Applying NLP for Intelligent Document Analysis using papAI 7 AI...
Read MoreAI Origins: Yann LeCun
Table of Contents
ToggleWelcome to ”AI Origins “ series. In this collection of articles, we take you on an inspiring journey through the history of artificial intelligence, focusing on the remarkable individuals who have played a crucial role in making AI accessible to everyone.
Throughout these articles, we’ll dive into the life stories and contributions of these innovative minds who have reshaped the AI landscape, paving the way for a more inclusive and transformative future. Join us as we celebrate the human ingenuity behind the AI revolution and explore the extraordinary individuals who have made it all possible.
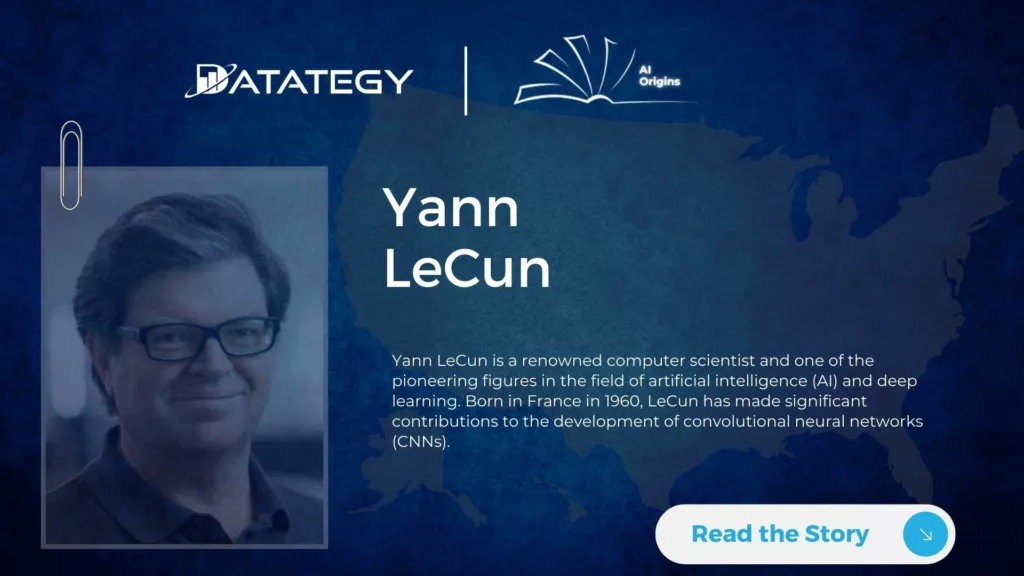
Yann LeCun stands as a leading figure in the field of artificial intelligence (AI), renowned for his pioneering contributions to deep learning and computer vision. Born in Soisy-sous-Montmorency, France, his innovative work has played a pivotal role in advancing the capabilities of AI technology. In 2018, LeCun’s outstanding achievements were recognized with the prestigious Turing Award, a testament to his profound impact on the field of computer science.
With a wealth of experience and expertise in AI research, he continues to spearhead groundbreaking initiatives, shaping the trajectory of AI and machine learning. LeCun’s dedication to advancing the frontiers of AI has established him as a prominent influencer, driving innovation and transformative change in the field.
Key Dates about Yann LeCun
Yann LeCun Timeline
Date | Event |
---|---|
1960 | Yann LeCun is born in Soisy-sous-Montmorency, France. |
1987 | Received his Ph.D. in Computer Science from Université Pierre et Marie Curie in France |
1987 | Publishes the paper on Convolutional Neural Networks (CNNs), which becomes a foundational work in computer vision. |
1990 | Develops the LeNet-5 architecture, a pioneering CNN model for handwritten digit recognition, which laid the groundwork for modern deep learning. |
1996 | Appointed as the Head of the Image Processing Research Group at AT&T Labs, where he continued to advance CNN research. |
2003 | Becomes a Professor at New York University, where he establishes the NYU Center for Data Science and continues his research in AI. |
2006 | Appointed as the Director of AI Research at Facebook, leading efforts to advance AI technologies and applications. |
2013 | Awarded the IEEE Neural Network Pioneer Award for his contributions to the development and application of neural networks. |
2018 | Receives the Turing Award, along with Geoffrey Hinton and Yoshua Bengio, for their groundbreaking work in deep learning. |
Present | Continues to be a prominent figure in AI research and education. |
A Landmark Innovation in Computer Vision
Yann LeCun’s LeNet-5 represents a pivotal moment in artificial intelligence, particularly in computer vision. Developed in the early 1990s, LeNet-5 introduced Convolutional Neural Networks (CNNs) and revolutionized how machines recognize handwritten digits. Its innovative architecture automatically learns hierarchical features from raw pixel data, eliminating manual feature extraction.
LeNet-5’s translation invariance and pooling layers ensure robustness and computational efficiency. This landmark model laid the foundation for modern CNNs, impacting diverse applications in image recognition, from object detection to medical imaging. LeNet-5 remains a cornerstone in unsupervised learning and AI advancement.
Benefits of LeNet-5
Benefit | Description |
---|---|
Handwritten Digit Recognition | LeNet-5 was designed specifically for handwritten digit recognition tasks, making it highly effective for tasks such as reading zip codes, postal codes, and bank checks. |
Convolutional Neural Network | LeNet-5 introduced the concept of Convolutional Neural Networks (CNNs), a type of neural network particularly suited for image recognition tasks due to its hierarchical structure. |
Parameter Efficiency | LeNet-5 achieved remarkable parameter efficiency, meaning it required relatively few parameters compared to traditional neural network architectures, making it computationally efficient. |
Feature Hierarchies | LeNet-5 utilized a hierarchical structure of feature extractors, where lower layers captured simple features like edges and corners, while higher layers captured more complex features like shapes and patterns. |
Translation Invariance | LeNet-5 demonstrated strong translation invariance, meaning it could recognize patterns in an image regardless of their position, making it robust to variations in object location within the image. |
Pooling Layers | LeNet-5 incorporated pooling layers, which helped reduce the spatial dimensions of the feature maps while retaining important information, leading to improved computational efficiency and generalization. |
Interested in discovering papAI?
Our AI expert team is at your disposal for any questions
“DATATEGY EARLY CAREERS PROGRAM” With Samar CHERNI
“DATATEGY EARLY CAREERS PROGRAM” With Samar CHERNI Hello, my name...
Read MoreAI Origins: Yann LeCun
AI Origins: Yann LeCun Welcome to ”AI Origins “ series....
Read MoreThe Rise of Humanoid Robots: Progress and Challenges
The Rise of Humanoid Robots: Progress and Challenges Some humanoid...
Read More