Transform Defense Logs & Rapport into Situational Awareness with AI...
Read MoreFraud Detection in Energy: Using AI to Detect Fraudulent Energy Billing Practices
Table of Contents
ToggleThe energy sector is quite concerned about fraudulent energy billing practices since they result in millions of dollars being lost every year. The Association of Certified Fraud Examiners (ACFE) reported that fraud costs companies an average of 5% of their annual sales. False invoicing techniques are common in the energy sector, and it is claimed that only energy theft costs the world $90 billion annually. It might be difficult to identify fraudulent billing practices, but with the development of artificial intelligence (AI) and machine learning, energy companies can now use powerful data analytics to identify and stop fraud.
Find out how papAI can improve the deployment of AI projects in the Energy industry here.
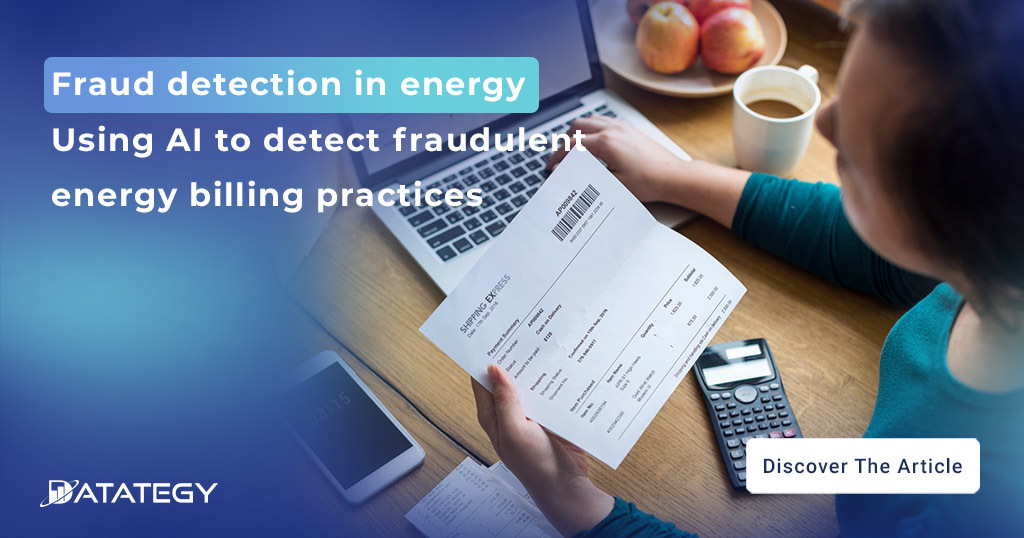
In this article, we will discuss how AI-based fraud detection techniques can help energy companies detect fraudulent energy billing practices, leading to significant cost savings and promoting sustainable growth in the energy sector.
The Impact of Fraudulent Energy Billing Practices
Fraudulent energy billing practices have a significant impact on the energy sector, with consequences ranging from financial losses to reduced energy access for consumers. These practices can occur at any point in the energy value chain, from energy production to distribution and billing
1- Financial losses for energy companies
Energy companies’ financial losses are among the most glaring effects of illegal energy billing practices. Fraudulent activities might include altering energy meters and fabricating statistics on energy use. Such actions might result in incorrect accounting, costing energy providers money that was rightly theirs. Organizations lose an average of 5% of their annual income to fraud, according to a research by the Association of Certified Fraud Examiners (ACFE), and the energy industry is no different.
2- Effect on the Development of the Energy Sector
The expansion and development of the energy sector may be negatively impacted by dishonest energy billing practices. With its ability to power companies, create jobs, and promote social progress, the energy industry is a vital contributor to economic growth and development. Yet, dishonest behavior can undermine these initiatives, resulting in a decline in investment, a lack of faith in energy systems, and a general loss of confidence in the industry.
3- Customers' Access to Energy is Restricted
Consumers may also be impacted by fraudulent energy billing practices, especially if they are already having trouble accessing electricity. Fraudulent actions often cause consumers’ energy bills to grow, which they might not be able to pay. Consumers who are unable to acquire the energy they require to satisfy their fundamental needs may therefore experience energy poverty as a result of this. Health issues, fewer educational possibilities, and diminished economic production are just a few of the negative effects of energy poverty.
4- Increased Legal and Regulatory Risks
Energy companies may face legal and regulatory concerns as a result of fraudulent energy billing practices. Regulation violations, such those involving billing and pricing, may incur fines, penalties, and legal proceedings, resulting in further monetary losses and reputational harm.
Empowering the Energy Industry through AI Revolution: The Critical Role of CDOs
Chief Data Officers (CDOs) in the energy industry face several challenges when it comes to adopting AI technologies. There is a cultural shift that must occur within energy companies to embrace AI technologies. CDOs must continue to explore and implement AI technologies to improve efficiency, reduce costs, and meet sustainability goals.
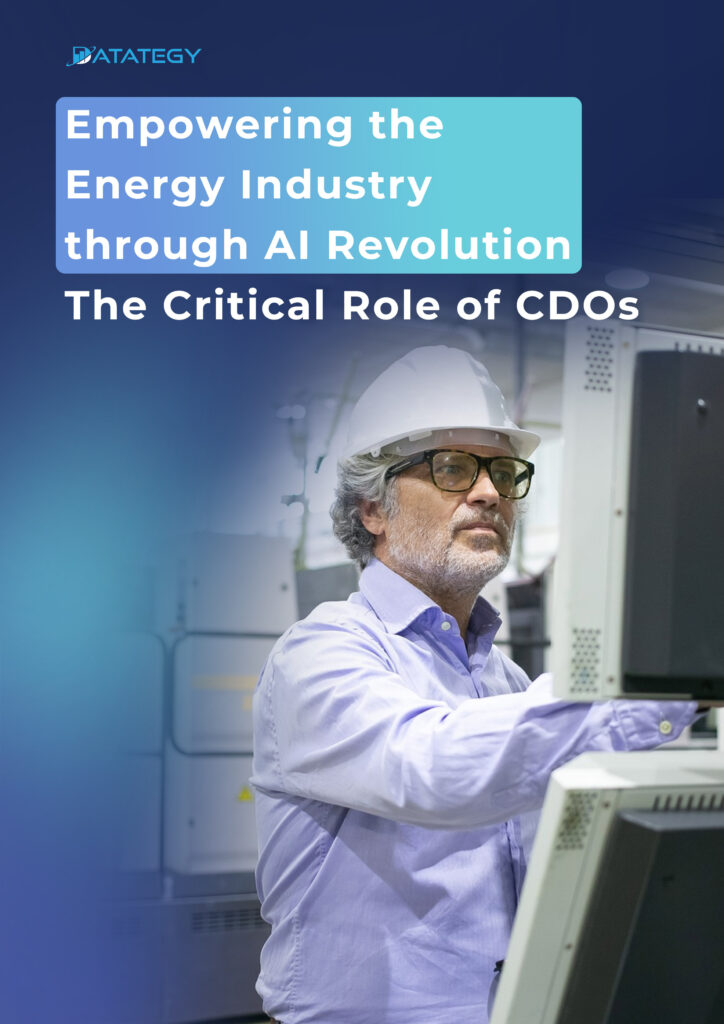
Advanced AI Techniques to Detect and Prevent Fraud
1- Machine Learning Algorithms
Large volumes of energy usage data may be analyzed using machine learning algorithms to look for trends and anomalies that can point to fraud. With the use of these algorithms, it is possible to spot irregularities in energy consumption patterns and unexplained behavior, including energy theft, meter manipulation, and billing fraud. Energy firms may create a strong fraud detection system by applying machine learning algorithms that can be taught using historical data to discover trends and anomalies.
2- Natural Language Processing (NLP)
To detect possible fraud, NLP approaches may be used to evaluate text-based data like emails, chat logs, and customer reviews. NLP algorithms may examine the tone and mood of customer reviews to spot odd trends and spot probable fraud incidents. This method can be especially helpful in spotting instances of social engineering, in which fraudsters may try to persuade victims to divulge critical information.
3- Image and Video Analysis
Physical signs of fraud, such as manipulated energy meters or unauthorized connections to the energy grid, can be found using image and video analysis. Energy companies can take action and stop fraud by using AI-based image and video analysis to spot anomalies and identify suspect conduct.
4- Predictive Analytics
Future energy consumption trends may be predicted using predictive analytics approaches, which can also be used to spot possible fraud situations early on. These methods forecast future trends using previous data, enabling energy corporations to spot patterns that could point to fraud. Energy companies may reduce revenue loss and safeguard their clients by detecting probable fraud incidents early.
Real-world Examples of AI-based energy billing Fraud Detection
Example 1: Pacific Gas and Electric Company In 2014, Pacific Gas and Electric Company (PG&E) implemented an AI-based fraud detection system to identify energy theft and other fraudulent activities. The system analyzed customer data and usage patterns to identify potential fraud cases, and PG&E personnel were notified when potential fraud was detected.
The system was successful in identifying a wide range of fraudulent activities, including meter tampering and energy diversion. As a result, PG&E was able to recover significant amounts of revenue and prevent further losses due to fraud. The company also saw improvements in customer satisfaction and trust, as customers were reassured that PG&E was taking steps to prevent fraudulent activities.
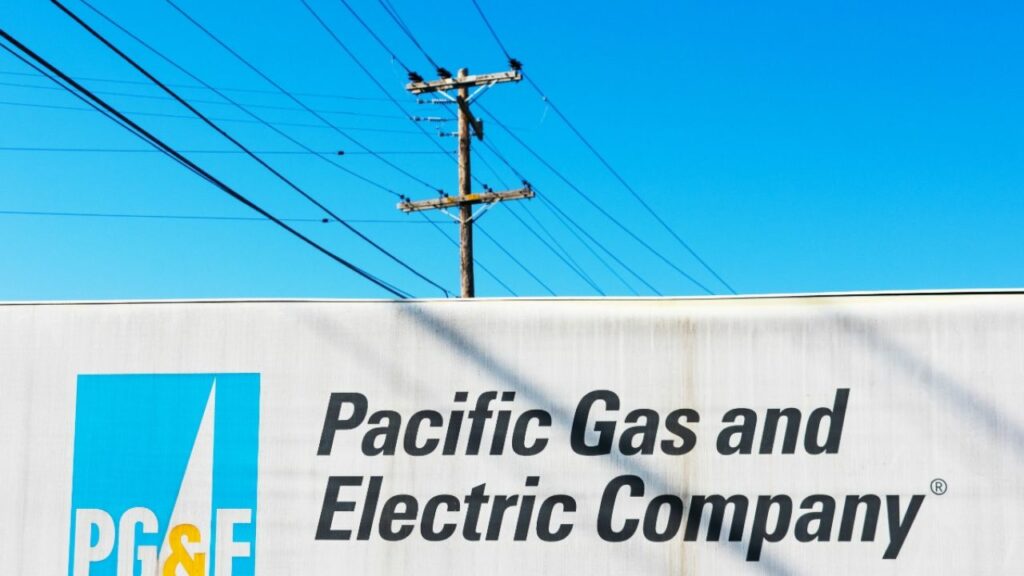
Example 2: Southern Company Southern Company is a leading energy provider in the southeastern United States, and it has also implemented an AI-based fraud detection system. The system uses machine learning algorithms to analyze energy usage patterns and identify potential fraud cases. The system has been successful in identifying a wide range of fraudulent activities, including billing fraud and energy diversion.
The solution has significantly impacted Southern Company’s business operations, enabling it to recoup lost money and stop more losses brought on by fraudulent activity. While clients are satisfied that Southern Company is taking measures to stop fraudulent activity, the firm has also observed gains in customer satisfaction and confidence.
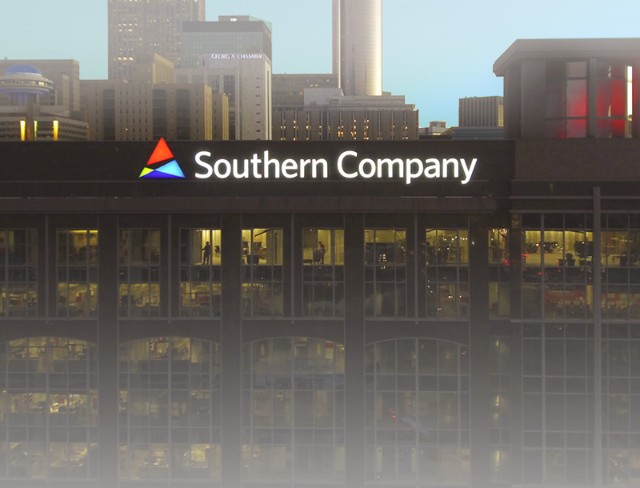
Example 3: Enel is an Italian energy company that has also implemented an AI-based fraud detection system. The system analyzes energy usage data in real-time and uses machine learning algorithms to identify potential fraud cases. The system has been successful in identifying a wide range of fraudulent activities, including meter tampering and energy diversion.
Enel’s operations have been significantly impacted by the technology, which has allowed the business to recoup lost money and stop additional losses brought on by fraudulent activity. When clients are satisfied that Enel is taking measures to stop fraudulent actions, the firm has also observed gains in customer satisfaction and confidence.
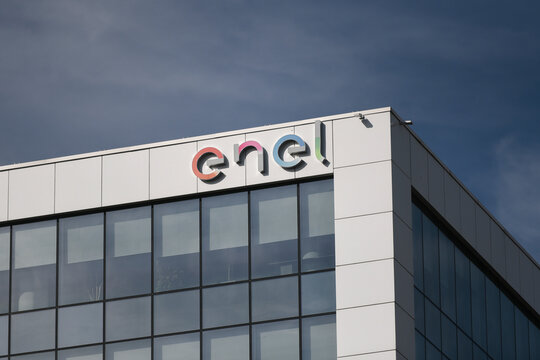
Benefits of using AI-based fraud detection
More accuracy: Compared to conventional approaches, AI-based fraud detection solutions can evaluate huge amounts of data with better precision. Energy usage data may be analyzed by machine learning algorithms to find trends and abnormalities, which enables the early identification of fraud.
Speed: Rapid data processing capabilities of AI-based fraud detection algorithms allow energy businesses to react in real-time to suspected fraud incidents. This can assist in stopping additional revenue losses and preserving the company’s name.
Scalability: Depending on the demands of the business, AI-based fraud detection systems may be scaled up or down. This enables energy firms to manage heavy data loads at peak times without affecting the system’s accuracy.
Reduce manual efforts: AI-based fraud detection approaches automate the fraud detection process, eliminating the need for manual inspection and analysis. This reduces manual work and operating expenses. By doing this, energy businesses may save time and money and concentrate on other important business areas.
Increased customer satisfaction and trust: By identifying and thwarting fraudulent activity, the use of AI-based fraud detection systems may improve consumer happiness and confidence. Improved customer satisfaction can result from this, which is important for energy firms wanting to keep a competitive edge in the market.
Build your AI-based Energy Billing Fraud Detection Tool using papAI platform
By implementing these techniques, energy companies can ensure that they remain competitive, protect their revenue, and build trust with their customers. As AI technology continues to advance, the potential for energy billing fraud detection will only grow, providing energy companies with new and innovative ways to detect and prevent fraudulent activities.
Among the various AI platforms available, papAI stands out as a powerful tool for energy companies looking to build their own AI-based Energy Billing fraud detection tool. With papAI, energy companies can easily customize their fraud detection algorithms to their specific needs, using a drag-and-drop interface to build models that detect fraud with high accuracy. papAI also supports real-time data processing, allowing for immediate detection of fraudulent activity and rapid response to prevent losses. Furthermore, papAI is user-friendly and does not require extensive technical expertise, making it accessible to energy companies of all sizes.
If you’re interested in learning more about the papAI platform and how it can help you build your own tool, you can book a demo with one of our AI experts. Our team can show you how the platform works and help you get started on your own.
Interested in discovering papAI?
Our commercial team is at your disposal for any questions.
Why AIOps Is Key to Cyber Threat Detection in Defense?
Why AIOps Is Key to Cyber Threat Detection in Defense?...
Read MoreHow AI Transforms Predictive Maintenance in Defense Equipment
How AI Transforms Predictive Maintenance in Defense Equipment In a...
Read MoreHow to Scale AI Without Breaking Your Infrastructure in 2025
How to Scale AI Without Breaking Your Infrastructure in 2025...
Read More