Understanding Multimodal AI Complexity Businesses commonly use the term “multimodal”...
Read MoreHow AI is Reshaping Sentiment Analysis?
Table of Contents
ToggleIn the last decade, the rise of social networks and mobile apps flooded us with a wealth of texts, images, and videos. Recognizing the value of this trove, businesses have embraced the challenge of analyzing it to glean deeper insights into their customers.
At the forefront of customer understanding lies sentiment analysis, a cornerstone for extracting actionable insights. Here, AI technologies play a pivotal role in crafting these analytical tools, shaping the future of customer-centric strategies.
For example, Artificial neural networks achieve 85% accuracy in identifying the sentiment, the highest accuracy level among other methods.
Find out how papAI can improve the deployment of AI projects in Sales & Marketing.
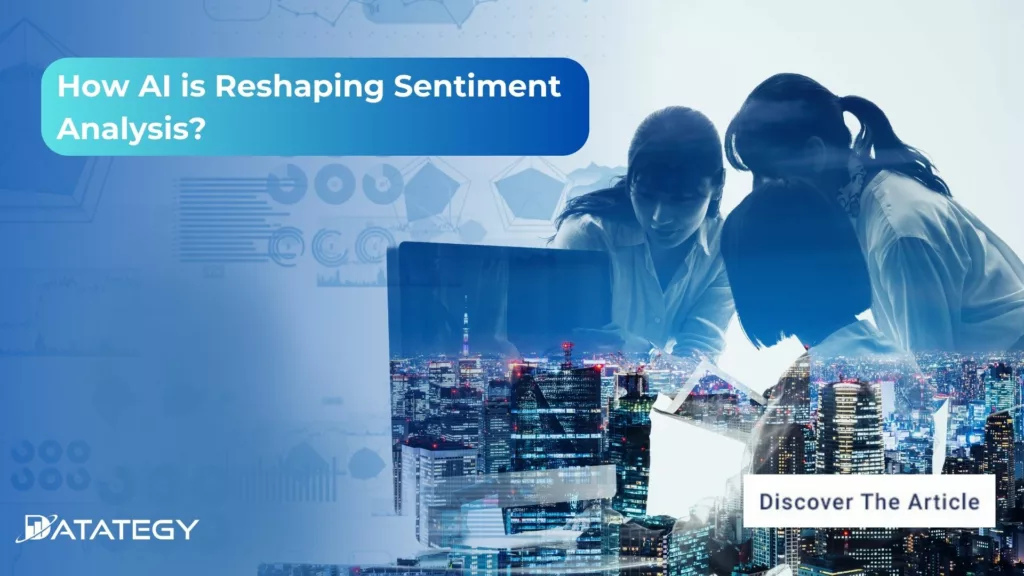
In this article, we’ll take a look into sentiment analysis approaches and explore how they contribute to enhancing customer satisfaction for companies.
What does Sentiment Analysis Mean?
Sentiment analysis, also known as analysis of opinions, is a potent method for interpreting the feelings, viewpoints, and attitudes included in textual data. Sentiment analysis’s primary goal is to determine if a text represents neutral, positive, or negative sentiments. Businesses can obtain invaluable insights into public opinion, brand perception, and customer satisfaction levels by analyzing large volumes of textual data from sources such as social media, product reviews, customer reviews, and surveys.
Basically, Sentiment analysis allows companies to listen to their customers as a whole. It does this by offering useful information that can be used to guide decision-making processes in a variety of areas, including marketing, customer support, product development, and brand management.
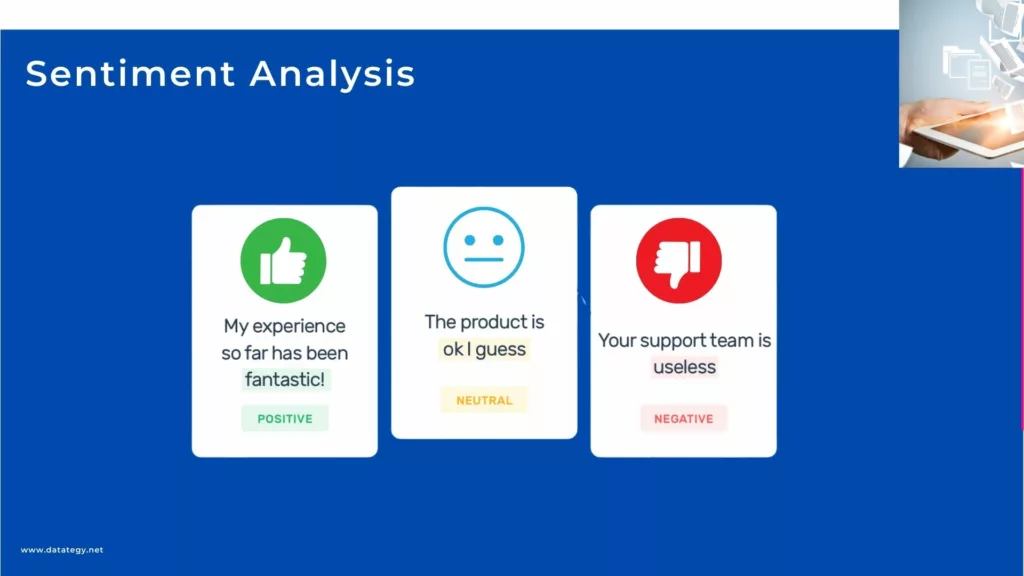
Standard NLP Pipeline
What are the Old Methods of Analyzing Feelings?
Manual Annotation
Human analysts read and annotate text to classify sentiment as positive, negative, or neutral in manual annotation, also referred to as human-driven sentiment analysis. Using this method, each text is thoroughly examined by skilled annotators who then tag each text with a sentiment based on how they understand the content. This approach depends on human judgment, understanding context, and subjective evaluation to precisely identify the attitudes and feelings expressed in the text.
Lexicon-Based Approach
To assign sentiment scores to words or phrases, the Lexicon-Based Approach in sentiment analysis uses predefined sentiment dictionaries or lexicons. These lexicons include lists of words or phrases that are connected to one of three sentiment polarities: neutral, positive, or negative. The text is tokenized into words or phrases during analysis, and the sentiment score of each token is calculated by comparing it to the lexicon’s entries. The overall sentiment of the text is then determined by adding together the sentiment scores of each individual token.
Rule-Based Systems
This approach to sentiment analysis uses a predetermined set of guidelines, or heuristics, to identify the sentiment contained in a text. Usually, grammatical constructions, linguistic patterns, or syntactic elements linked to positive, negative, or neutral sentiment serve as the foundation for these rules. These rules are applied to the text during the analysis process in order to find sentiment indicators and categorise the overall sentiment appropriately.
How AI is used in Sentiment Analysis
- Natural Language Processing (NLP): The goal of the artificial intelligence (AI) field of natural language processing (NLP) is to empower machines to comprehend, interpret, and produce human language. NLP techniques are applied to text data analysis in sentiment analysis to extract meaningful information about the sentiments expressed in the text. Lexical, syntactic, and semantic analysis are just a few of the levels at which text data is processed by NLP algorithms in order to comprehend the underlying structure and meaning of the language.
This makes it possible for AI systems to recognise linguistic patterns, words, and phrases that convey sentiment—a critical component of precise sentiment analysis. Artificial intelligence (AI) algorithms are able to determine the sentiment polarity of textual data—whether it is positive, negative, or neutral—by utilising natural language processing (NLP) techniques. This allows businesses to obtain important customer opinion insights.
- Machine Learning Models: Due to their ability to automatically classify sentiment in text data, machine learning (ML) models are essential to sentiment analysis. The labeled datasets used to train these AI-powered models assign a sentiment label (such as positive, negative, or neutral) to each text sample. The machine learning model gains the ability to recognize patterns and connections between the text data’s features and the sentiment labels that correspond with them during the training phase.
Based on the patterns discovered in the training data, the machine learning model can reliably identify the sentiment of fresh, unseen textual data once it has been trained. Because of this, businesses can effectively analyze massive amounts of textual data without requiring human intervention by automating the sentiment analysis process. Furthermore, ML models can have their performance enhanced and optimized.
Deep Learning: Deep Learning is a subset of machine learning that utilizes artificial neural networks with multiple layers (hence the term “deep”) to learn and extract complex patterns and relationships from data. In the context of sentiment analysis, Deep Learning techniques play a crucial role in automatically extracting nuanced sentiment signals from textual data, enabling AI systems to understand the underlying sentiment expressed within the text.
- Sentiment Lexicons: Also known as sentiment dictionaries or lexica, these are curated collections of words or phrases that are annotated with sentiment scores indicating their emotional polarity (e.g., positive, negative, or neutral). In sentiment analysis, AI systems leverage predefined sentiment lexicons to assign sentiment scores to words or phrases found within the text data.
By matching words or phrases in the text against entries in the sentiment lexicon, AI algorithms can determine the overall sentiment expressed in the text. Sentiment lexicons provide a valuable resource for sentiment analysis, allowing AI systems to quickly and efficiently assess the sentiment of textual data without the need for complex computational techniques. Additionally, sentiment lexicons can be customized or expanded to include domain-specific terminology or to account for linguistic variations, enhancing the accuracy and relevance of sentiment analysis in specific contexts.
What the Benefits of using AI in Sentiment Analysis?
Increased Accuracy: By quickly processing enormous volumes of textual data, AI algorithms significantly contribute to the improvement of sentiment analysis accuracy. Due to their manual and labor-intensive nature, traditional sentiment analysis methods frequently have trouble accurately analyzing large volumes of data. In contrast, businesses can extract more precise insights into customer sentiments thanks to AI-powered algorithms that can process large datasets quickly.
Artificial intelligence (AI) algorithms can more accurately classify sentiment by utilizing sophisticated techniques like machine learning and natural language processing (NLP), which enable the algorithms to effectively discern linguistic nuances and context. Businesses can now make more informed decisions based on trustworthy insights into the opinions and preferences of their customers thanks to this increased accuracy.
Real-time Insights: Artificial Intelligence facilitates sentiment analysis in real-time, furnishing enterprises with prompt feedback on customer attitudes and viewpoints. Real-time data analysis is challenging with traditional sentiment analysis techniques because they frequently rely on labor- and time-intensive manual processes. However, businesses can monitor and respond to customer feedback and sentiments as they happen with the help of AI-powered sentiment analysis solutions, which can process data quickly and efficiently.
Businesses may respond quickly and efficiently to customer concerns and preferences by staying flexible and responsive thanks to this real-time analysis. Businesses are able to maintain a competitive advantage by using AI to analyze customer sentiment in real-time and stay ahead of market trends.
Automation: Improving efficiency and scalability is one of the main advantages of automation in sentiment analysis. AI algorithms make it easy for businesses to analyze enormous datasets because they can process large volumes of data quickly and accurately. Because of its scalability, businesses can increase the volume of data they analyze and still maintain accuracy and performance when scaling their sentiment analysis efforts.
Furthermore, automation expedites the sentiment analysis procedure, saving time and money over manual data analysis. Businesses can analyze data more effectively and make quicker, more informed decisions based on real-time insights into customer sentiments and preferences by automating repetitive tasks and workflows.
Demystifying AI: A Comprehensive Guide to Key Concepts and Terminology
This guide will cover the essential terminology that every beginner needs to know. Whether you are a student, a business owner, or simply someone who is interested in AI, this guide will provide you with a solid foundation in AI terminology to help you better understand this exciting field.
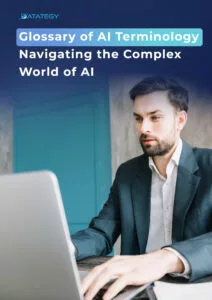
Advanced NLP Applications: How is papAI 7 used in Intelligent Text Analysis?
Overview of papAI 7
With papAI 7, enterprises can now leverage artificial intelligence in a completely new way. It is an advanced end-to-end AI platform. Users can simplify their AI development process from data preprocessing to model deployment with papAI 7’s extensive feature set. Data preprocessing, feature engineering, model training, evaluation, and deployment are just a few of the many AI tasks that can be supported by this platform’s many tools and functionalities.
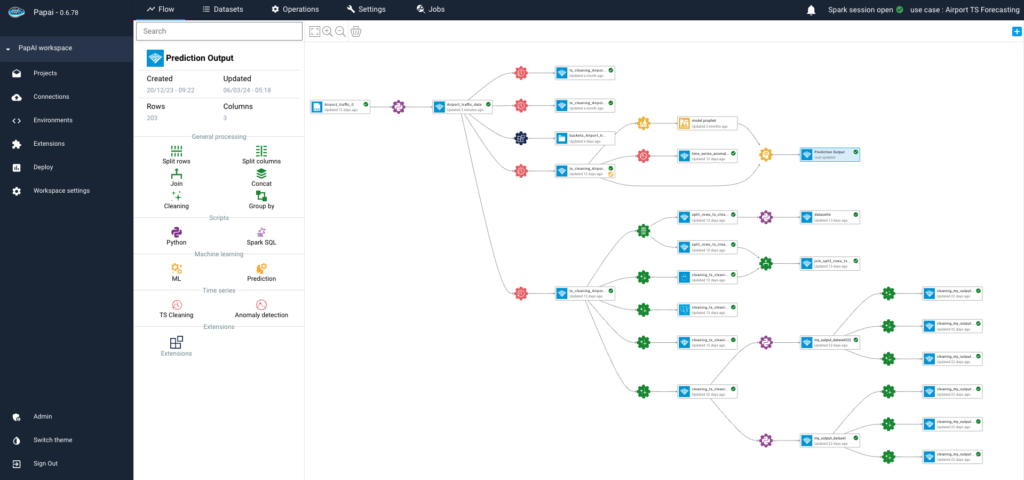
papAI 7 Workflow
Financial Document Entity Detection using NLP : A Comprehensive Guide
Identifying and extracting crucial data from financial documents requires the use of cutting-edge technology like machine learning and natural language processing (NLP) to identify certain entities and phrases. Terms might include acronyms, industry-specific jargon, rules, and KPIs, while entities could include names of businesses, people, dates, financial numbers, and more.
This use case is essential to the financial industry’s process simplification efforts because it allows stakeholders to obtain insightful information from a variety of financial data sources.
The precise extraction of entities and phrases enables organizations to make confident and accurate judgments, whether they are identifying possible dangers, spotting fraudulent activity, or doing market analysis.
1- File Upload & Data Visualization
In this initial step, we leverage the capabilities of papAI 7 by uploading a text file to gain deeper insights into our data. By harnessing papAI’s advanced analysis tools, we unlock a more detailed perspective of our input data.
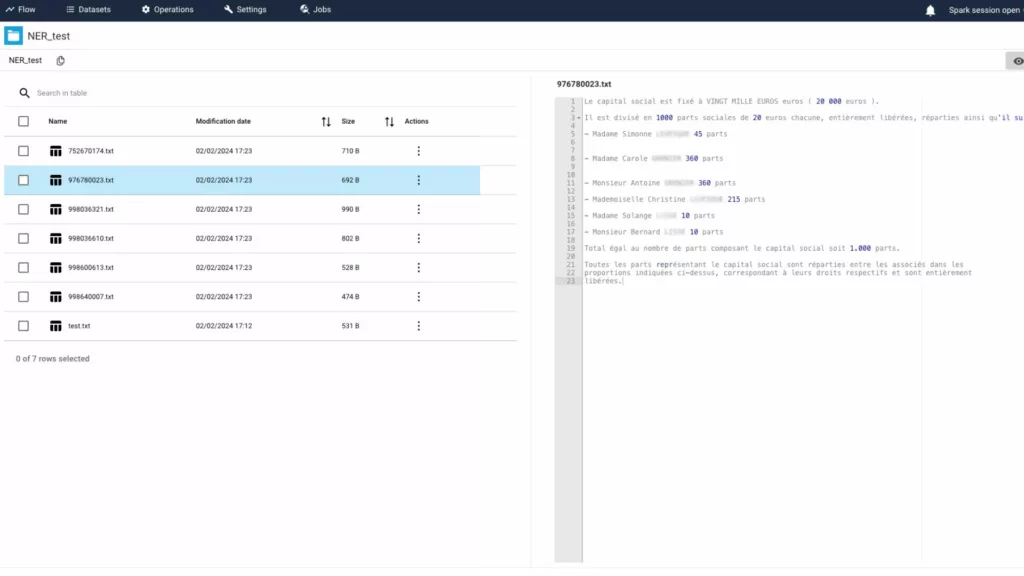
2- Entity Labeling & Recognition
In this second step on papAI 7, we engage in the process of selecting specific entities within our text data for labeling and extraction. Through advanced named entity recognition techniques, papAI identifies and extracts key information such as names of people, organizations, locations, dates, and more. This step lays the foundation for deeper analysis and insights into our data.
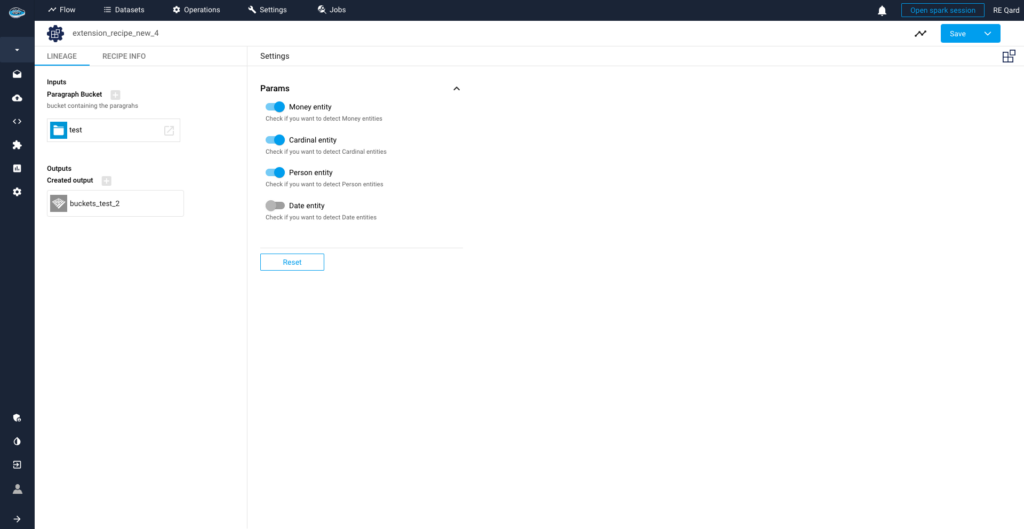
3- Pre-Relationship Assessment Stage
During this pivotal step on papAI 7, we focus on examining the lists of entities that have been extracted from the text data. Through evaluation and analysis, we ensure the accuracy and relevance of the identified entities before proceeding to establish relationships between them. This phase serves as a critical quality control checkpoint, ensuring the integrity and reliability of subsequent analyses and insights derived from the data.
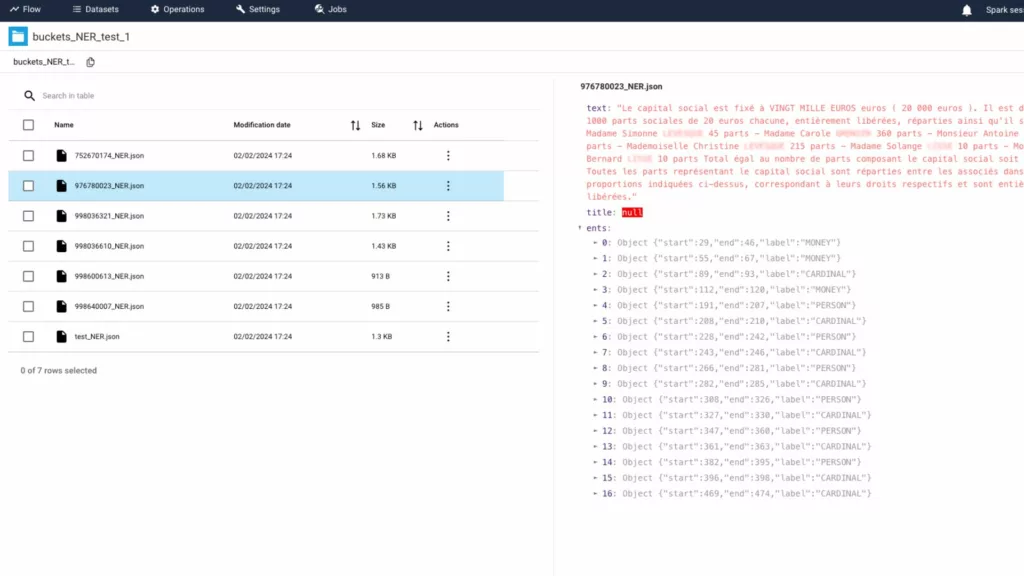
4- Highest Probability Result Extraction
In this concluding step on papAI platform, we attain our initial results by validating the relationships between entities extracted from the text data. Through meticulous validation processes, we identify and prioritize relationships with the highest probability, ensuring the reliability and accuracy of our extracted data.
By selecting values with the highest probability, we obtain the most dependable results, facilitating informed decision-making and strategic insights based on the analyzed data.
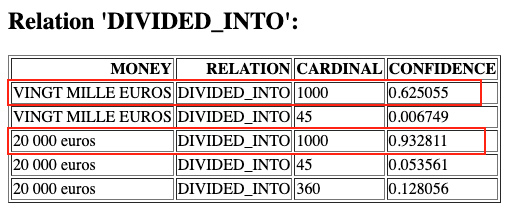
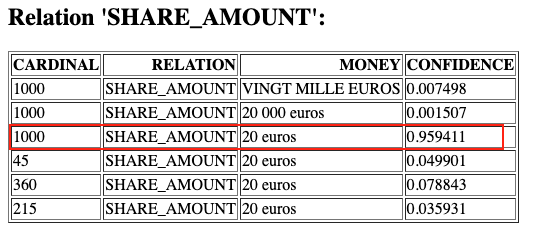
Create Your Own Custom NLP Tool to Examine Your Different Text Files with papAI 7
Using advanced techniques including sentiment analysis, entity identification, and topic modeling, papAI helps users to better comprehend a variety of text data sources, extract pertinent information, and spot trends.
The opportunities for data-driven innovation and decision-making are unlimited when using papAI’s natural language processing (NLP) capabilities to analyze financial records, customer reviews, or social media postings.
Are you interested in using specialized NLP tools to examine your text documents? To learn more about how papAI 7 can revolutionize your data analysis procedures, schedule a demo with a Datategy AI expert now.
Interested in discovering papAI?
Our AI expert team is at your disposal for any questions
How Do AI Predictive Models Estimate Car Prices?
How Do AI Predictive Models Estimate Car Prices? Valuation methods...
Read More“DATATEGY EARLY CAREERS PROGRAM” With Tommy GUYOT
“DATATEGY EARLY CAREERS PROGRAM” With Tommy GUYOT Hello! My name...
Read More