Transform Defense Logs & Rapport into Situational Awareness with AI...
Read MoreCustomer Churn Prediction in Telecom Industry Using AI: A Data-Driven AI Approach
Table of Contents
ToggleIn order to examine massive data, such as data consumption, call recording, and application usage, artificial intelligence (AI) in the telecoms industry utilizes software and algorithms that enhance decision-making. Additionally, AI supports telecommunications companies by assisting with virtual assistance, network security, and defect detection.
AI makes it simpler for the telecommunications sector to manage daily operations, address issues more effectively, and increase customer satisfaction by allowing information to be extracted from their massive data sets. Therefore, how AI may increase client retention is the focus of our article.
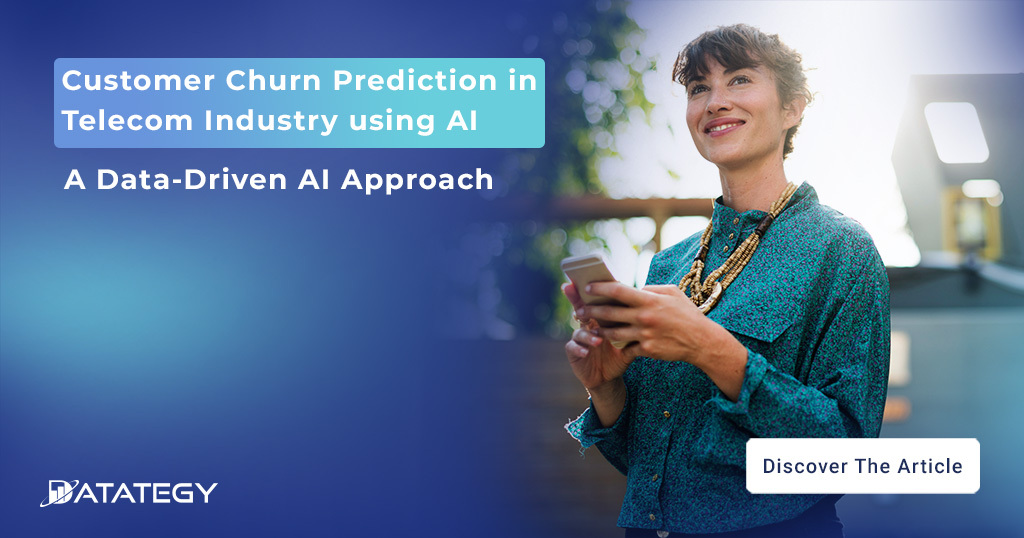
According to a McKinsey study, telecom companies that have used AI predictive analytics have reduced customer churn by 15-20%. In this article, we examine how papAI solution can help predict customer churn.
Overview of AI in the Telecom Industry
The global AI in telecommunication market size was valued at $1.2 billion in 2021 and is projected to reach $38.8 billion by 2031, growing at a CAGR of 41.4% from 2022 to 2031.
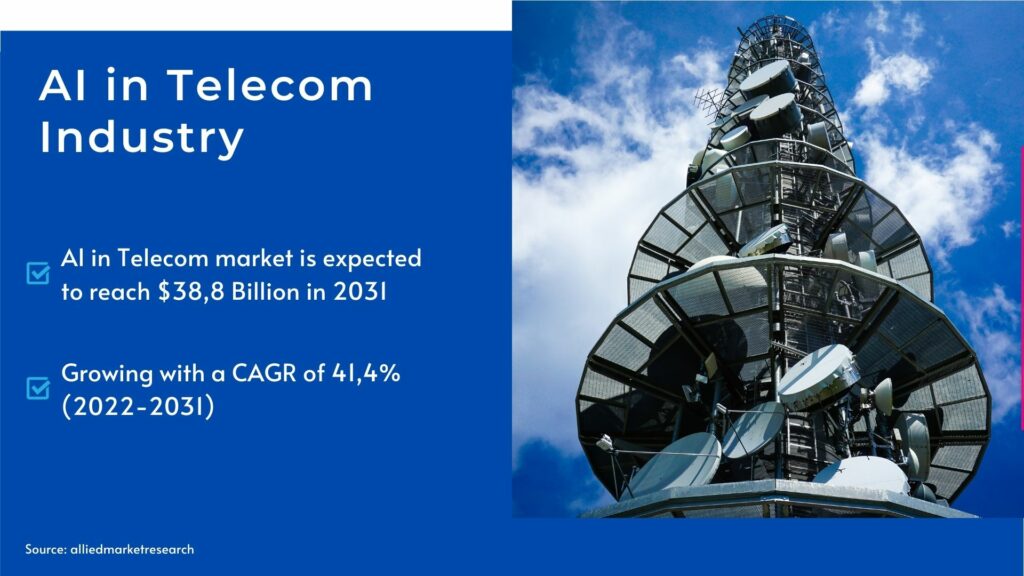
A Regional Perspective on AI in Telecom
Due to the evolving technology and communications sector in the region, which is expected to fuel the telecom AI market during the forecast period, North America dominated the global telecom AI market in 2022 with a valuation of $0.4 Billion and is expected to maintain its position during the forecast period.
However, due to its growing digital capabilities and a fiercely competitive industry, Asia Pacific is expected to witness considerable development during the forecast period, which is expected to drive the growth of the region’s AI in the telecom market in the coming years.
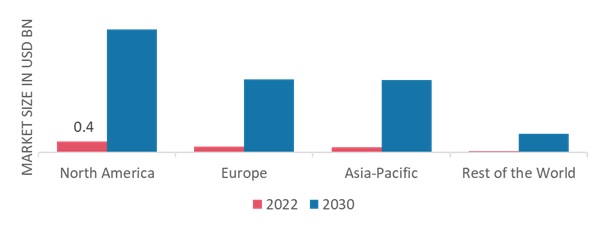
Source: Secondary Research, Primary Research, MRFR Database.
What Does Customer Churn Mean in the Telecom Industry?
In the telecom sector, the occurrence when clients cancel their subscriptions or change to a new service provider is referred to as “customer churn.” It is a measurement of the rate at which clients switch from one telecom provider to another. Churn can happen for a number of reasons, such as unhappiness with the service, problems with the network’s quality, a lack of individualized services, or the availability of cheaper prices from rivals. Customer churn is a major issue for telecom firms since it may result in lost sales, a decline in market share, and harm to a company’s reputation. Therefore, for telecom enterprises to succeed and survive in the long run, it is essential to identify and manage customer churn.
The Benefits of AI in Telecom Industry
The capacity of AI to effectively forecast and identify consumers who are most likely to churn in the telecom business, notably in connection to customer turnover, is one of its key advantages. In order to spot minor trends and churn signs, AI systems can analyze enormous volumes of consumer data, including demographics, use patterns, customer service interactions, and past behavior.
Telecom firms may proactively engage with tailored offers, discounts, or enhanced services to keep at-risk clients by utilizing AI. This focused strategy greatly enhances the efficacy of churn prevention techniques, resulting in better rates of customer retention and higher levels of customer satisfaction. In the end, AI enables telecom businesses to use proactive strategies to reduce churn, improve client retention, and spur company development.
Leading the data revolution: CDO role in today's organizations
The need for CDOs is expanding as a result of the growing significance of data in today’s corporate environment. Any organization’s success depends on the CDO, who is the primary force behind digital innovation and change.
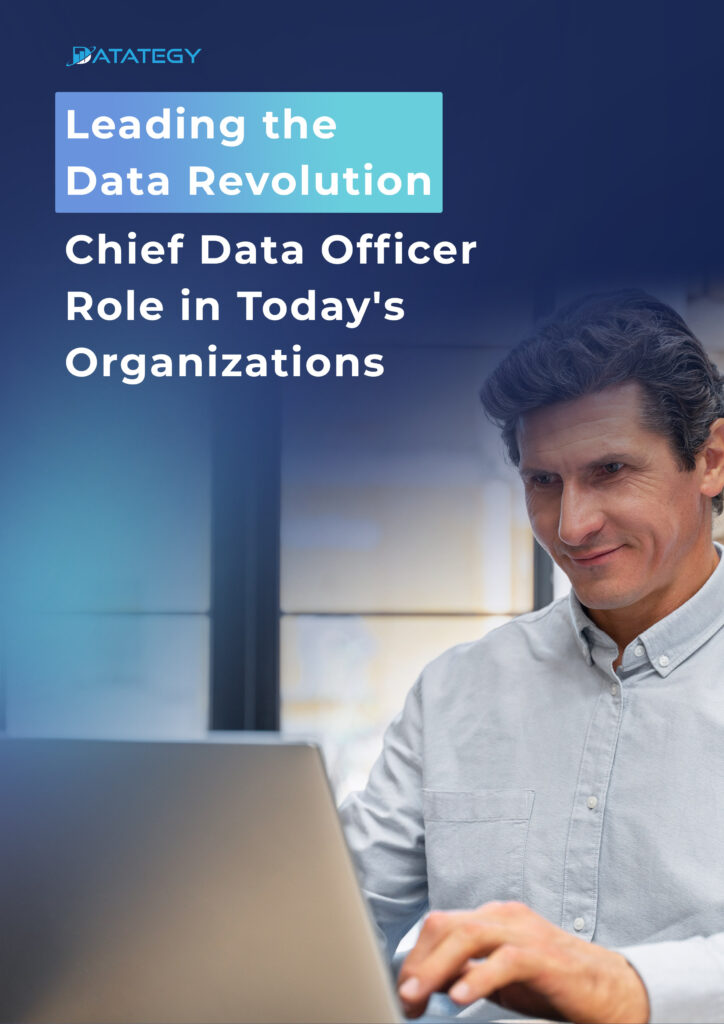
Case Study: How papAI Solution can Enhance Customer Churn Prediction?
For the purpose of our analysis, we used a Teleco dataset representing different churn data from telco customers.
The data set includes information about:
– Customers who left within the last month – the column is called Churn
– Services that each customer has signed up for – phone, multiple lines, internet, online security, online backup, device protection, tech support, and streaming TV and movies
– Customer account information – how long they’ve been a customer, contract, payment method, paperless billing, monthly charges, and total charges
– Demographic info about customers – gender, age range, and if they have partners and dependents
The Model We Will Use
As a strong statistical tool, survival analysis offers a thorough framework for examining customer attrition in a variety of sectors, including telecoms. Survival analysis allows us to examine the time until an event of interest, such as the churn of telecom customers, as opposed to traditional analysis approaches, which concentrate on static outcomes.
We can better understand the timing and factors that contribute to customer loss by analyzing survival curves, risk rates, and associated statistical models. This will allow businesses to proactively identify and address churn, enhance retention strategies, and ultimately improve customer satisfaction and profitability.
The Analysis's papAI Strengths
Applying papAI survival models ML task on the telco churn dataset, on the tenure (number of months a customer kept his subscription).
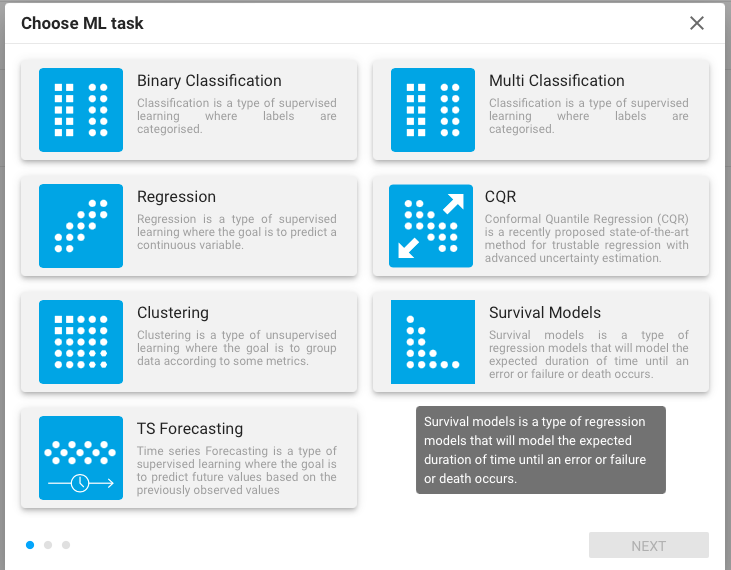
We gain insights into how different factors affect the timing and likelihood of customer attrition.
Hazard Ratio
The hazard ratio (HR) is a measure of the relative risk associated with a specific covariate in the Cox proportional hazards model. It quantifies the change in the hazard rate (risk of an event) between two groups, typically defined by different levels of a covariate
Features Coefficient
This plot is used to compare the effect of each covariate used in the model on the hazard ratio (HR). If HR > 1, the covariate is a risk factor, else if HR < 1 then the covariate is a protective factor on survival and else if it is equal to 1 then it has no effect on survival.
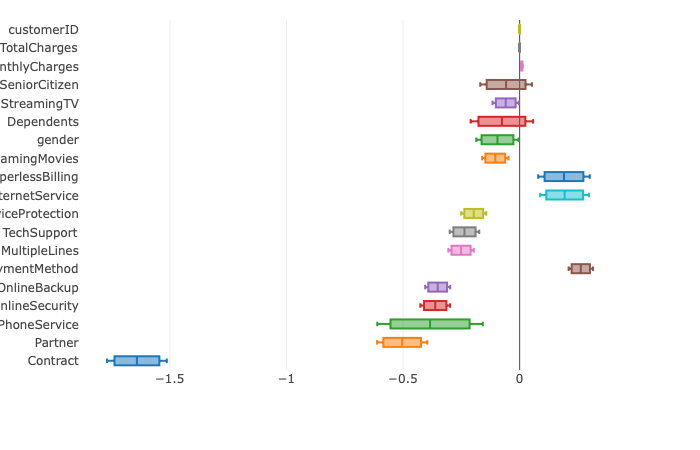
This box plot enables us to clearly see the effect of each feature on survival (churn).
Different Features Effects on Churn (Feature Selection)
We use the survival function plot to check the effect of different features on churn. The x axis represents the months a customer kept his subscription, and the y axis the probability that the customer keeps his subscription
Contract:
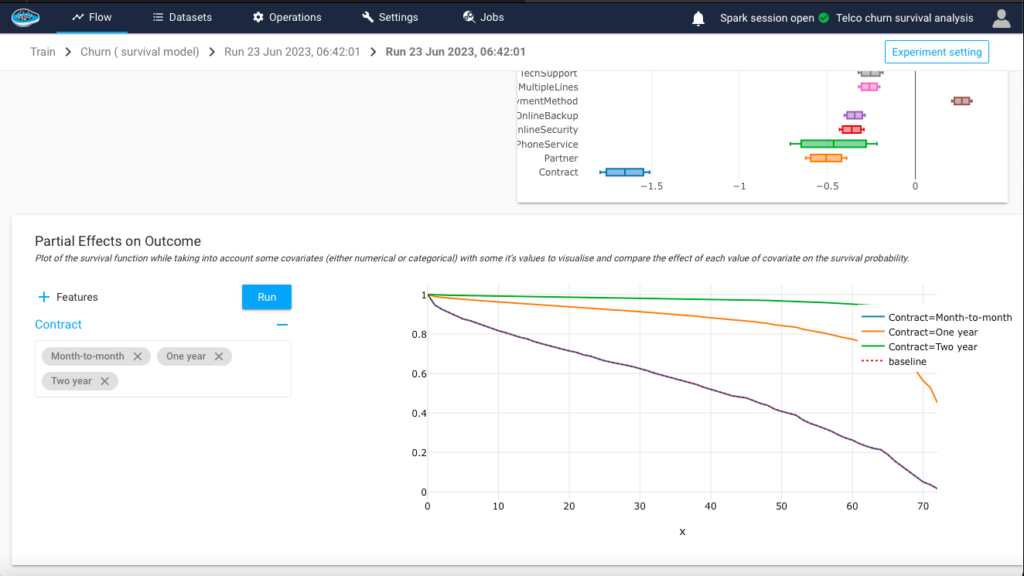
Upon analyzing the data, it is evident that customers with long-term contracts exhibit a higher likelihood of remaining with the company. This finding suggests that the contract duration plays a significant role in customer retention.
Phone Service:
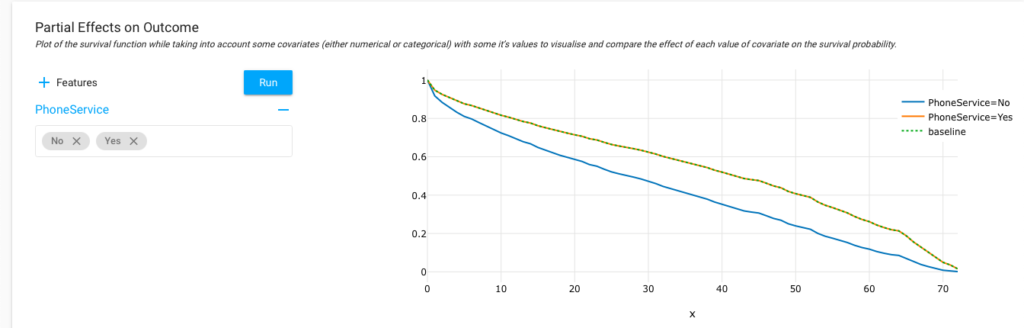
We notice that a user with no phone service is more likely to quit his subscription.
Partner:
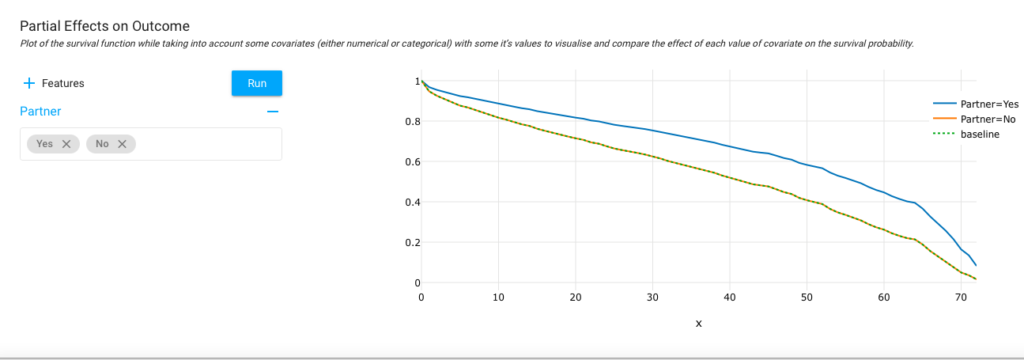
We can also notice that a user who has a partner is more likely to stay longer with the company.
Gender:
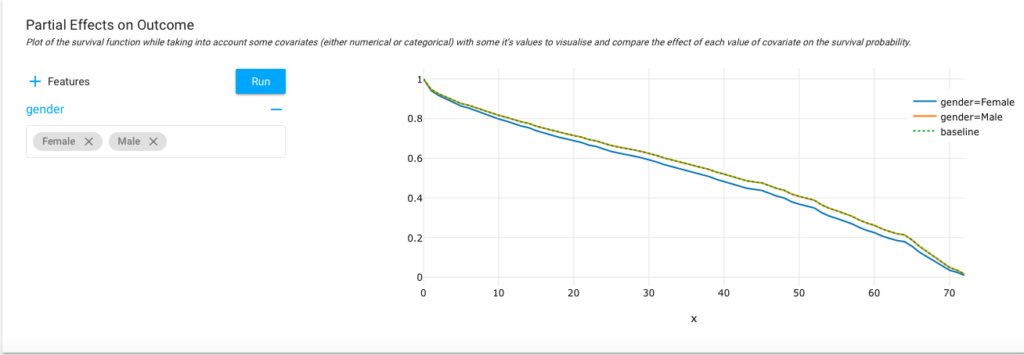
Based on the analysis conducted, it appears that there is no significant difference between the two genders in their tendency to churn. The churn rates for both genders appear to be similar, suggesting that gender alone may not be a strong predictor of churn in this telco dataset.
Payment method
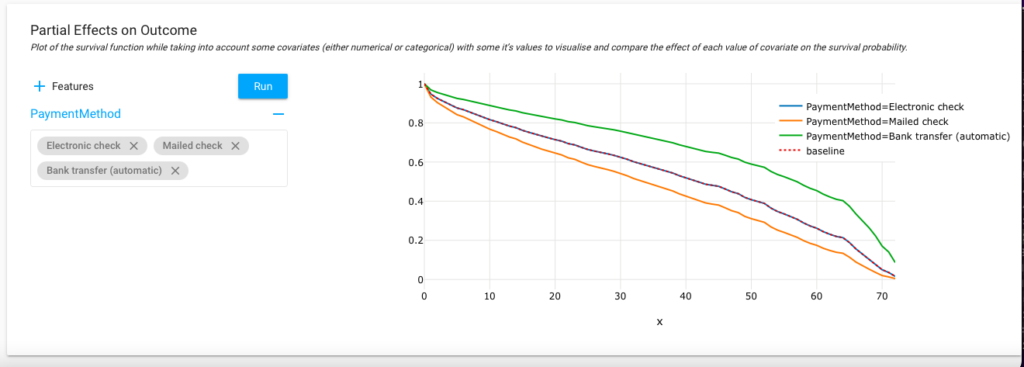
We can also observe that customers who utilize bank transfer automatic payments for their subscriptions are more inclined to remain subscribed compared to those who opt for more traditional payment methods. This phenomenon can potentially be attributed to customers’ tendency to overlook automatic subscriptions, leading to continued payments without regular evaluation.
Metrics
Metrics are an important measure of an ML model performance , papAi offers various metrics concerning each run of the ML model, for our specific run using Cox Proportional Hazard algorithm, we have 3 of them :
Aic (Akaike’s Information Criterion): Rewards goodness of fit used for comparing models , models with the lower AIC value is considered a better fit to the data.
Concordance index: It assesses the concordance or agreement between the predicted survival times and the actual observed survival times of individuals in a dataset.
A C-index of 0.5 indicates a model that performs no better than random chance, while a C-index of 1.0 represents a perfect predictive model. Generally, a C-index above 0.7 is considered acceptable, and higher values indicate better predictive accuracy.
Log-likelihood ratio test: The log-likelihood ratio test provides a formal statistical framework to evaluate the significance of including or excluding covariates in survival analysis models and helps in model selection and refinement

Enhance the Accuracy of Customer Churn Prediction Projects by Building Your Own AI-based Tool with papAI Solution
papAI Solution has played a pivotal role in enabling us to conduct a comprehensive churn analysis in the telecom industry. By leveraging the platform’s capabilities, we were able to explore and analyze large volumes of customer data efficiently and effectively.
The platform provided us with the necessary tools to preprocess, visualize, and model the data, allowing us to uncover valuable insights into customer churn dynamics. Additionally, the platform’s integration with survival analysis techniques, such as the Cox proportional hazards model, empowered us to delve deeper into the timing and drivers of churn, considering the time-to-event nature of the data. This enabled us to identify significant predictors of churn and understand their impact on customer retention.
The flexibility, scalability, and robustness of our data analysis platform have facilitated a comprehensive understanding of telco churn, empowering businesses to make data-driven decisions, improve customer retention strategies, and ultimately drive growth and success in the competitive telecommunications landscape.
Want to find out more about the functionality of papAI Solution? book your demo now. Our team of AI experts can help you create a customized AI-based tool that meets the unique needs of your organization.
Interested in discovering papAI?
Our commercial team is at your disposal for any questions
Why AIOps Is Key to Cyber Threat Detection in Defense?
Why AIOps Is Key to Cyber Threat Detection in Defense?...
Read MoreHow AI Transforms Predictive Maintenance in Defense Equipment
How AI Transforms Predictive Maintenance in Defense Equipment In a...
Read MoreHow to Scale AI Without Breaking Your Infrastructure in 2025
How to Scale AI Without Breaking Your Infrastructure in 2025...
Read More