Transform Defense Logs & Rapport into Situational Awareness with AI...
Read MoreSmart Grids Unleashed:
How is AI Used to Forecast Energy Consumption?
Table of Contents
ToggleEnergy consumption is a significant concern in today’s society. The growing need for energy and the subsequent increase in energy costs highlight the importance of finding methods to improve energy usage. Utilizing artificial intelligence (AI) to predict energy consumption and enhance the operations of smart grids is a potential remedy for this issue.
The International Energy Agency (IEA) estimated that AI could reduce global energy consumption by 30% by 2040. This technology has the potential to revolutionize energy management and consumption. By providing cost-effective information and lowering expenses, it would benefit all stakeholders, including individuals, organizations, and governments.
Find out how papAI can improve the deployment of AI projects in the Energy industry here.
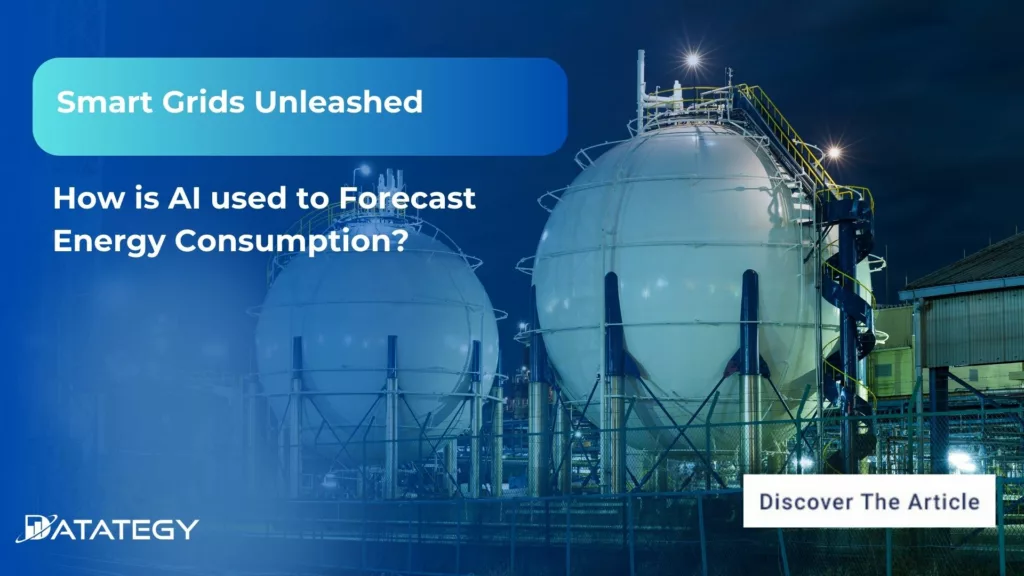
In this article, we look at the real-world applications of AI in forecasting demand and consumption in the energy industry.
What do We mean by " Smart Grids "?
According to Wikipedia, The smart grid represents the full suite of current and proposed responses to the challenges of electricity supply. For us Smart Grids manage and optimize the flow of power, it make use of a network of digital communication technologies, cutting-edge sensors, and real-time data analytics.
Smart grids provide a dynamic approach to energy management because they are more responsive and flexible than traditional networks. They are complex systems that seamlessly integrate many parts, such as sensors, automation systems, smart meters, and communication networks, to generate an intelligent ecosystem that changes how we produce, distribute, and use energy.
What are its Key Components and How does it work?
1- Smart meters and sensors: Consider attempting to control the energy use of a whole city without knowing who is consuming what, when, or where. Sensors and smart meters can help with that. These tiny devices, which continuously gather real-time data on power use, voltage levels, and system health, function as the smart grid’s nervous system.
Customers may now view their energy usage without waiting for monthly bills thanks to smart meters. Rather, they receive immediate information that enables them to modify their usage and reduce expenses. On the utility side, sensors track power flow, identify outages, and identify inefficiencies, enabling quicker reaction times and improved grid management.
2- AI-Powered Data Analytics: Although smart grids produce enormous volumes of data every second, raw data is insufficient on its own. As the operation’s brain, AI analyses trends, forecasts changes in demand, and optimizes the allocation of energy.
In addition to predicting, AI can identify abnormalities, such as abrupt increases in power or equipment failures, which enables utilities to address problems before they become complete blackouts. To put it briefly, AI improves the grid’s intelligence, responsiveness, and economy.
3- Automated Control Systems: Without automation, a smart grid wouldn’t function any better than a self-driving automobile that still requires a driver. In order to maintain smooth operation, automated control systems make sure that power distribution rapidly adapts to supply and demand.
Additionally, these systems react instantly to problems such as broken electrical lines or variations in voltage. Reliability and efficiency are increased by automated controls that identify and address issues immediately rather than waiting for human involvement. This implies a system that can manage erratic energy demands, fewer blackouts, and reduced expenses.
4- Decentralized Energy: Decentralization strengthens the grid. Energy is derived from several sources dispersed over various regions rather than relying on a small number of massive power plants. There is less chance of widespread disruptions if one source fails since other sources can take over.
Additionally, the grid can better balance supply and demand when AI controls energy flow. For example, AI makes sure that extra energy produced by solar panels throughout the day is either stored or sent to regions with greater demand. By doing this, waste is avoided and efficiency is increased.
Smart networks that embrace decentralized energy lessen carbon emissions, cut dependency on fossil fuels, and open the door to a cleaner, more sustainable future.
What are the AI Techniques for Energy Prediction
Time Series Analysis and Demand Forecasting
One statistical method for working with time-ordered data points is time series analysis. It entails examining past usage data to find trends, seasonal patterns, and anomalies in the context of energy consumption. Because it predicts future energy demands based on historical consumption patterns, this research is essential for demand forecasting.
Long Short-Term Memory (LSTM) networks are a type of advanced AI model. They help improve the accuracy of time series forecasting. These models work well for predicting energy use. They can learn from data that comes in sequences and understand long-term patterns. Energy suppliers can use time series analysis to predict demand changes. This helps them balance supply and demand by adjusting their operations.
Neural Networks
Grid efficiency has been significantly impacted by neural networks, especially deep learning models, which have made demand-side management more efficient and increased the accuracy of energy consumption estimates. Recurrent neural networks (RNNs) and convolutional neural networks (CNNs) are frequently employed in this setting.
Because of their skill in identifying geographical characteristics, CNNs have been used to forecast the production of renewable energy by analyzing data from a variety of sources, including satellite photos and weather maps. Because they can process sequential data, RNNs—including LSTMs—have been used to predict energy demand by identifying trends in consumption data across time. Neural networks are used in energy systems to improve grid stability, optimize resource allocation, and make forecasts that are more accurate.
Reinforcement Learning for Real-Time Adaptation
In the field of machine learning known as reinforcement learning (RL), an agent gains decision-making skills by interacting with its surroundings in order to accomplish a certain objective. RL has been used in energy systems to create adaptive control schemes that can react to changes in energy supply and demand in real-time.
Energy storage systems, for example, have been optimized to charge and discharge in response to price signals and demand variations through the application of RL algorithms. This real-time adaptability improves the integration of renewable energy sources, lowers operating costs, and balances the grid. Additionally, RL has been used in demand response programs, where it learns the best ways to modify customer energy use during peak hours to preserve grid stability.
What are the Challenges in Energy Forecasting?
The problems associated with the conventional approaches to energy prediction give rise to the necessity of forecasting energy use. The dynamic nature of energy usage is frequently difficult for conventional methods to adjust to, which results in errors and inefficiencies.
Unexpected demand surges, weather changes, and the incorporation of renewable energy sources are just a few examples of the complicated factors that necessitate a more advanced forecasting system.
The limitations of conventional energy forecast techniques are addressed by smart grids, which provide a revolutionary alternative. Smart grids improve the precision and responsiveness of energy consumption predictions by utilizing cutting-edge technology like artificial intelligence and real-time data analytics.
With the inclusion of factors influencing consumption patterns, these intelligent systems offer a comprehensive picture of the energy environment. The amalgamation of automated methods, smart metres, and sensors enables the ongoing observation of energy use, hence enabling more precise and prompt forecasts. Consequently, smart grids open the door for robust and adaptable energy management solutions in addition to easing the difficulties associated with traditional forecasts.
AI's Role in Energy Forecasting
Machine learning algorithms examine weather data, past consumption trends, and other pertinent factors. These algorithms can make better predictions because they can understand complicated connections and adapt to changing situations. By using machine learning, this method helps energy forecasting models improve their ability to predict future outcomes.
1- Processing Data in Real-Time in Smart Grid Settings:
AI’s ability to handle real-time data in smart grid situations is one of its unique qualities in energy forecasting. AI-enabled smart grids can handle an endless supply of data from sensors, smart metres, and other Internet of Things devices. With these real-time processing capabilities, projections may be instantly adjusted in response to new patterns or unanticipated shifts in the behavior of energy usage. Consequently, utilities and grid operators can respond to demand changes with an unparalleled level of agility, which guarantees a more robust and flexible energy infrastructure.
2- Predictive Analytics for Improving Grid Reliability:
Through predictive analytics, AI actively contributes to improving grid dependability in energy forecasting, going beyond simple prediction accuracy. AI is able to predict any problems in the electrical system, including overloads or equipment breakdowns, before they happen by utilising sophisticated algorithms. Models for predictive analytics can offer information about the condition of grid components, enabling preventive maintenance and reducing downtime. This proactive strategy improves operational effectiveness while also adding to the electricity grid’s overall stability and dependability.
3- Risk Analysis and Scenario Planning
AI is so advanced that it may be used in energy forecasting for risk analysis and scenario planning. Artificial intelligence (AI) systems can evaluate the probability of various occurrences, such as severe weather, abrupt demand surges, or equipment malfunctions, by utilising probabilistic models and simulations. Energy stakeholders are empowered by this insight to proactively plan and distribute resources in accordance with possible hazards. By use of scenario planning, policymakers may investigate several scenarios and formulate robust approaches, guaranteeing a more flexible reaction to unanticipated obstacles.
4- Dynamic Demand Response Management:
AI is important for managing energy demand because it helps energy systems adapt quickly to changes in customer behavior. AI systems can predict changes in energy use by analyzing past data and considering factors like weather and events. AI is crucial for managing energy demand. It enables energy systems to adapt swiftly to changes in customer behavior. AI systems analyze past data and consider factors like weather and events to predict changes in energy use. Utilities can optimize the energy grid by using this data. They can encourage customers to use energy during times of lower demand through dynamic pricing techniques.
5- Cybersecurity Measures for Data Protection
Because AI depends so much on data to make correct predictions, strong cybersecurity becomes essential. Modern security measures must be included by AI systems used in energy forecasting in order to safeguard sensitive data from online attacks. In addition to protecting the forecasting process, maintaining data integrity and confidentiality also upholds stakeholders’ and customers’ confidence in the security of the energy infrastructure.
From Data to Action: How AI is Transforming the Energy Industry?
AI and ML can change how we make, deliver, and use energy. They use smart algorithms and data analysis to make energy systems more efficient, safe, and sustainable. Learn more in this white paper.
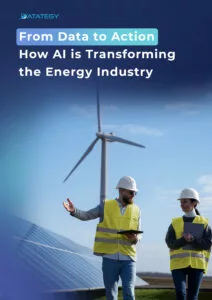
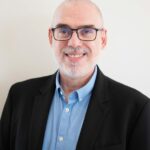
“At Datategy, we see AI as a game-changer for predicting energy use. Smart grids powered by AI make it possible to track and manage energy demand in real-time, helping reduce costs and boost efficiency. AI is about making smarter decisions for a cleaner, more reliable energy future.
Jean-Marc Briquet
Global Sales Director
Case Study: Using papAI Solution to Forecast Energy Consumption
Context
To determine the amount of energy that must be produced and distributed to the populace without having excess or insufficient energy, which might be restrictive, it is crucial to analyze the consumption pattern. But using our use case on energy consumption, we will demonstrate to you the effectiveness of ML models in predicting this trend and assisting energy suppliers in better organizing their production—all made possible by our papAI platform.
1- Importing the Dataset
First of all, you need a baseline of data that gathers information about a load of energy consumed. This is why we are using, for this use case, the hourly power consumption from the company PJM that serves the Northeastern states in the US with electricity. To use this dataset in the papAI platform, we need to import it from a data source.
Thanks to its different connectors, you can import any dataset from different sources, either from your local machine, an external DB, or an API. After selecting the data source where your dataset is located, you just simply select the desired dataset and tune up some settings according to your liking and it will be shown in your project ready-to-use for any operation available.
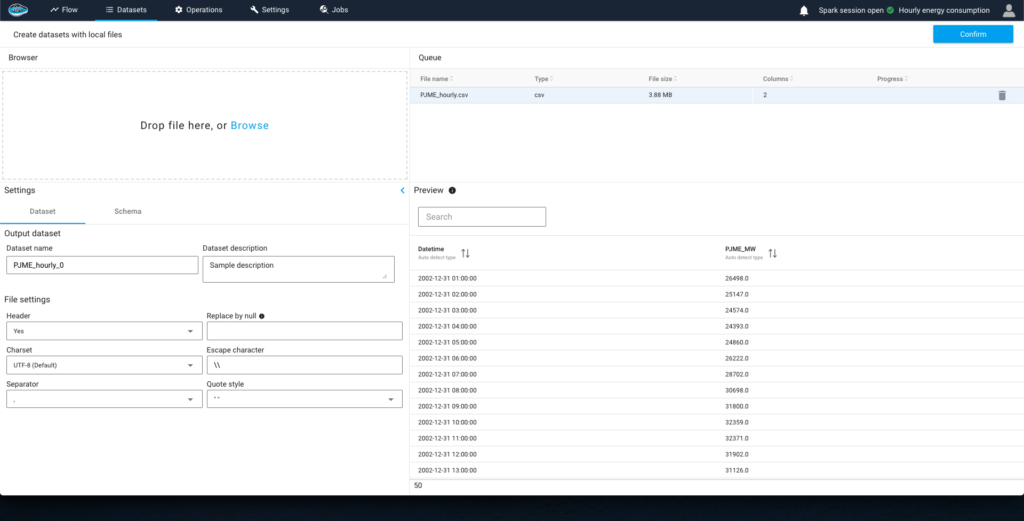
2- Examine and Handle your Dataset
Before delving into machine learning, it is imperative that we examine any discernible patterns that may aid in establishing the pipeline’s baseline for training, as well as any inconsistencies that must be corrected to guarantee optimal model training outcomes. In our instance, seasonality and a pattern are seen year; nonetheless, we will just consider the 2017 period and make predictions for 2018. This dataset is ready for training after some feature engineering.
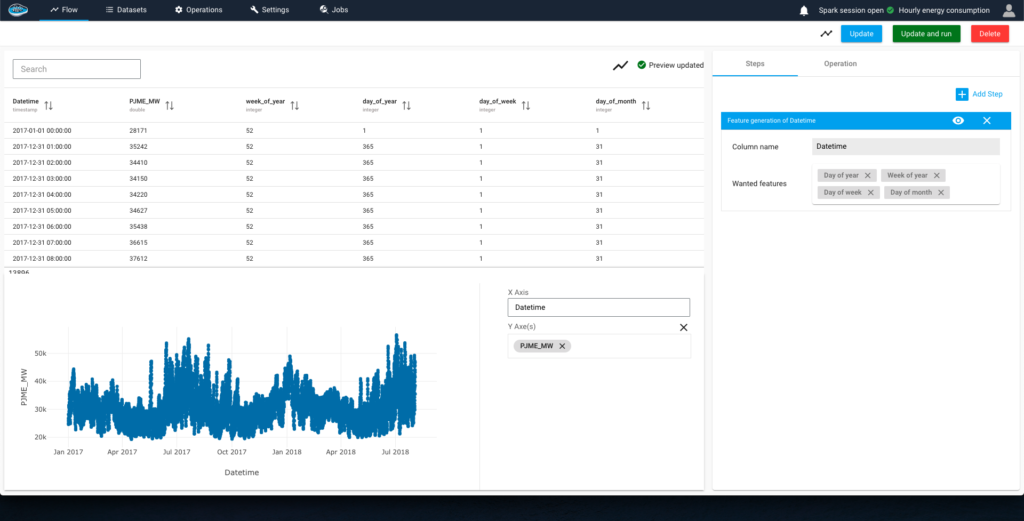
3- Build your own ML Pipeline
Once the training dataset is ready, you may create your own machine learning use case to train and evaluate models, track their performance, and select the best model for your company.
We must optimize the pipeline to train the model, which is why we use the AutoML module, which makes it simple and easy to construct utilizing a variety of training parameters and built-in methods. Our use case involves time-series forecasting, and before we access the experiments, we do a sanity check to confirm that the DateTime points were sampled appropriately. Once the automated check is complete, you may experiment and adjust a few parameters to predict the desired timeframe.
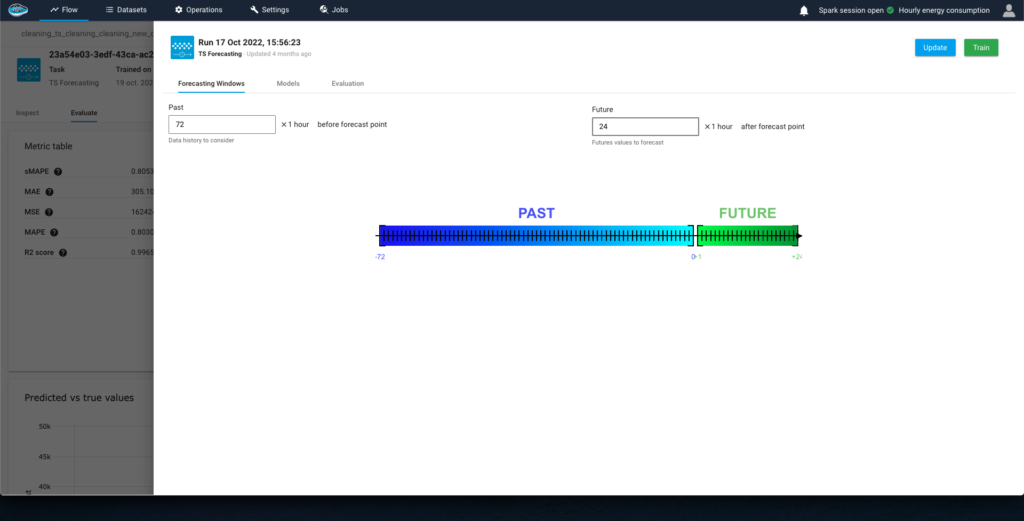
4- Evaluate the Forecasting Accuracy
Once the runs are successful, you can assess the model’s predicting accuracy and see whether it consistently follows the same pattern. The Evaluation module is where you may carry out this monitoring. It provides you with access to metrics pertaining to the model’s prediction, the error ratio, and a graph that shows the actual and anticipated data. When selecting the best model to use in a practical use case, all of these techniques might be helpful.
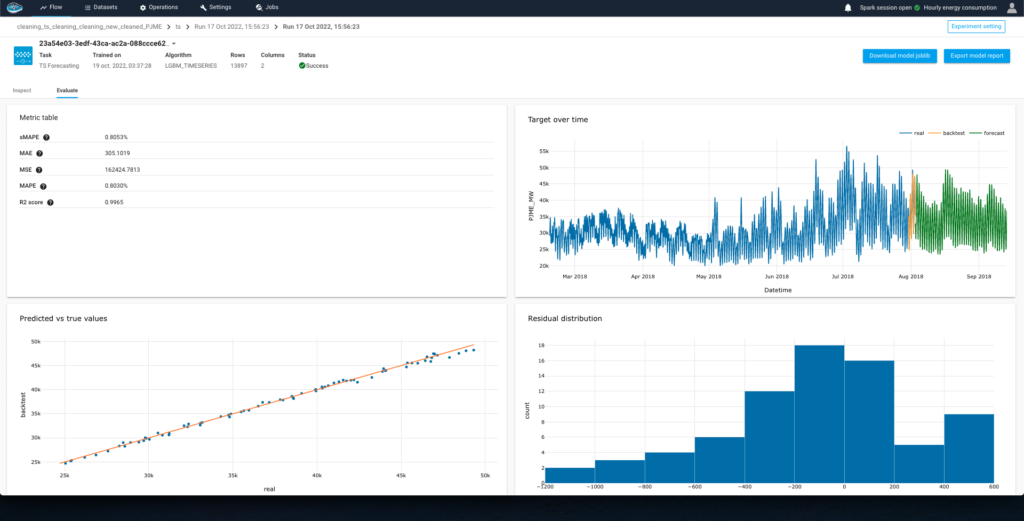
5- Predict values and deploy
Whenever the appropriate model has been selected, you can encourage it to apply its prediction powers to any dataset that has the same schema and evaluate its results. Once the model is a good match, you may utilize it to address your business use case and any external purpose.
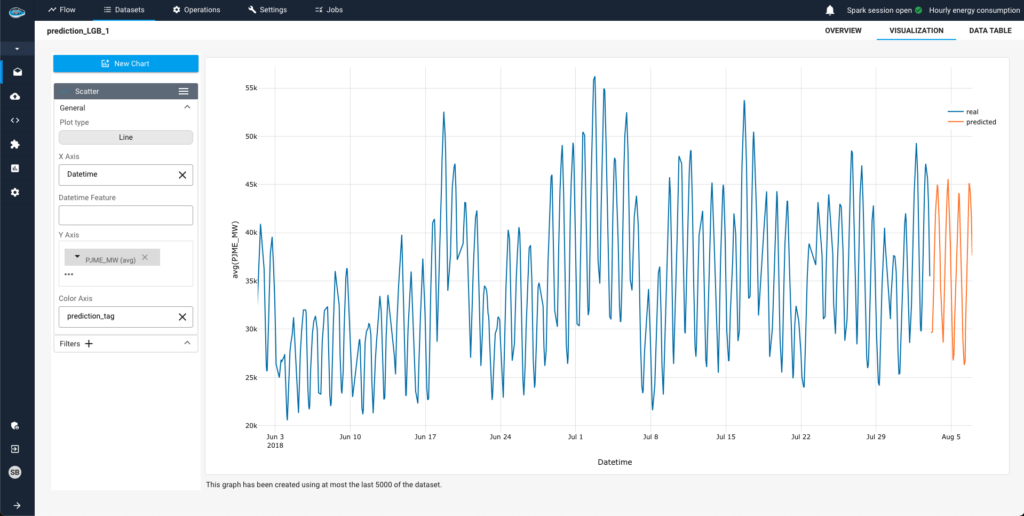
Create your Own AI-based Tool to Address Your Specific Use Case in Energy Industry using papAI
AI is being used more to predict energy consumption as the demand for energy increases. This helps businesses make sustainable choices. AI can process a lot of data and analyze patterns to give real-time information on energy use. This data can then be used to create effective energy management plans, save money on energy, and improve energy efficiency.
Schedule a demo right away to learn about the endless possibilities that come with leveraging papAI to exploit your data. Your data-driven initiatives will be transformed by our experts as they guide you through the process and demonstrate how to use papAI’s platform.
Smart grids are advanced energy distribution systems that utilize digital communication technologies, sensors, and real-time data analytics to optimize energy flow. Unlike traditional grids, which operate on a fixed supply-demand model.
AI enhances energy forecasting by analyzing historical usage data, weather patterns, and real-time sensor inputs. Techniques such as time series analysis, neural networks, and reinforcement learning help identify patterns, and predict demand fluctuations.
Energy forecasting faces challenges such as unpredictable demand surges, weather variability, and the integration of renewable energy.
Interested in discovering papAI
Our team of AI experts will be happy to answer any questions you may have
Why AIOps Is Key to Cyber Threat Detection in Defense?
Why AIOps Is Key to Cyber Threat Detection in Defense?...
Read MoreHow AI Transforms Predictive Maintenance in Defense Equipment
How AI Transforms Predictive Maintenance in Defense Equipment In a...
Read MoreHow to Scale AI Without Breaking Your Infrastructure in 2025
How to Scale AI Without Breaking Your Infrastructure in 2025...
Read More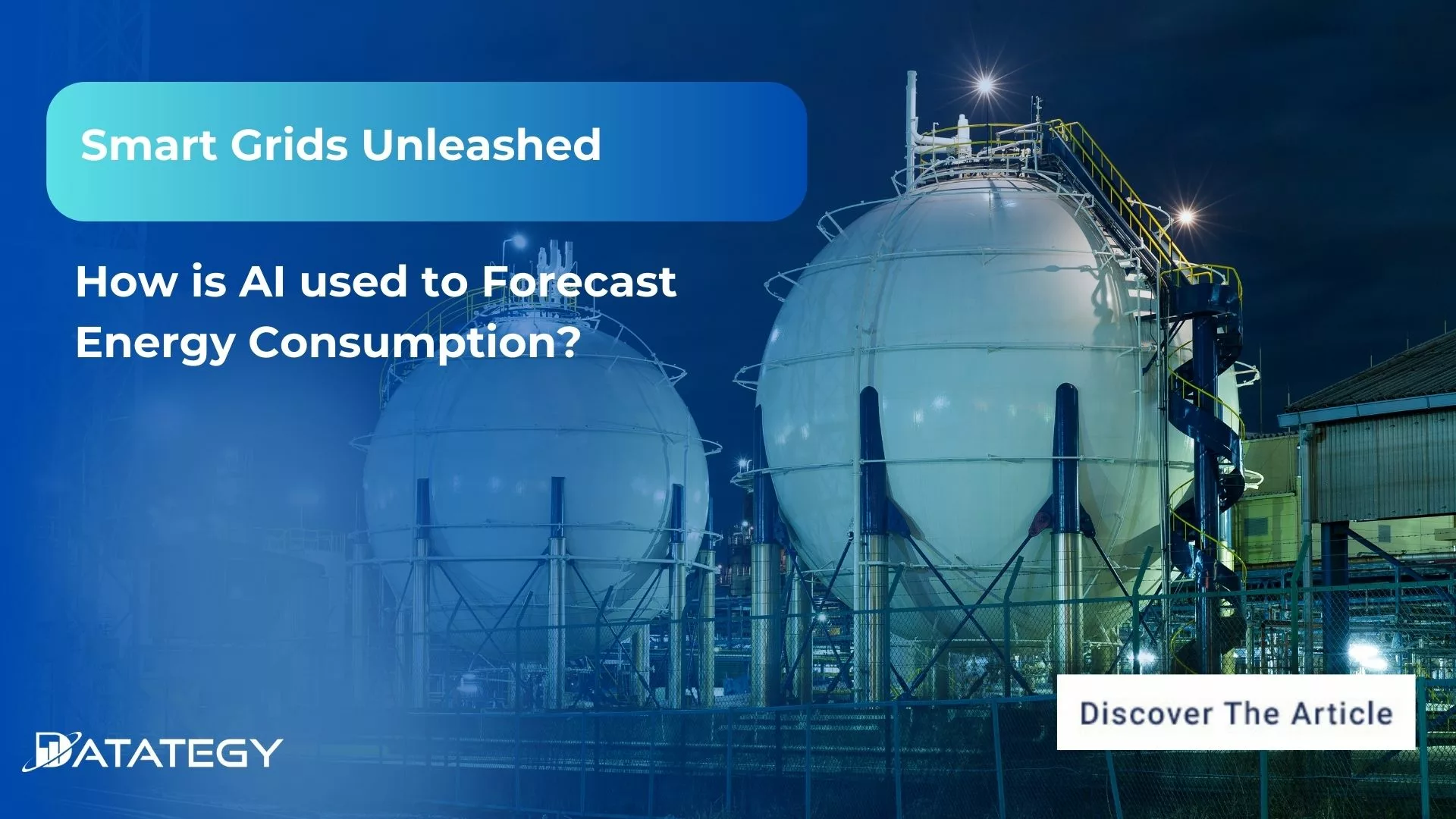
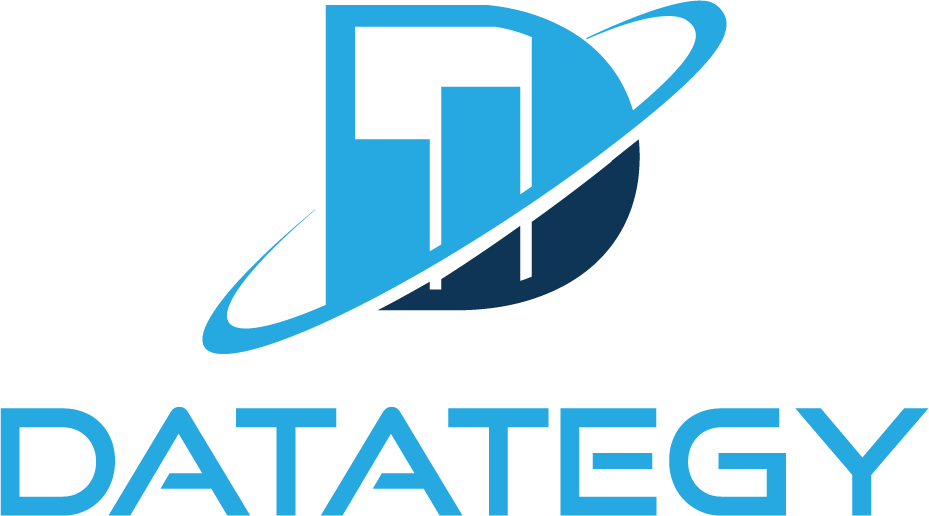