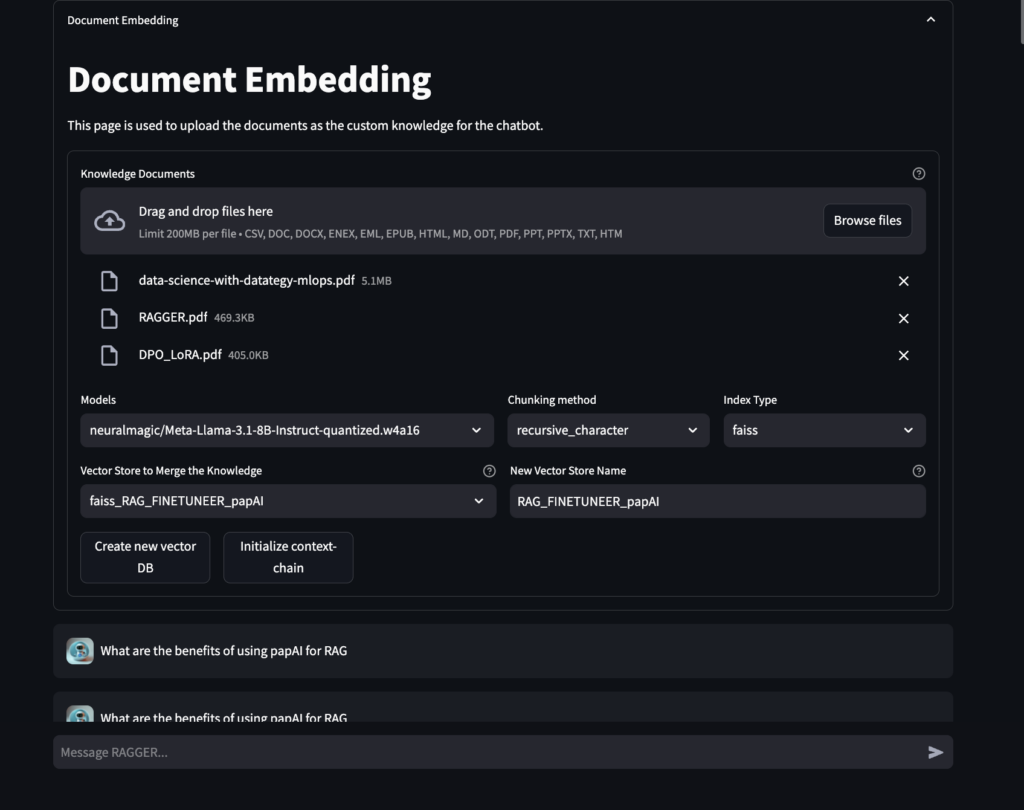
2- Response Generation
the combines papAI-RAG leverages papAI’s data validation and quality control features alongside advanced weighted reciprocal rank fusion and reranking methods for context selection and response generation. Benefits include reduced hallucination rates, improved response relevance, better explainability through visualizations, and a 66.6% reduction in energy consumption compared to traditional RAG approaches.
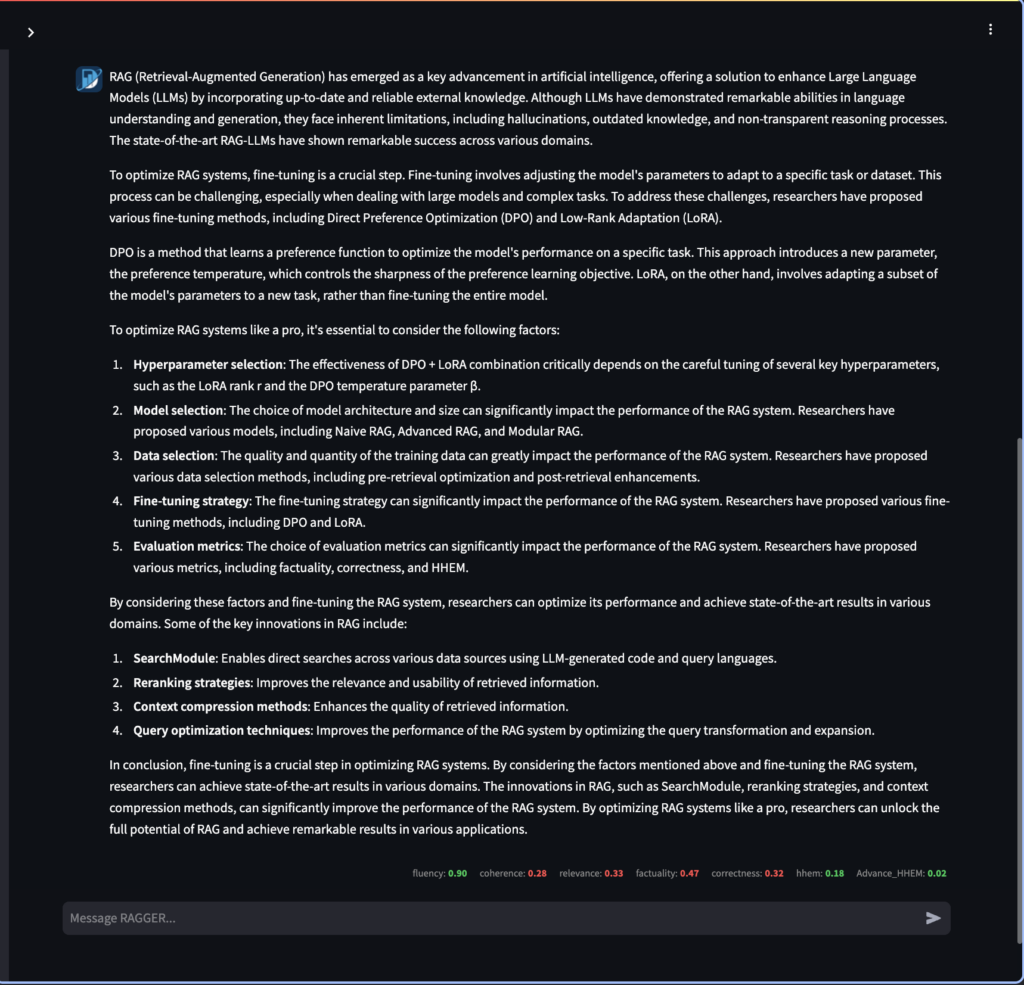
Build Your Own RAG and Unlock the Full Power of Your Sales & Marketing Data with Datategy
In a world where data is everywhere but often underutilized, sales and marketing teams need more than generic AI tools; they need solutions built for their goals, their data, and their pace.
With Datategy’s RAG systems, you can design a Retrieval-Augmented Generation system tailored to your business context. Whether you’re personalizing campaigns at scale, enabling your salesforce with real-time insights, automating content generation, or aligning communication across teams, our platform offers a flexible, high-performance foundation to accelerate your commercial impact.
Discover how a custom RAG system can transform the way your teams sell, market, and grow. Schedule a demo today.
Retrieval-Augmented Generation (RAG) is an advanced architectural paradigm intended to improve large language models’ (LLMs’) performance in knowledge-intensive activities.
- Embedding Models: These transform raw text into dense vector representations, enabling computers to understand the semantic meaning of words and phrases for efficient information retrieval.
- Retrievers Systems: These are responsible for locating and obtaining relevant information from vast amounts of data using methods like sparse retrieval (keyword matching) and dense retrieval (semantic meaning matching).
- Generators: These convert the retrieved relevant information into grammatically correct, contextually appropriate, and human-readable text, often utilizing large language models.
1. Automated Campaign Brief Generation.
2. Content Creation and Summarization.
3. Market Research and Trend Analysis.
4. Customer Support Enhancement.
1. Sales Enablement.
2. Contract Document Analysis.
3. Analysis and Reporting.
4. Prospect / Client Communications.
Interested in discovering papAI?
Our AI expert team is at your disposal for any questions
“DATATEGY EARLY CAREERS PROGRAM” With Noé Vartanian
“DATATEGY EARLY CAREERS PROGRAM” With Noé Vartanian Hello all, my...
Read MoreUse AI to Predict Your Customer’s Future Value
Use AI to Predict Your Customer’s Future Value Understanding and...
Read MoreHow Geospatial Analytics Transforms Decision-Making
How Geospatial Analytics Transforms Decision-Making Making the right judgments in...
Read More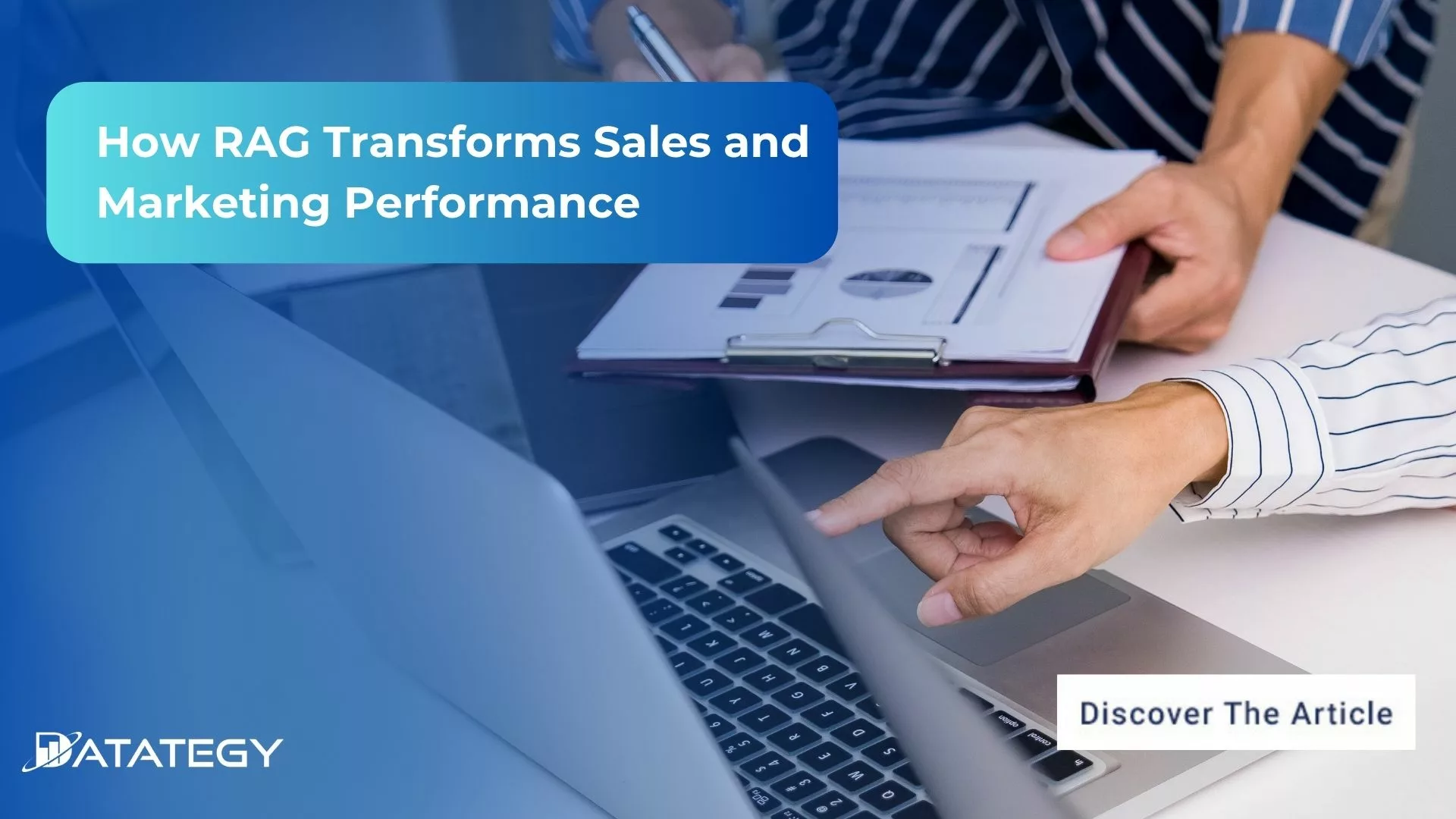
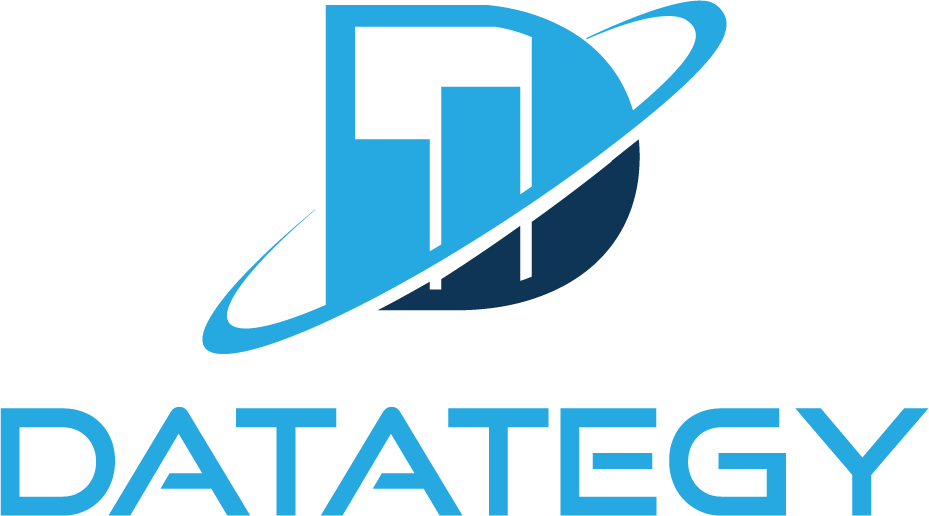