Transform Defense Logs & Rapport into Situational Awareness with AI...
Read MoreCardiovascular disease prediction using papAI
Table of Contents
ToggleThe heart is a hollow muscle that ensures the body’s blood circulation. It supplies all tissues with oxygen and removes metabolic waste products such as carbon dioxide (CO2). Despite considerable progress in treatment and prevention, cardiovascular disease is still the leading cause of death in the world with more than 17 million deaths annually.
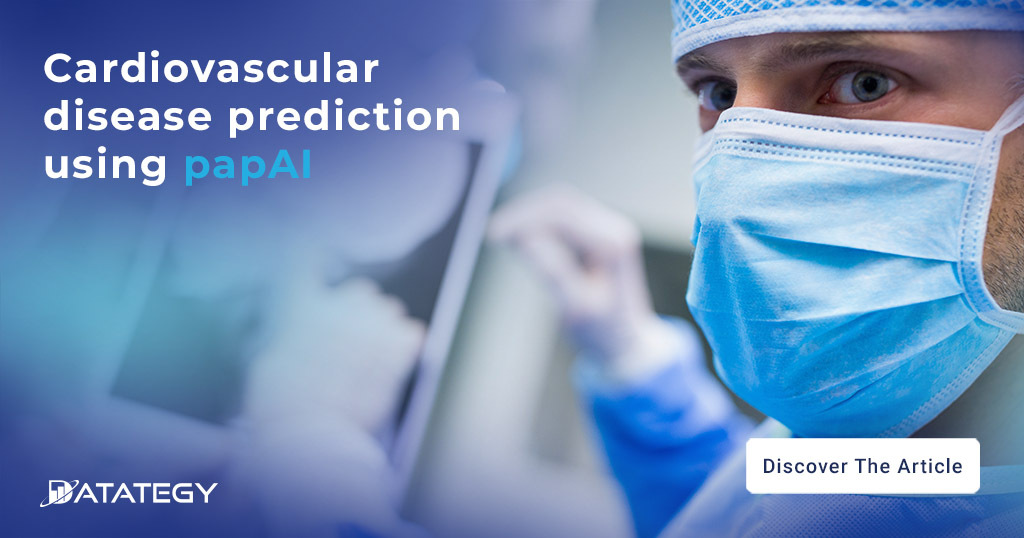
Cardiovascular disease affect more than 30% of hospital patients, and the older people are more exposed to them. Therefore, its prevention and treatment are a major public health issue. We studied several Machine Learning models to analyze the patient’s data and we found a correlation between the different symptoms. There is a lot of data available regarding the health of the patients and they need a tool that can analyze it quickly, in order to know if the patient has a cardiovascular disease or not.
Cardiovascular risk factors
- Age and gender : men over 50 and women over 60 are more likely to suffer a cardiovascular or cardiac event
- Family history of cardiovascular disease : the cardiovascular risk increases if a close relative such as a father, mother, brother or sister has already suffered from cardiovascular disease at an early age
- Excess cholesterol : when cholesterol levels are too high, it accumulates on the artery walls. These fatty deposits eventually form atheromatous plaques, which can then impede or block blood flow
- Obesity and overweight : the cardiovascular risk increases even more when the excess fat is in the belly and waist area
- Chronic stress : Chronic stress can have important consequences for physical and mental health, including an increased risk of developing cardiovascular disease.
1- Identifying the target
In our case, we need to identify the target: our goal is to predict the appearance of any heart disease in a certain population. We must declare a positive outcome as someone having the risk of getting the disease. Then we can determine what are the risk factors of developing it.
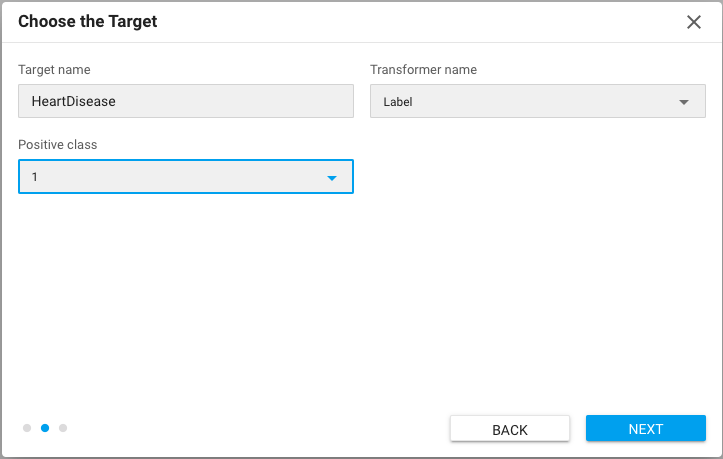
2- Configure the models
A first step to build our model is the selection of features so we can understand their relationship with the target and apply it into the model training.
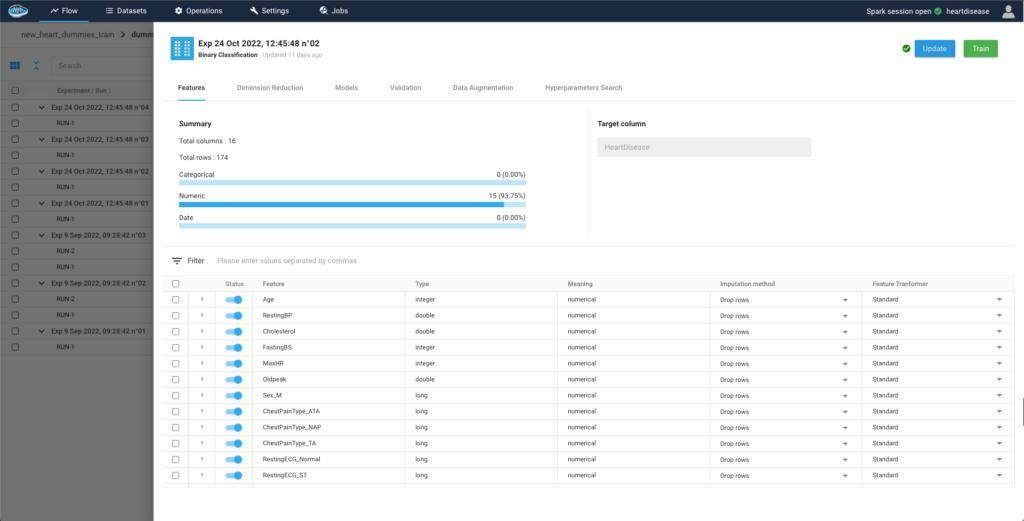
Afterwards, we select the models that could be compatible with the problem we want to solve. papAI integrates a large catalogue of ready-to-use models to easily build, train and deploy an AI pipeline. For more complex projects, papAI also provides an AutoML feature
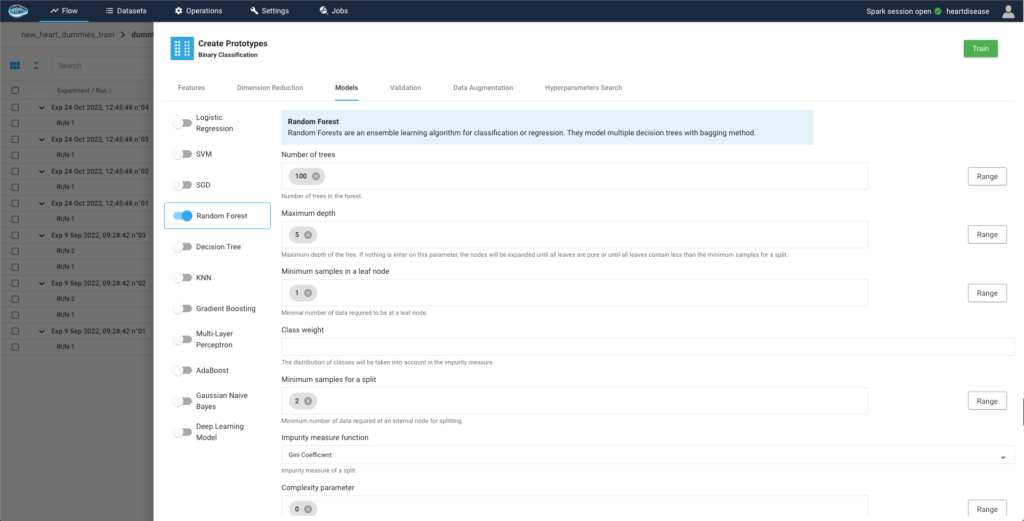
3- Evaluate the trained experiments
After running an experiment comes the evaluation process. We must identify its effectiveness and its performance when predicting the target through a series of metrics implemented into papAI’s interface. For example, evaluation metrics include accuracy, density chart, confusion matrix …
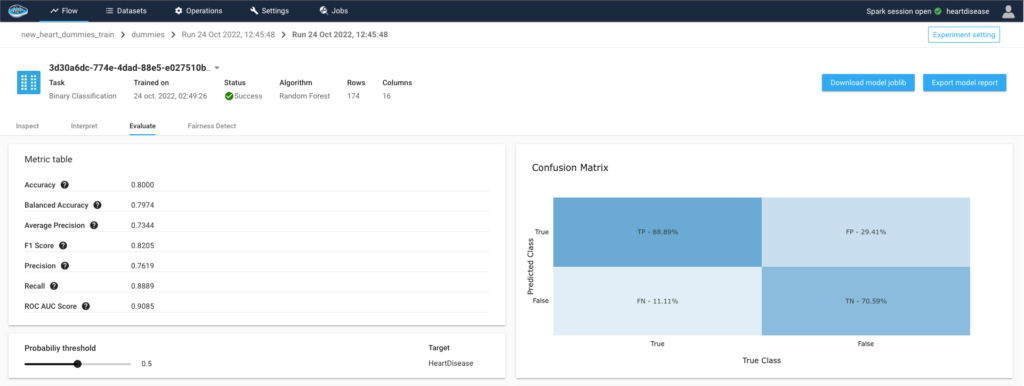
Through the density chart we can clearly see the ability for the model to determine the difference between both classes, people with or without heart disease, at a median threshold of 0.5, meaning that model successfully detected the two population independently.
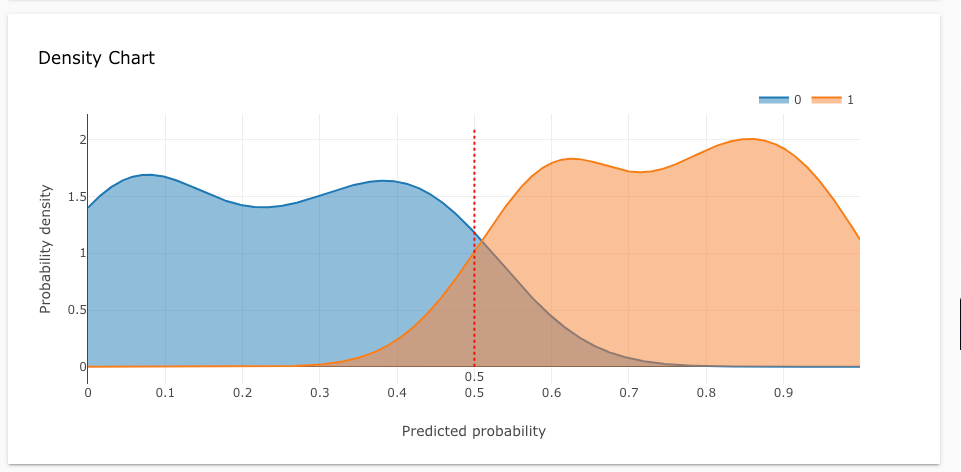
4- Interpret the predicted outcome
The evaluation process goes along with the explainability part to understand the model’s behaviour and the influence of each variable on the prediction’s probability. We can then assess the probable risk factors of having an heart disease.
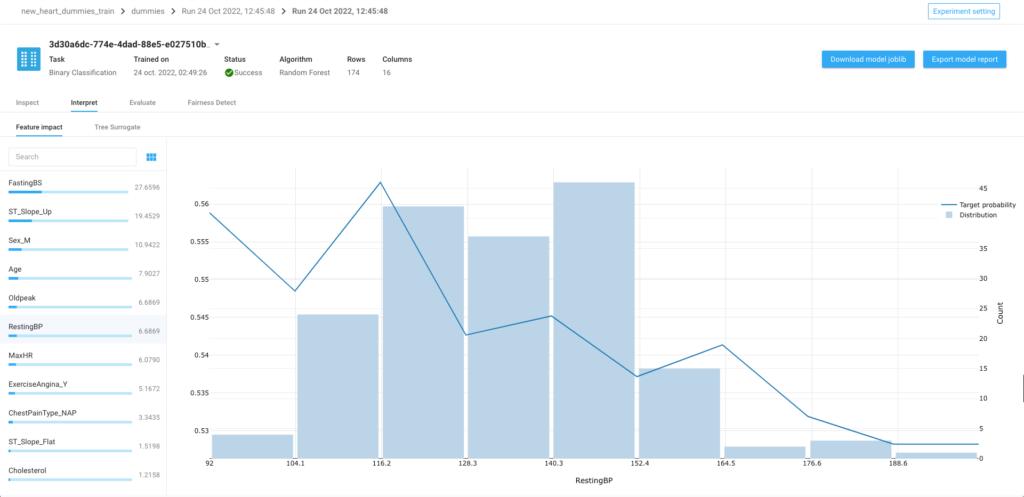
After going through these important processes, a model is chosen and promoted in order to apply it on an testing dataset and obtain individual predictions with a local interpretability. Thanks to this local interpretability, we can deeply understand the decision made by the model and try to develop cases to modify the predicted outcome.
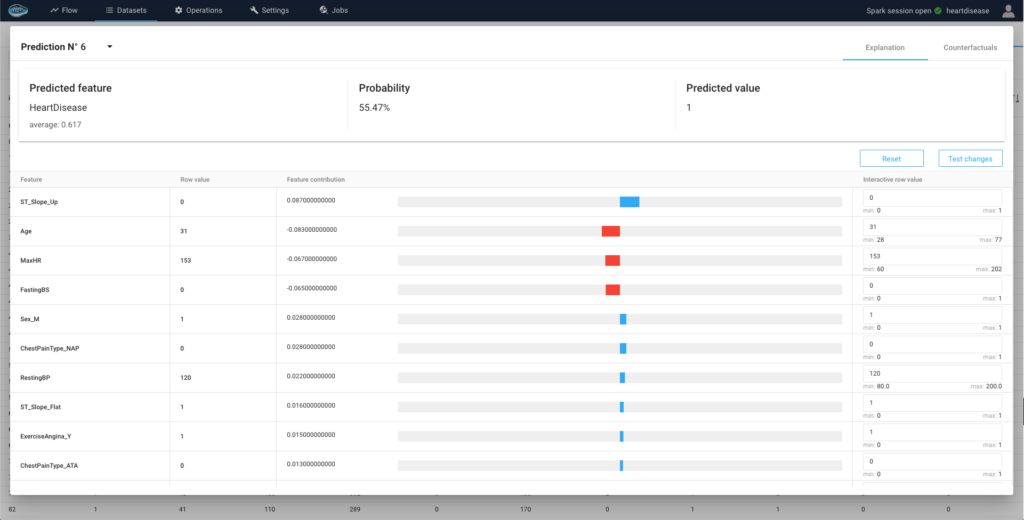
Because of organizational and structural issues, the treatment of the patient by healthcare professionals could be delayed and thus leading to a higher mortality rate. It is therefore important to better detect people at risk in order to offer them faster and personalized care. The papAI platform provides concrete help in detecting patients with cardiovascular diseases with a solution that offers a high degree of explainability of the results in order to identify as precisely as possible the factors to be monitored.
Interested in discovering papAI ?
Our commercial team is at your disposal for any question
Why AIOps Is Key to Cyber Threat Detection in Defense?
Why AIOps Is Key to Cyber Threat Detection in Defense?...
Read MoreHow AI Transforms Predictive Maintenance in Defense Equipment
How AI Transforms Predictive Maintenance in Defense Equipment In a...
Read More