Understanding Multimodal AI Complexity Businesses commonly use the term “multimodal”...
Read More Streamlining Banking Processes:
Automated Loan Approvals Using AI
Table of Contents
ToggleThe Banking sector always struggles to streamline operations to effectively satisfy client needs in today’s fast-paced environment. Loan approvals are one area that has attracted a lot of attention. Traditional loan approval procedures sometimes require laborious manual operations, which causes delays and client unhappiness. However, due to the advancement of artificial intelligence (AI), bank firms can now speed up, improve accuracy, and personalize their loan approval procedures.
Find out how papAI can improve the deployment of AI projects in the Banking Sector here.
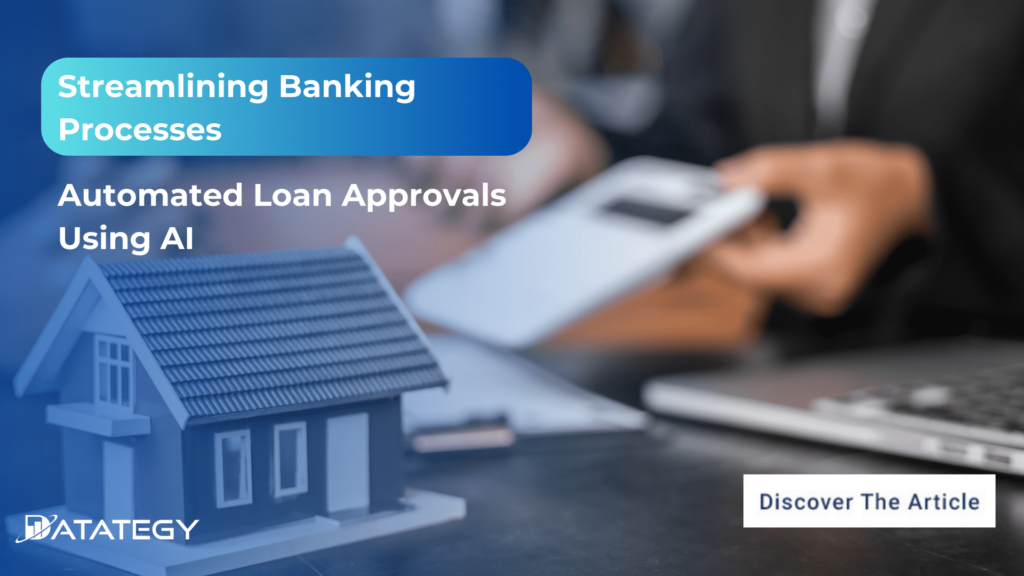
In this article, we’ll look at how papAI solution can help to automate loan approvals for better decision-making.
State of AI adopting in banking industry
The level of artificial intelligence (AI) maturity in the banking industry differs greatly among various organizations. While some banks are only now beginning to investigate AI’s possibilities, some have completely embraced it and are deploying it in a variety of sectors.
The amount of money invested in the technology is one of the primary criteria that influence the maturity of AI in a bank. Banks that have made significant investments in AI are probably using the technology more effectively.
To enhance customer service, these banks may have introduced AI-powered chatbots and virtual assistants. They may also be utilizing machine learning algorithms to analyze financial data and identify and stop fraud. On the other hand, the “Accenture levels of AI maturity by industry ranking” reveals that banks are far from behind industry leaders in their AI maturity.
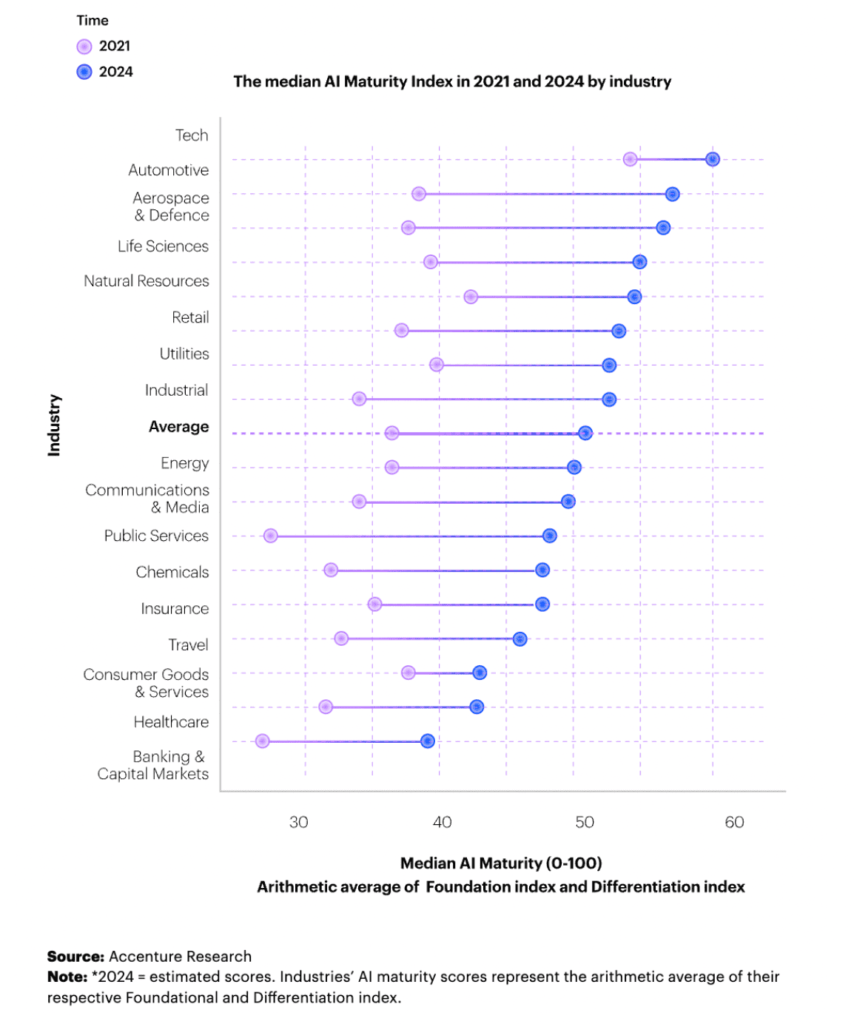
The creation and use of AI solutions in banking organizations is far less advanced. Only 1% of financial organizations, according to Accenture, are AI Achievers. The fact that 75% of financial institutions are still in the early experimental stage of AI research is even more worrisome. This is significant because Achievers, Builders, and Innovators frequently invest more time, skill, and technology into implementing AI ambitions and transforming their organizations.
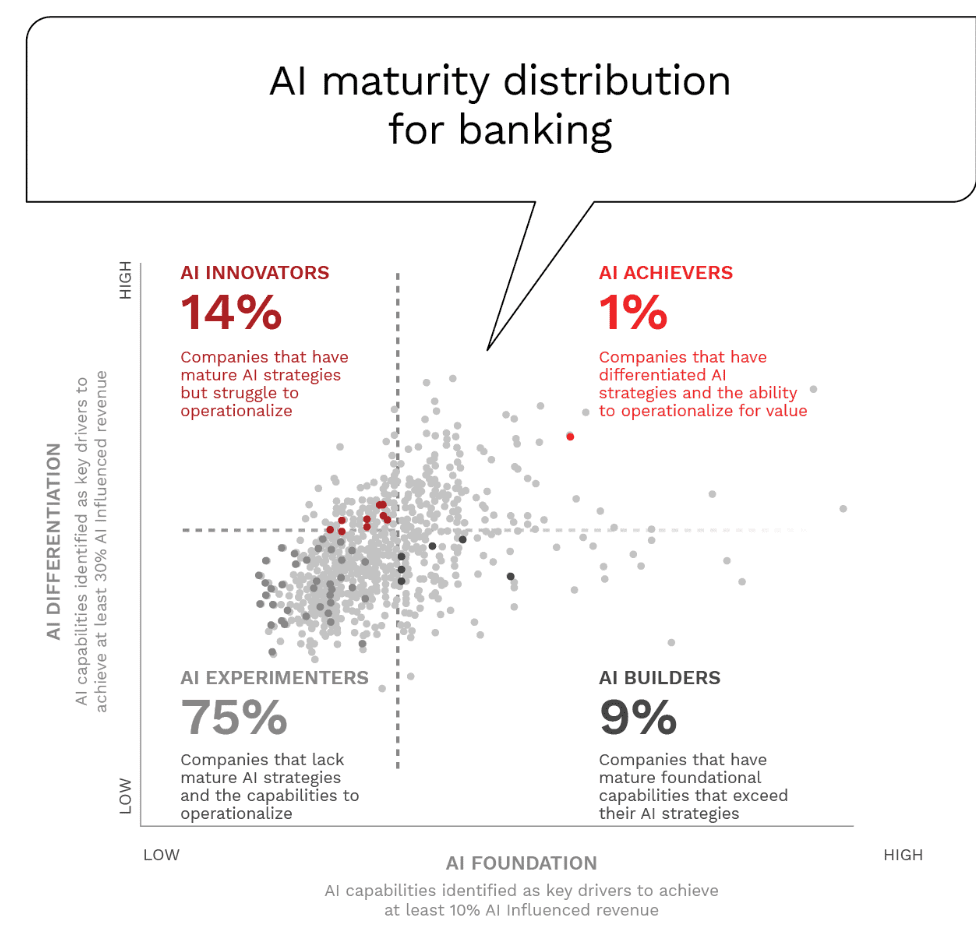
The Loan Approval Process: An Overview
The process through which a financial institution evaluates a borrower’s creditworthiness and determines whether to approve the requested loan is known as loan approval. In the past, this procedure required a significant quantity of documentation and manual examination, which resulted in lengthy waiting times for candidates. The desire for rapid and easy financial services is driving the need to streamline loan approvals. While banks work to reduce operating costs and the risks involved with manual processing, customers want quicker choices and frictionless experiences.
How AI Revolutionizes Loan Approvals
1- Data Collection and Analysis
Data collection has been transformed with the advent of AI. Systems that use artificial intelligence (AI) are capable of automatically retrieving and analyzing enormous volumes of data from a variety of sources, including digital records, governmental databases, financial institutions, and even social media. These automated data-gathering techniques not only quicken the procedure but also guarantee the precision and thoroughness of the data gathered.
AI is able to extract crucial information and pertinent patterns from unstructured data, such as text and photos, allowing for a more thorough evaluation of a candidate’s financial status. The applicant’s creditworthiness is seen holistically thanks to this in-depth analysis, which was not possible with manual data collection.
2- Risk Assessment and Credit Scoring
An essential step in the loan process is risk assessment. In the past, loan officers assessed the credit risk of applicants using past data and their own judgment. However, banks may now make data-driven choices, leading to more accurate assessments, thanks to AI-driven risk assessment.
Huge volumes of data are used by AI algorithms to find patterns and trends that people might miss. AI algorithms can determine how likely a borrower is to fail on a loan by looking at their credit history, debt-to-income ratio, payment behavior, employment stability, and other pertinent indicators. By minimizing the likelihood of lending to high-risk borrowers, this thorough review also lowers the possibility of bank losses.
On the other hand, AI-driven credit scoring systems use a wider variety of data sources, such as unconventional ones like utility bill payments, social media usage, and even educational history. The creditworthiness of those with little credit history or those who were previously disqualified from the standard credit scoring system may now be evaluated by AI algorithms thanks to alternative data.
3- Enhance Fraud Detection
A new age of fraud detection has been ushered in by AI-powered loan approval systems that can analyze enormous volumes of data using cutting-edge algorithms and machine learning skills. Contrary to rule-based systems, AI is capable of spotting intricate patterns and minute irregularities that may point to fraudulent activity, even when they are skillfully concealed.
The capacity of AI to do behavioral analysis is one of the technology’s main advantages in the fraud detection space. AI algorithms develop unique profiles for each application by analyzing past data and consumer behavior. These profiles are built using a variety of information, such as financial background, spending trends, transaction patterns, and internet activity.
The AI system assesses a new loan application by contrasting the behavior of the applicant with their predetermined profile. The system sends out a warning for additional research if there are any deviations or contradictions. For instance, AI can identify some actions as possibly fraudulent if an applicant’s spending habits abruptly change or if several loan applications are submitted quickly from various places.
The Advantages of Automatic Loan Approval
Lower Operating Costs:
In the past, manual assessments required a sizable workforce, which accounted for a sizable amount of operating costs associated with traditional loan approval procedures. Longer approval delays and higher operational costs for financial institutions were the results of loan officers, administrative employees, and support staff spending a lot of time assessing and processing loan applications. Research by Accenture claims that by 2025, AI-based automation in the financial services industry would result in cost savings of up to 30%. Savings on operating costs, risk management, and compliance are all included.
Improved Decision-Making:
By reducing the dependence on human judgment and prejudice, the data-driven method ensures fair and consistent evaluations for all loan applicants. AI can precisely forecast credit risk and the possibility of loan payback because of its capacity to spot patterns and trends in prior data. By reducing the likelihood of issuing high-risk loans, this predictive skill also shields banks from future financial losses. Additionally, AI’s real-time flexibility enables it to keep up with evolving market conditions and client behaviors, which makes lending choices more proactive and responsive.
Enhance Customer Experience:
According to a study by the Harvard Business Review, AI can reduce loan approval times by up to 90%. Because AI-driven judgments are made quickly and effectively, applicants don’t have to worry about waiting around for lengthy periods of time to hear back about their loan applications. Customers may apply for loans more easily because of the smooth and user-friendly application process made possible by AI interfaces and chatbots, which also reduces paperwork and streamlines the procedure. With the use of AI’s data-driven insights, banks may provide individualized loan choices that are catered to customers’ credit profiles and financial demands, making the loan products more attractive and relevant.
Case study:
Innovative Credit Scoring with papAI: A Comprehensive Guide
1- Data Presentation
In the world of finance, understanding the eligibility of individuals for credit loans is of paramount importance. Lending institutions rely on comprehensive datasets to assess the creditworthiness of potential borrowers, make informed lending decisions, and manage risk effectively. The dataset used in our experiment encompassing 45 distinct columns, is a valuable resource in this pursuit.
This dataset captures a wide range of attributes related to loan applicants, loan details, financial history, and other pertinent information. Each column in this dataset plays a crucial role in assessing the eligibility of individuals for credit loans. It provides financial institutions, data scientists, and analysts with the necessary insights to make informed decisions regarding loan approvals, interest rates, and repayment expectations.
The dataset covers essential information such as Member ID, loan amount, interest rates, employment details, home ownership, annual income, and payment history. Additionally, it includes more intricate features such as delinquency history, credit utilization, and months since the last derogatory event. All of these factors contribute to a holistic view of an applicant’s creditworthiness.
Understanding and analyzing this dataset can lead to valuable insights for risk assessment, marketing strategies, and the development of more accurate predictive models. Financial institutions can use this dataset to refine their lending criteria and enhance their ability to extend credit to deserving individuals while managing risks effectively.
As we delve deeper into this dataset, we will explore each column’s significance and its potential impact on loan eligibility and lending practices. By leveraging the insights gleaned from this dataset, financial institutions can make more informed and responsible decisions, ultimately benefiting both borrowers and lenders.
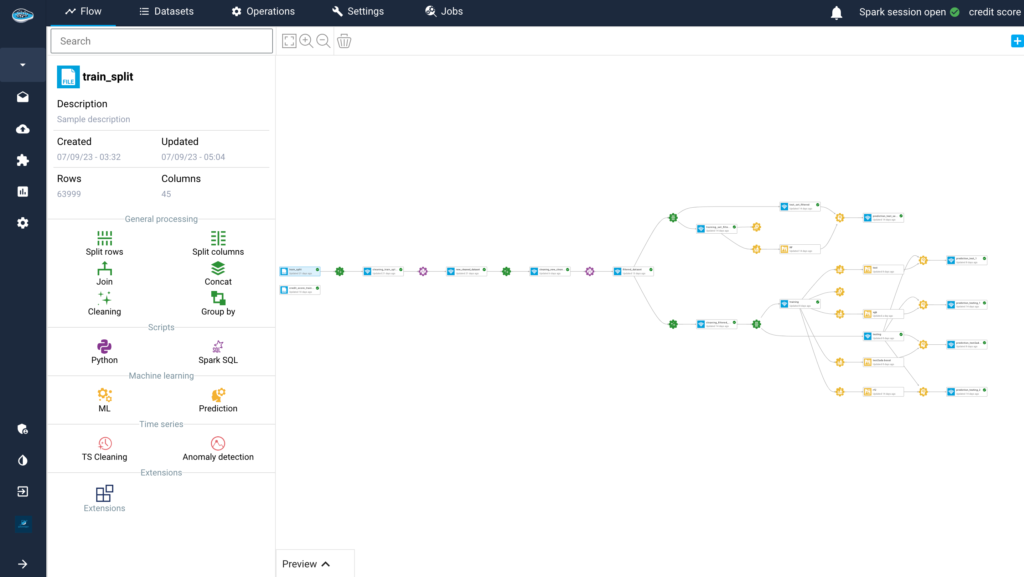
2- Data Analysis and Preparation
In the journey toward better loan eligibility prediction, meticulous data analysis and preparation serve as the foundation for informed decision-making. Our process begins with a comprehensive exploration of numeric columns, employing the powerful `df.corr()` method to unravel the intricate relationships within the data. One standout metric we focus on is `revol_util`, which signifies borrowers’ revolving utilization rates—a pivotal factor in assessing creditworthiness.
As we delve deeper, we find that our dataset adheres to a bell curve distribution, albeit adorned with some pesky outliers. Our strategy for these outliers is to impute the median, offering robust resistance to their skewing influence.
Next, we scrutinize the ‘Total Collected Amount’ (`tot_coll_amt`) column, which, burdened by a bias toward zero values, is deemed inconsequential. Therefore, we promptly remove it from our analysis. A similar situation arises with the ‘Total Current Balance’ (`tot_cur_bal`) column, replete with outliers. Our solution is to replace them with the median, ensuring a more reliable dataset.
In the realm of categorical columns, we are vigilant about eliminating the irrelevant and superfluous. ‘batch_enrolled,’ ‘desc,’ ‘zip_code,’ and ‘addr_state’ fall victim to this scrutiny. They offer little to no insight into loan eligibility and thus find their way out of our analysis.
We take a pragmatic approach to employment information—substituting ‘Unknown’ for missing values in ’emp_title’ and converting ’emp_length’ into numeric values for consistency.
The ‘term’ column, often laden with the ‘months’ tag, undergoes a simplifying transformation as we strip away this excess, rendering it more amenable to analysis.
Lastly, ‘last_week_pay,’ when distilled down to its numeric essence, provides us with valuable insights, further enriching our dataset. By meticulously executing these steps, we sculpt our raw data into a refined, insightful, and actionable dataset. This preparatory phase sets the stage for constructing accurate predictive models that will empower lenders to make prudent loan eligibility decisions.
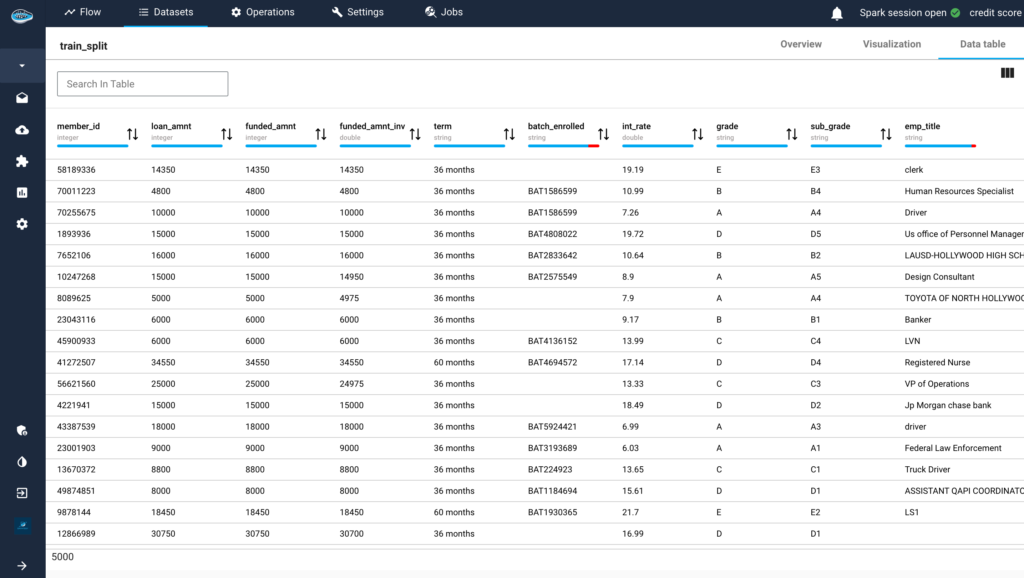
Next, we split the dataset into training and testing sets. We use a stratified splitting method that helps prevent biases that may arise from uneven distribution, ensuring that both sets have representative samples. Specifically, we allocate 80% of the data for training and the remaining 20% for testing.
3- Model Training
papAI’s Machine Learning Lab offers a wide range of pre-built machine learning algorithms and model selection tools. Choosing the right algorithm is essential for accurate credit scoring. You can experiment with various algorithms such as Logistic Regression, Random Forest, Gradient Boosting, and Neural Networks.
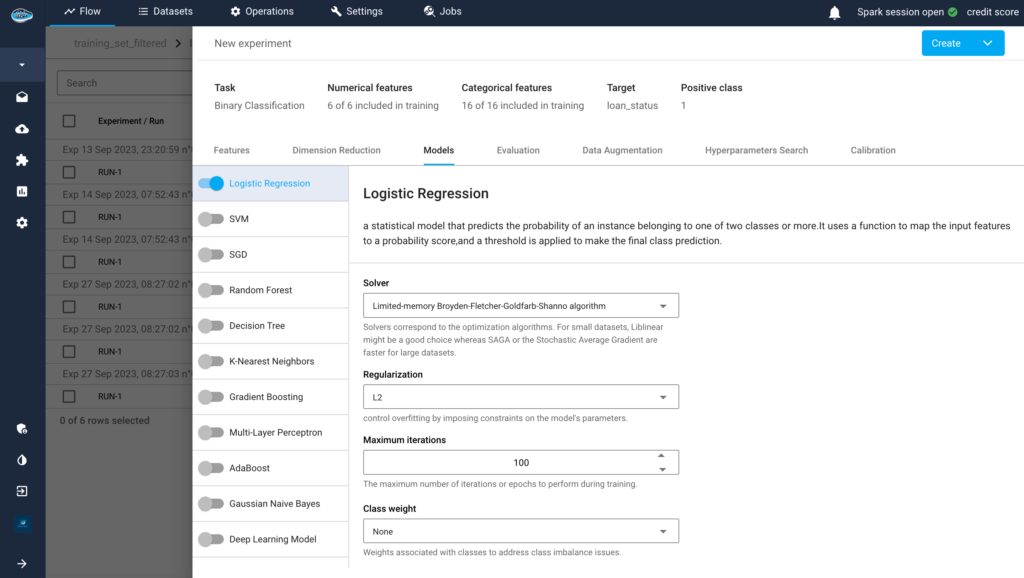
papAI provides a user-friendly interface to compare the performance of these models using metrics like accuracy, precision, recall, and ROC curves. This iterative process ensures you select the best model. Once you’ve chosen a model, papAI simplifies the training process. You can specify hyperparameters and train the model with just a few clicks. The platform also supports automated hyperparameter tuning to fine-tune your model for optimal performance.
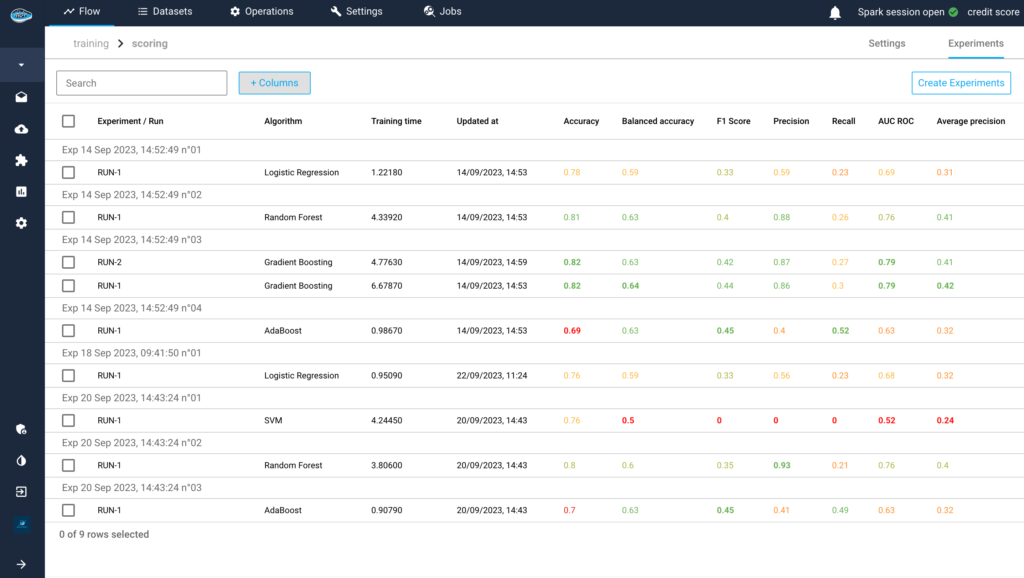
After training your credit scoring model, it’s essential to evaluate its performance rigorously. papAI’s Machine Learning Lab provides detailed model evaluation reports, including confusion matrices, precision-recall curves, and feature importance scores. These insights help you assess the model’s effectiveness in identifying creditworthy individuals.
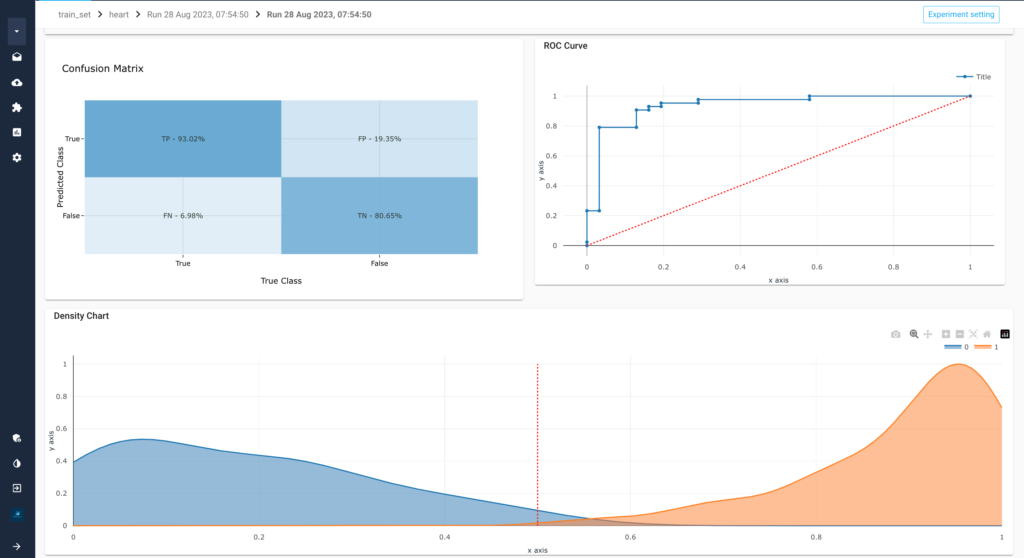
4- Explainability and Interpretability
In the quest for transparency and fairness in credit scoring models, understanding how specific features influence credit decisions and providing counterfactual explanations is important. papAI’s Machine Learning Lab offers valuable tools for achieving this level of interpretability, allowing financial institutions to build trust with both regulators and customers.
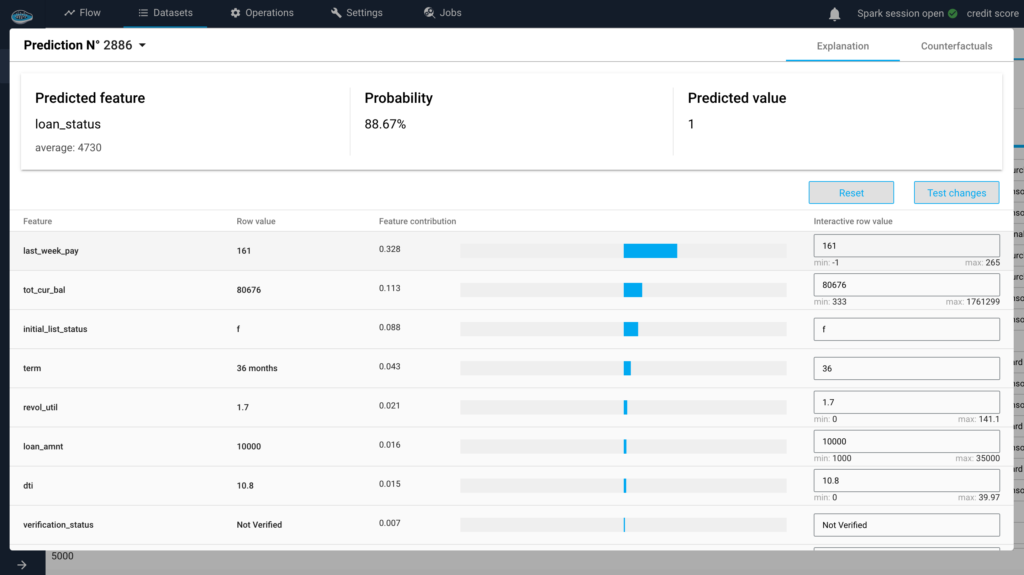
- Credit scoring models often consider a multitude of features when making lending decisions. It’s vital to know which features have the most significant impact on these decisions. papAI’s platform provides feature importance scores, which help answer questions like:
- Which Features Matter Most: By ranking features based on their influence on the model’s predictions, you can identify the key drivers of creditworthiness.
- Direction of Impact: Feature importance scores can reveal whether an increase in a particular feature, such as income or credit utilization, is positively or negatively correlated with creditworthiness.
Understanding feature impact empowers financial institutions to refine lending policies and communicate more effectively with customers. For instance, if credit history is found to be the most influential factor, institutions can emphasize the importance of maintaining a positive credit record to potential borrowers.
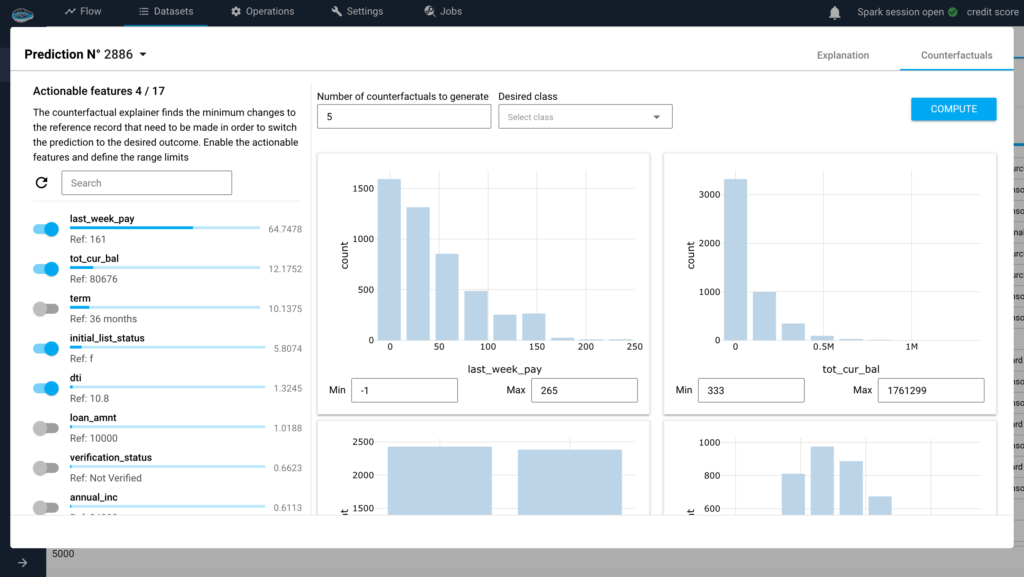
Counterfactual explanations provide actionable insights into how individuals can change their financial behavior to improve their creditworthiness. papAI’s Machine Learning Lab supports the generation of counterfactual explanations for specific cases, helping borrowers understand what steps they can take to obtain better credit terms. Key aspects of counterfactual explanations include:
- “What-If” Scenarios: By altering feature values while keeping other factors constant, papAI’s platform can generate “what-if” scenarios. For example, it can show how an applicant’s credit score would change if they reduced their credit card balances.
- Customized Advice: Counterfactuals offer personalized advice to borrowers. For instance, an applicant might receive recommendations like “Paying off your outstanding debt by 20% could improve your creditworthiness.”
- Empowering Borrowers: Providing actionable steps for improvement not only fosters customer trust but also encourages responsible financial behavior.
By offering feature impact explanations and actionable counterfactuals, financial institutions using papAI’s Machine Learning Lab can enhance transparency, promote responsible lending, and ensure that credit scoring practices are not only accurate but also customer-centric.
Build Your Own AI-based Tool using papAI Solution to Automate your Loan Approvals
You may utilize AI to make your company’s AI initiatives more comprehensible with the help of papAI solution. Using papAI, you have the option to create your own AI-based tool that is tailored to your specific needs. Modern machine learning methods will significantly improve the accuracy and efficiency of your prediction processes.
Through a process of data mining, cleansing, and visualization that can hasten the deployment of AI projects, papAI solution aids in explaining and making transparent the outcomes. Reserve your demo today. Our team of specialists can assist you in developing a special AI-based product that is tailored to the requirements of your company.
Interested in discovering papAI?
Our commercial team is at your disposal for any questions
How Do AI Predictive Models Estimate Car Prices?
How Do AI Predictive Models Estimate Car Prices? Valuation methods...
Read More“DATATEGY EARLY CAREERS PROGRAM” With Tommy GUYOT
“DATATEGY EARLY CAREERS PROGRAM” With Tommy GUYOT Hello! My name...
Read More