Transform Defense Logs & Rapport into Situational Awareness with AI...
Read MoreElevating Marketing Agility:
Enhancing Marketing Strategy through Machine Learning-Driven Customer Segmentation
Table of Contents
ToggleMachine learning (ML) is a powerful tool that is causing a major shift in the landscape of customer segmentation. This ground-breaking methodology is introducing a new age of advertising tactics distinguished by personalized and dynamic methods. Machine learning is redefining the basic core of conventional procedures by utilizing cutting-edge algorithms and thorough data analysis.
The impact is apparent, according to research from Evergage: 88% of marketers have seen a significant improvement in their marketing efforts as a result of integrating machine learning-backed personalization.
Find out how papAI can improve the deployment of AI projects in Sales & Marketing.
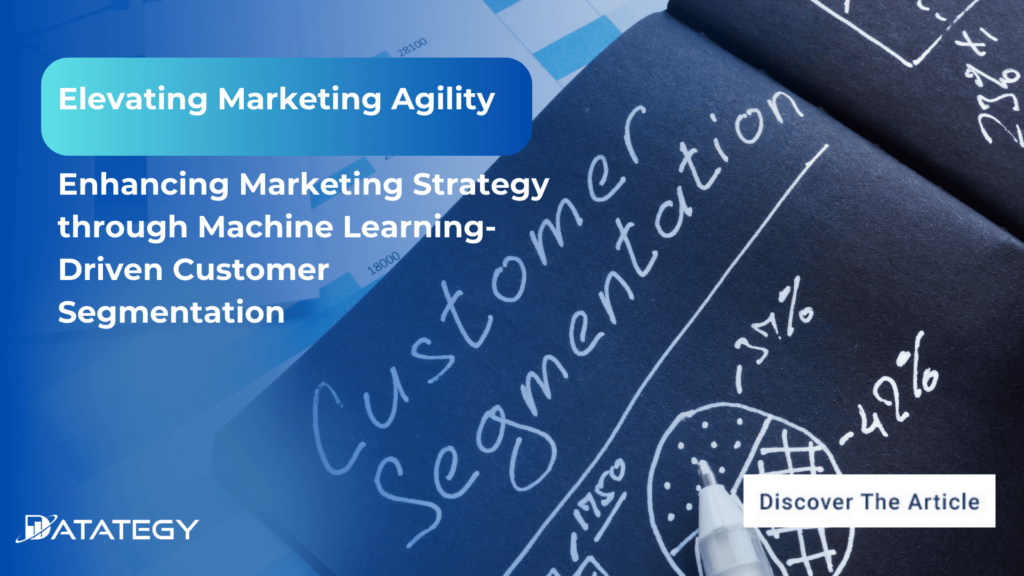
In this article, we will delve into the fascinating world of machine learning using papAI and its huge impact on the intricate details of customer segmentation.
What Does Machine Learning (ML) Mean?
Machine learning is a branch of computer science and artificial intelligence (AI) that focuses on utilizing data and algorithms to simulate human learning, gradually improving the system’s accuracy.
Machine learning is a crucial component of data science, a rapidly increasing field. In order to provide classifications or predictions and uncover crucial insights in data mining projects, algorithms are trained using statistical approaches.
Ideally, the choices taken as a result of these insights affect important growth metrics in applications and businesses. As big data continues to grow and improve, data scientists will become increasingly in demand. They will be required to help identify the most important business concerns and the
Customer Segmentation: How Important Is It?
Customer segmentation is crucial to the success of every business. It comprises dividing the target market into a number of groups based on characteristics, routines, or interests that they have in common. By being aware of the different needs and motivations of distinct customer groups, businesses can tailor their marketing strategies and offerings to effectively engage and convert their target audience. For the following reasons, customer segmentation is crucial:
Personalized Communication: Customers of today want customized service. Businesses may communicate with certain segments of their consumer base in a relevant and tailored way that speaks to their individual requirements, goals, and interests. By personalizing their communication, businesses may foster closer bonds with their customers, foster greater brand loyalty, and forge long-lasting connections.
Targeted marketing: By segmenting their customer base into discrete groups, businesses may focus their resources and efforts on the customers most likely to be interested in their products or services. Knowing the specific needs and preferences of each group allows businesses to create customized marketing campaigns that engage their audience, raise conversion rates, and boost return on investment.
More Effective Customer Retention: By segmenting their customer base, businesses may identify the most crucial clientele groups and allocate resources to strengthen relationships with these groups. Giving customers personalized experiences and persistently addressing their shifting needs may help businesses enhance their client retention rates. More brand evangelists and recommendations from loyal and content customers will drive the business’s future growth.
Allocating Resources Effectively: Resource allocation is made more effective by customer segmentation. You may maximise your cash, time, and energy for the best impact by concentrating your marketing efforts on high-potential categories.
Limitations of Conventional Customer Segmentation
Although traditional customer segmentation approaches have been widely used in the past, they have a number of limitations that make them less relevant in the current fast-paced business environment. Some important issues with traditional customer segmentation include the following:
1- More Segmentation Criteria
Typical demographic variables utilized in conventional customer segmentation include age, gender, and location. Although these metrics do provide some insights, they do not adequately represent the complexity and richness of customer behavior. Customers today are affected by a variety of factors, including online interactions, social media presence, and digital touchpoints. A sole concentration on demographic data may lead to a lack of understanding of customer behavior and preferences.
2- Lack of up-to-date data
Traditional customer segmentation places a strong emphasis on historical data, which offers insights into previous behavior and preferences. Although previous data is useful, it may not be able to forecast client behavior in the future. Relying entirely on previous performance may restrict the capacity to predict and address changing client demands because market dynamics and customer preferences are both dynamic. Future trend identification is a proactive task for businesses, and standard categorization could not offer the required foresight.
3- Uncertainty Regarding Future Behaviour
Traditional customer segmentation focuses a lot of weight on historical data since it provides information about past preferences and behavior. Although historical data is valuable, it might not be able to predict future customer behavior. Because both market dynamics and consumer preferences are dynamic, relying solely on past performance may limit the ability to anticipate and respond to changing client wants. Identification of future trends is a proactive responsibility for firms, and conventional categorization might not provide the necessary foresight.
Opportunities and Trends for Future Customer Segmentation
1- Segmenting using Several Channels
In today’s omnichannel world, customers interact with businesses through a multitude of touchpoints, including websites, social media, mobile applications, and physical shops. Cross-channel segmentation combines consumer data from these many sources to present a unified customer picture. This enables businesses to deliver consistent, seamless experiences across a variety of media, boosting customer satisfaction and loyalty.
2- Micro Segmetation
According to Deloitte research, businesses using micro-segmentation techniques saw an average 19% boost in revenue and a 15% drop in marketing expenses. As part of conventional customer segmentation, customers are frequently split into large categories. Customer segmentation is moving towards micro-segmentation, which categorizes clients into far more specific groups in the future. By employing micro-segmentation to identify the unique preferences and behaviors of each individual customer, businesses can provide very personalized experiences and services.
3- Forecasting Segmentation
According to a study by Forrester, companies that effectively utilize predictive segmentation experience a 35% increase in customer engagement rates and a 25% increase in marketing-generated revenue. In order to predict future client preferences and behaviors, predictive segmentation makes use of machine learning techniques. The algorithms can analyze past consumer data to find patterns and trends that may be used by firms to forecast future customer behavior. This foresight enables businesses to proactively modify their marketing tactics and services to match client requirements before they express them, improving customer happiness and increasing conversion rates.
How to choose the best AI solution for your data project?
In this white paper, we provide an overview of AI solutions on the market. We give you concrete guidelines to choose the solution that reinforces the collaboration between your teams.
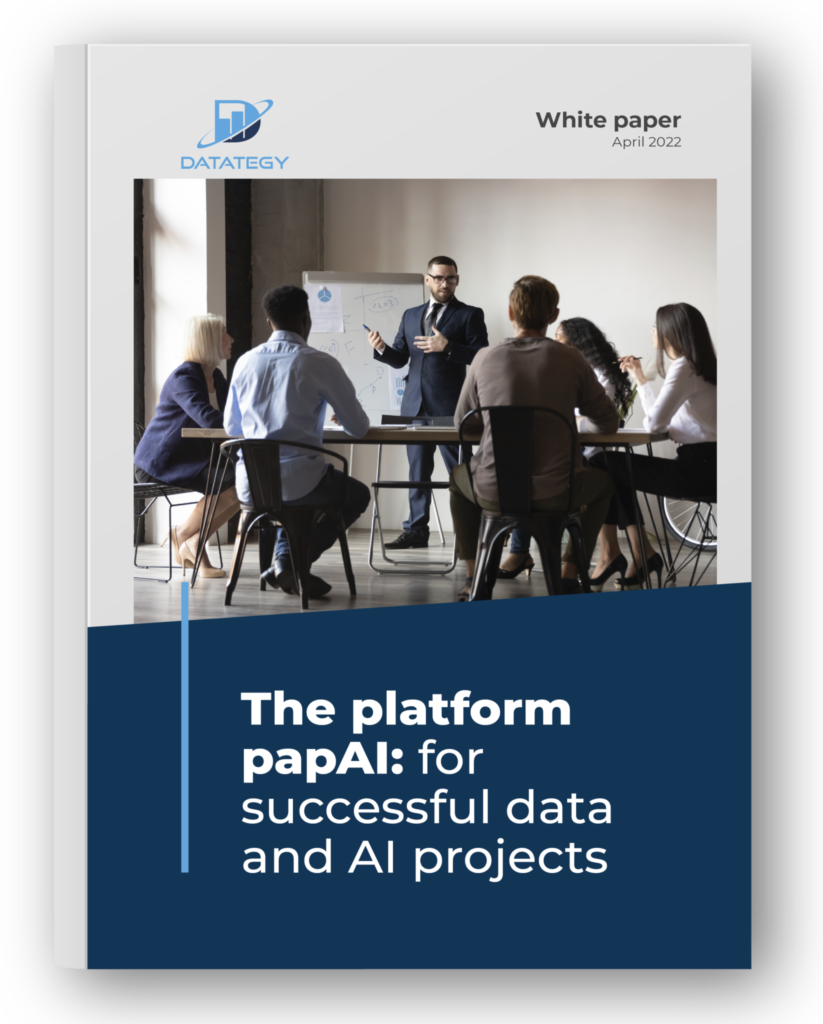
Case Study:
How Does PapAI Solution Help you Succeed in Personalized Marketing with Efficient Customer Segmentation?
An excellent tool that helps companies and organizations manage and comprehend their client interactions is a customer relationship management (CRM) system.
With the development of the Internet, CRM systems—originally designed for large corporations—have become more widely available to small company owners. These technologies make it easier to gather and arrange client data in a centralized CRM database, opening the door to more complex analytical methods like customer segmentation and thorough contact histories.
1- Data Importation
Thanks to the variety of data sources available, you have the flexibility to import data from virtually anywhere into your papAI project for analysis and visualization. Whether it’s from your local machine, an external database (SQL or NoSQL), cloud storage, or an API, papAI makes it easy to bring in data for analysis. Additionally, you can even create a completely new dataset using the specialized Python or SQL recipe editor.
To get started with importing your data, you can use the tools provided in the papAI interface. For our specific use case, we’ll be importing our dataset from our local machine using the appropriate tool. You can access this tool by clicking the plus button located in the top right corner of the interface or by using the Import dataset button in the Flow interface.
Once you’ve selected the local import option, a new interface will appear that allows you to easily import any tabular file in CSV or XLSX format. You can import your desired files either by clicking the Import button or by using the drag-and-drop feature. Once your data has been imported, you can preview a subset of the data to verify that it was imported correctly. After ensuring that everything is in order, you can simply select the Import button to start the uploading process. A progress bar will keep you informed of the status of the upload, and when it’s complete, your dataset will be ready for use in your project’s flow.
2- Data Analysis and Preparation
a- Cohort Analysis
Once you’ve imported your dataset into papAI, you can begin exploring its content and obtaining an initial analysis to determine the cleaning steps necessary to extract the most valuable insights from your data. Cohort analysis involves segregating data within a dataset into comparable groups for analysis. These groups, known as cohorts, typically share similar qualities or experiences over a specific period.
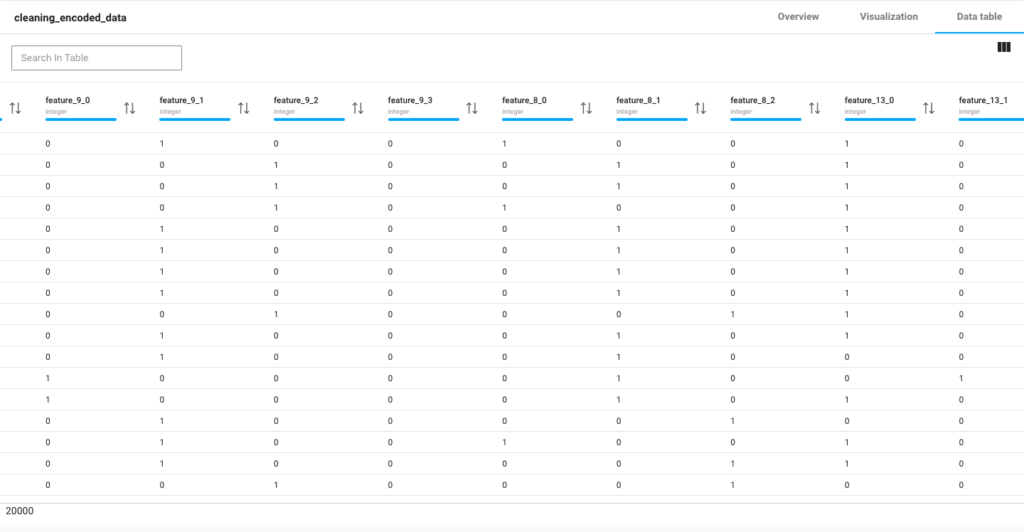
Dataset visualization
The dataset consists of several variables that provide detailed information about each transaction. The InvoiceNo variable represents a unique identifier for each transaction, distinguishing invoices from aborted operations indicated by the prefix C. The StockCode variable corresponds to a specific product code, uniquely identifying each item in the company’s product catalog. The Description variable contains the name or description of the product. The Quantity variable indicates the number of products sold for each invoice, reflecting the quantity purchased. The InvoiceDate variable records the date and time of each invoice. The UnitPrice variable denotes the price of each product in British Pounds (GBP). The CustomerID variable represents a unique customer identification number. Lastly, the Country variable specifies the country where the respective customer resides. These variables collectively provide comprehensive details about the transactions, products, customers, and their locations.
In our tutorial we will need to add a new column total price that indicates the quantity multiplied by the unit price and to do so, our platform offers some basic operations as you can see in the following video.
Examining a customer’s or user’s behavior throughout their lifecycle can unveil significant trends. By dividing customers into smaller groups, patterns throughout each individual’s journey can be observed more effectively. This approach contrasts with analyzing all clients uniformly, disregarding the natural cycle that a client undergoes.
We can also divide the customers based on the product description and filter them by descending order of bought products.
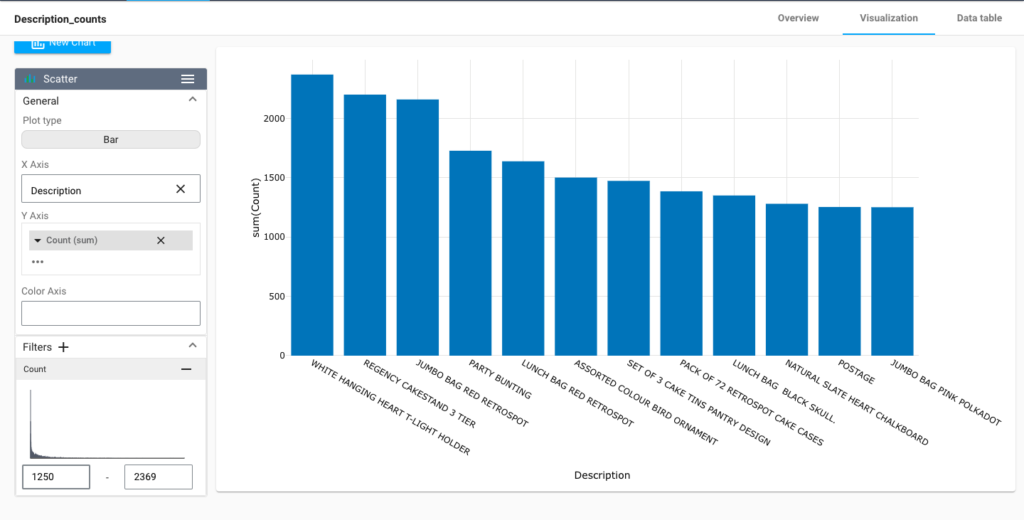
Segmentation based on product description
By visualizing the data, we can gain insights into the underlying patterns and trends that might not be immediately apparent from just looking at the raw data. This can help us to identify potential issues or opportunities to improve the quality of our data.
b- Customer Segmentation With RFM
Customer segmentation with RFM (Recency, Frequency, Monetary) analysis is a powerful technique used to categorize customers based on their transactional behavior. RFM analysis considers three key factors: recency, which measures how recently a customer made a purchase; frequency, which measures how often a customer makes purchases; and monetary, which measures the total monetary value of a customer’s purchases. By analyzing these three dimensions, customers can be segmented into distinct groups that share similar characteristics and behaviors.
To calculate the RFM score we have to create a Python recipe, to do so just click on the dataset you want to calculate the RFM on then in the left menu you click on Python, and a new script will be created in which you fill in your script.
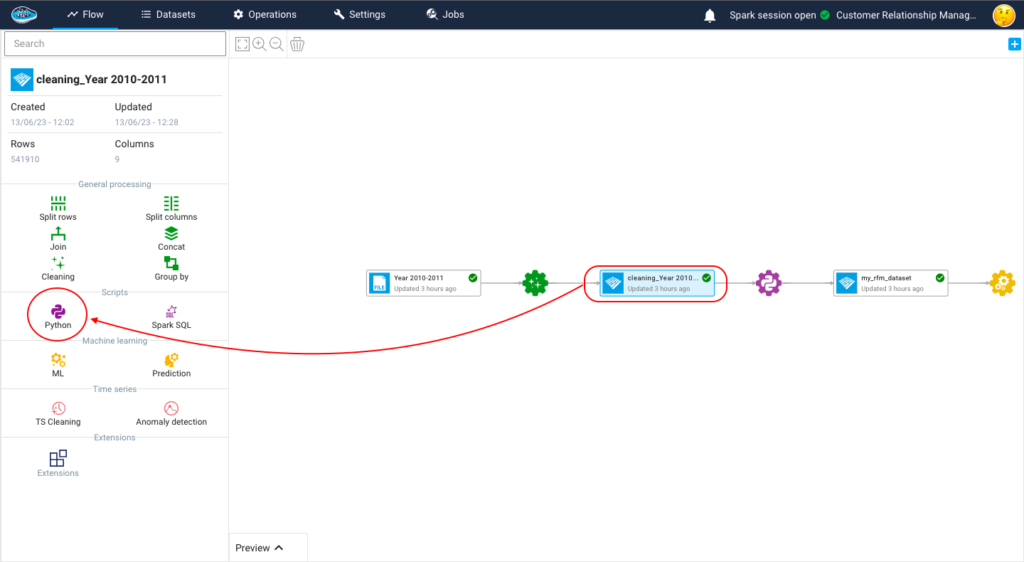
Python recipe creation
The code begins by importing a dataset. This dataset likely contains information about customer transactions, including the customer ID, invoice date, invoice details, and total price. The InvoiceDate column is then converted to DateTime format to enable further calculations based on dates. The maximum date in the InvoiceDate column is also determined.
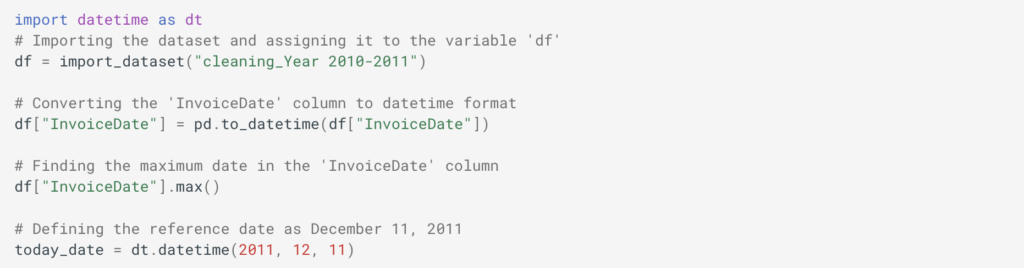
RFM values are calculated for each customer by grouping the dataset based on the Customer_ID column and performing aggregations. The following RFM metrics are calculated:
Recency: It represents the number of days between the latest invoice date and a reference date (December 11, 2011 in this case).
Frequency: It represents the number of unique invoices for each customer.
Monetary: It represents the sum of total prices for each customer. To ensure meaningful segmentation, customers with zero monetary value (i.e., no purchases) are filtered out.
To ensure meaningful segmentation, customers with zero monetary value (i.e., no purchases) are filtered out.
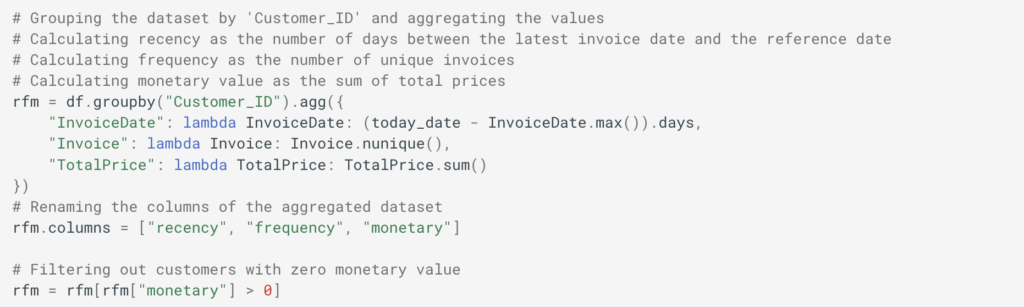
RFM scores are assigned to each customer based on their recency, frequency, and monetary values. The scores are divided into quintiles (5 bins) to create a relative ranking. The highest score indicates the best value for a particular RFM metric. The following steps are performed for scoring:
Recency Score: The recency values are divided into 5 equal-sized bins, and labels from 5 to 1 are assigned, with 5 being the most recent purchases.
Frequency Score: The frequency values are ranked and divided into 5 equal-sized bins, and labels from 1 to 5 are assigned, with 1 indicating the lowest frequency.
Monetary Score: The monetary values are divided into 5 equal-sized bins, and labels from 1 to 5 are assigned, with 1 indicating the lowest monetary value.
The RFM scores for each customer are combined to create an overall RFM score. For example, if a customer has a recency score of 4 and a frequency score of 3, their RFM score will be ’43’
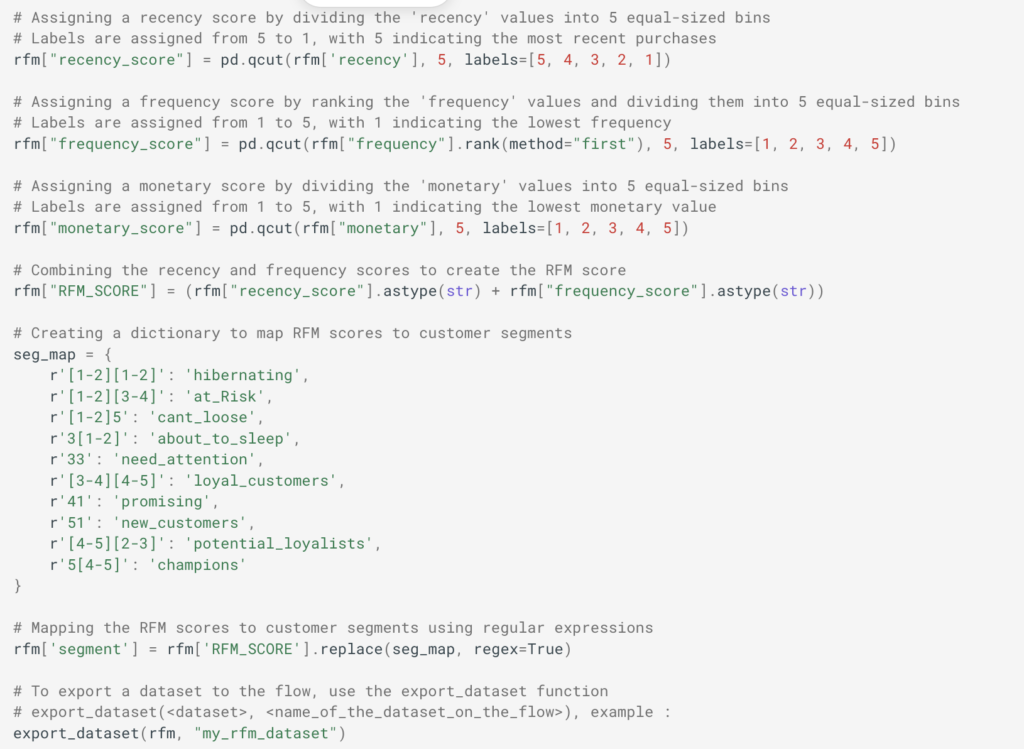
Recency score reflects the idea that customers who have made more recent purchases are likely to be more engaged and responsive to marketing efforts. Frequency score highlights the loyalty and engagement level of a customer, as frequent purchasers often represent the most valuable customers. Monetary score value provides insights into the spending power and profitability of a customer, as customers who have spent more are typically more valuable to the business.
c- Clustering
Your dataset is prepared at this stage to be used for training and testing various models before selecting the best one to be utilized in production. By selecting the training dataset and then the ML Lab icon, we can start the machine learning process. You’ll get access to the ML Lab, where you may test various models. However, you must first identify the use case you wish to address. Since the New use case button must be clicked, creating an ML use case is quite easy. You may select the kind of use case needed to respond to it through a pop-up; in our example, it’s a Clustering problem.
When accessing your use case, you are able to create and build your own ML pipeline easily through the ML Lab. The ML Lab gives you the ability to create a pipeline from scratch with multiple models and parameters to optimize the process and extract the best model without any code. To begin the process, you need to select Create Prototypes and a new interface will appear with the first step which is the feature selection. Through this step, you select the features to be taken into account in the model training and also apply some preprocessing to ensure better results. Following the feature selection comes the model selection where we are going to simply select the regular ML models such as Mean Shift or K-Means with their default parameters To add them, simply toggle the button next to the model to activate it.
3- Model evaluation
In the papAI AutoML module, we have several metrics to evaluate our models. The Davies-Bouldin Index measures the quality of clustering by computing the average similarity between each cluster and its most similar cluster while considering the average dissimilarity between each cluster and the least similar cluster. The index ranges from 0 to infinity, where a lower value indicates better clustering. A value closer to 0 indicates tight and well-separated clusters.
The Silhouette Coefficient assesses the quality of clustering by evaluating how well each data point fits within its assigned cluster compared to other clusters. It computes the average silhouette coefficient for all data points, ranging from -1 to 1. A coefficient close to 1 indicates that the data point is well-matched to its own cluster and poorly matched to neighboring clusters, indicating good clustering. Negative values indicate that data points might be assigned to the wrong clusters.
The Calinski-Harabasz Index, also known as the Variance Ratio Criterion, is a measure of cluster separation and compactness. It calculates the ratio of between-cluster dispersion to within-cluster dispersion. A higher index value indicates better-defined and more separated clusters. It is often used to determine the optimal number of clusters by comparing index values across different cluster solutions. In our case, we focus mainly on the Silhouette Coefficient.
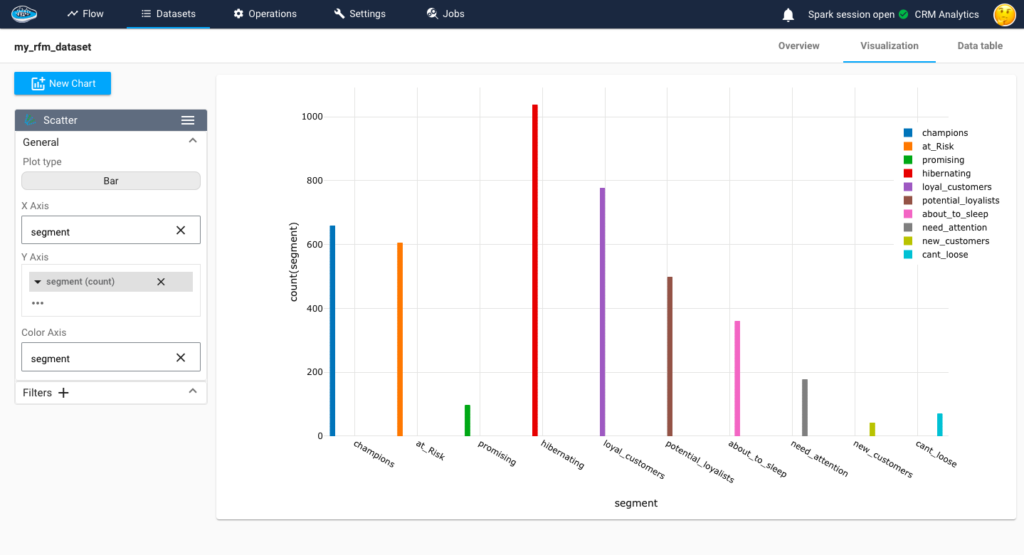
Segmentation of customers through the clustering model
Improve Customer Segmentation by Building your Own AI-based Tool with papAI solution
You have the ability to completely transform your customer segmentation strategy with papAI solution. With the help of our platform, you may create an AI-driven toolset that is specifically suited to meet your segmentation requirements. You may improve the accuracy and effectiveness of your segmentation efforts by using cutting-edge machine-learning approaches.
Book your personalized demo today to witness firsthand how papAI solution can transform your customer segmentation landscape. Our team of experts can help you create a custom AI-based tool that meets the unique needs of your organization.
Interested in discovering papAI?
Our commercial team is at your disposal for any questions
Why AIOps Is Key to Cyber Threat Detection in Defense?
Why AIOps Is Key to Cyber Threat Detection in Defense?...
Read MoreHow AI Transforms Predictive Maintenance in Defense Equipment
How AI Transforms Predictive Maintenance in Defense Equipment In a...
Read MoreHow to Scale AI Without Breaking Your Infrastructure in 2025
How to Scale AI Without Breaking Your Infrastructure in 2025...
Read More