Applying NLP for Intelligent Document Analysis using papAI 7 AI...
Read MoreNext-Gen Wind Farms:
Wind Turbine Optimization with AI
Table of Contents
ToggleRenewable energies have become an essential source of energy production in recent years. Among these technologies, wind power has established itself as a major alternative to conventional energy in some countries . According to last research, Wind power, named also (Clear Energy) is the fastest-growing source of renewable energy in the world. In 2023, wind power generated 10.7% of the world’s electricity.
Find out how papAI can improve the deployment of AI projects in the Renewable Energy Industry.
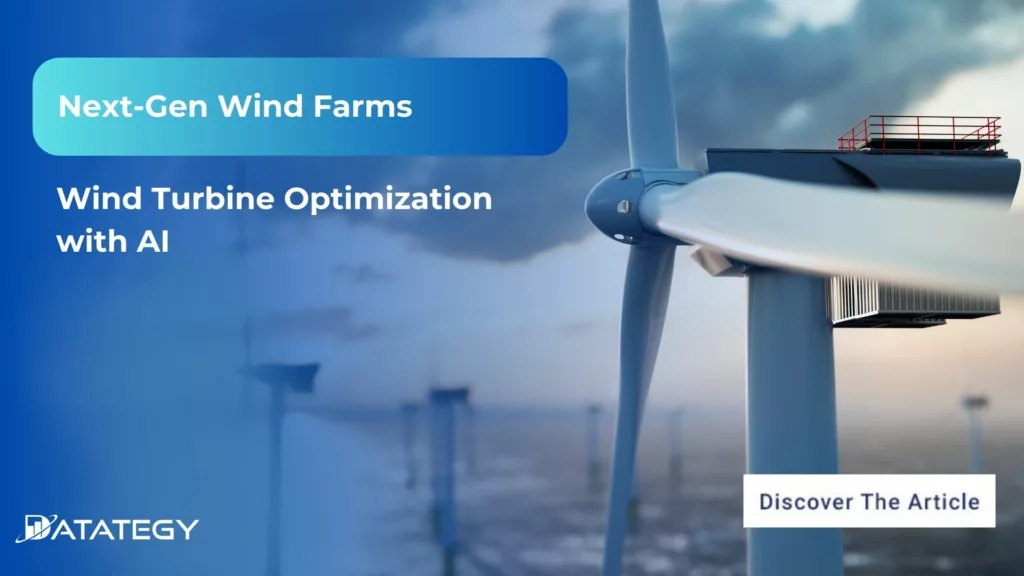
In this article, we will explore how artificial intelligence can optimize wind turbine processes and its ability to enhance innovation in this field.
The History of Wind Energy
Once a part of prehistoric life, wind energy has evolved into a major factor in the current revolution in renewable energy. Wind kinetic energy was first captured when windmills were used for water pumping and milling in the past.
When wind turbines that could produce energy were developed in the late 19th century, it was a revolutionary step forward. Yet wind energy didn’t become widely accepted as a competitive alternative to conventional power sources until the later part of the 20th century.
Large wind farms, made up of tall turbines placed in areas with the best wind conditions, characterize the current wind energy environment. Wind farms, both offshore and onshore, are becoming major players in the worldwide energy transition. With wind energy being a clean and plentiful source of electricity, it has become the front-runner in the green energy movement as the negative environmental effects of fossil fuels become more obvious.
Technological developments in the last several decades have ushered in a new age for wind energy. Next-generation wind turbines include more creative designs, higher energy output, and enhanced efficiency.
Overview of Wind Energy Industry
The announcement of the anticipated 2 terawatt (TW) milestone being achieved in a mere seven years represents a remarkable acceleration in the growth of global wind power capacity.
This ambitious target, set for the period between 2023 and 2030, signifies a substantial increase compared to the projections outlined in the 2030 global outlook released just a year prior.
According to GWEC Market Intelligence, the forecast for total wind power capacity additions during this timeframe has been revised upward by an impressive 143 gigawatts (GW), reflecting a significant 13% year-over-year (YoY) adjustment.
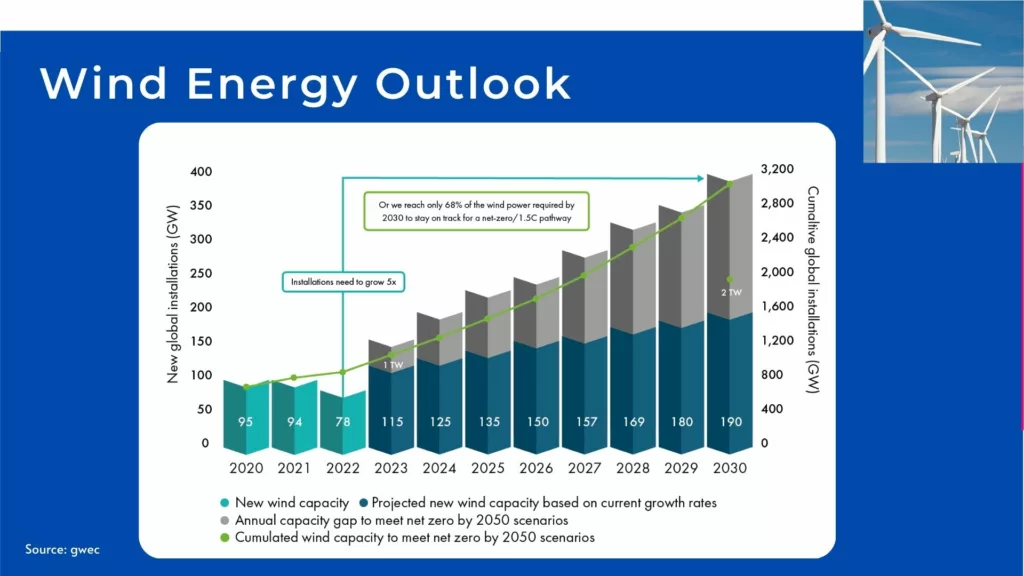
Perspective of new installations for onshore and offshore wind in the region (GW)
- North America: Over the next five years, it is anticipated that 60 GW of onshore wind capacity will be installed in North America, with 92% of that capacity being developed in the US and the remaining portion in Canada.
- Europe: The European onshore market is expected to see significant growth starting in 2024, driven by the resurgence of robust growth in well-established European markets including Germany, Spain, the UK, France, Italy, and Turkey.
- Middle East and Africa: Over the course of the following five years (2023–2027), 17 GW of additional capacity is anticipated to be installed, of which 5.3 GW will come from South Africa, 3.6 GW from Egypt, 2.4 GW from Saudi Arabia, and 2.2 GW from Morocco.
- South America: According to GWEC Market Intelligence, throughout the next five years, this area will add 26.5 GW of onshore wind power, with 78% of the increases coming from Brazil, Chile, and Colombia.
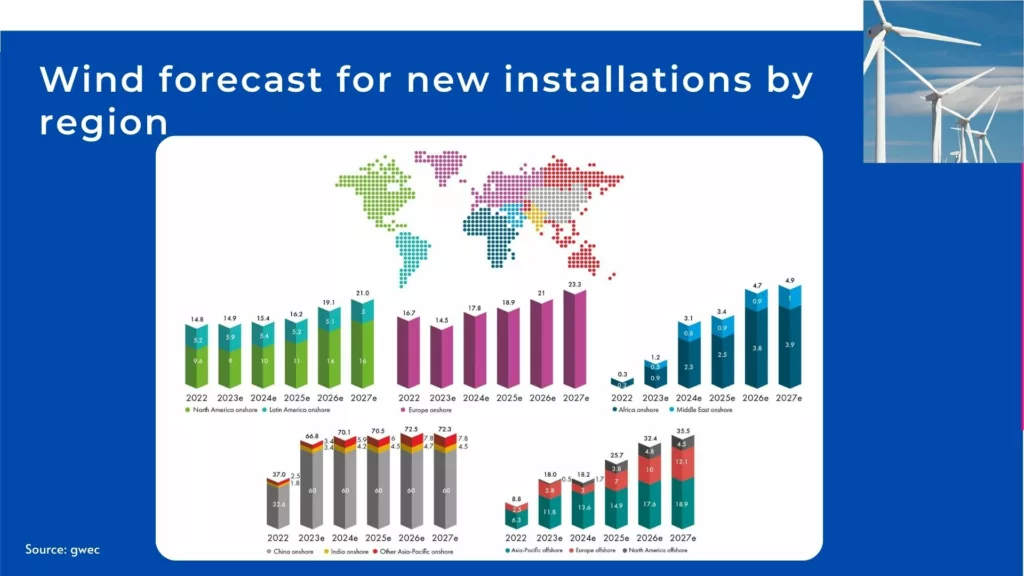
Advantages of Integrating AI in Wind Farm Operations
Enhanced Efficiency and Output Optimization
Analyzing and monitoring turbine performance in real-time is made possible by the incorporation of artificial intelligence (AI) into wind farm operations. In response to shifting wind conditions, AI algorithms can dynamically modify turbine settings, like blade pitch and yaw angles, to maximize energy capture. As a result, energy output rises and overall efficiency is improved.
AI can improve the efficiency of wind turbines by up to 20%. Study by the National Renewable Energy Laboratory (NREL)
Making Decisions Based on Data
AI provides thorough data analytics to wind farm operators, enabling them to make well-informed decisions. Operators may use machine learning algorithms to analyse both historical and current data to find trends, improve operating tactics, and make data-driven choices that improve the wind farm’s overall productivity and profitability.
Remote Monitoring and Control
With AI, wind farm assets may be remotely monitored and controlled. It is no longer necessary for operators to be physically present on-site since they may access real-time data and control systems from centralized locations. Reduced issue response times, increased operational efficiency, and decreased operating expenses result from this.
AI can increase the lifespan of wind turbines by up to 10%. Study by the University of California.
Cost Saving
In order to maintain wind farms’ sustainability and economic viability, cost-cutting methods are essential. Using cutting-edge technology, including artificial intelligence (AI), offers a variety of ways to save a significant amount of money.
AI can reduce the cost of wind energy by up to 15%. Study by the Technical University of Munich found
From Data to Action: How AI is Transforming the Energy Industry
The integration of AI and ML technologies in the energy industry has the potential to revolutionize the way we produce, distribute, and consume energy. By leveraging advanced algorithms and data analytics, AI and ML can improve the efficiency, safety, and sustainability of energy systems. Find out more in this white paper.
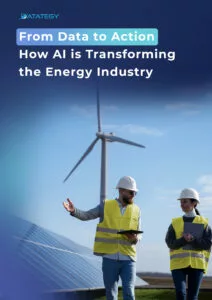
Real-Word Use Cases of using AI in Wind Turbine Optimization
Predictive Maintenance
One of the most important aspects of wind turbine optimization is predictive maintenance, which combines strategic foresight with cutting-edge technologies.
This method essentially uses Artificial Intelligence (AI) algorithms to carefully examine a large number of data points. In addition to a multitude of old maintenance logs, these data include real-time inputs from sensors built into the turbines. AI algorithms examine this large dataset to identify trends, abnormalities, and early warning signs of possible problems in the turbine’s parts.
Predictive maintenance’s proactive approach has significant implications for the wind energy industry. Maintenance teams may strategically react before concerns increase, minimizing downtime and optimizing operational efficiency, by correctly estimating when individual components are likely to fail.
In the field of wind energy, where turbines are frequently located in remote areas, making prompt on-site repairs difficult, this predictive capacity is very helpful. Long-term enhanced energy output is achieved as a result of not just lower maintenance costs but also a significant increase in the turbine’s operating lifespan.
Fault Detection and Diagnostics
In the world of wind energy, the smooth operation of complex turbine parts is critical to obtaining peak performance. A key strategy for guaranteeing the dependability and effectiveness of wind turbines is fault detection and diagnostics, which are supported by cutting-edge technical solutions.
The advanced Artificial Intelligence (AI) algorithms at the heart of this technical strategy can comprehend a wide range of sensor data produced in real-time by the turbines. These algorithms carefully examine changes in variables like vibrations, temperatures, and power outputs in an effort to spot abnormalities from standard operating circumstances that could point to possible problems.
To identify patterns linked to particular issues, large datasets are combed through by AI algorithms that function as watchful sentinels in the complex world of fault detection and diagnostics.
By incorporating past performance data, the algorithms are further improved, which enables them to develop and improve their diagnostic accuracy over time.
The technology notifies maintenance teams of the exact problem and its likely underlying cause as soon as a potential malfunction is identified. By enabling prompt intervention by maintenance staff and preventing small issues from becoming severe interruptions, this advanced warning method minimizes downtime.
Energy Forecasting
It becomes clear that energy forecasting is essential for efficient resource management and grid integration.
This technological method uses sophisticated Artificial Intelligence (AI) algorithms to process historical energy production records, real-time turbine performance parameters, and meteorological data in a methodical manner.
The main goal is to forecast wind patterns for the future with unmatched precision, giving stakeholders vital information about the anticipated production of energy over certain periods of time.
The process of energy forecasting entails the ongoing examination of several factors, including air pressure, wind direction, and speed. AI systems search through this complicated data landscape for patterns and connections, sometimes with the use of machine learning techniques.
The forecasting models are further improved by the addition of historical energy production data, which enables the modification and improvement of projections based on previous performance.
The end product is a highly sophisticated forecasting system that can provide probabilistic scenarios to take into account the inherent uncertainties in weather patterns in addition to precise estimates of energy output.
Weather Simulation and Condition Monitoring
Artificial Intelligence (AI)–based weather simulation is a highly technical method that has significant effects on turbine performance. In order to generate scenarios that increase productivity and simulate and anticipate wind patterns with an increased level of precision, artificial intelligence (AI) algorithms are integrated with sophisticated weather models.
These algorithms create a dynamic simulation environment that mimics real-world circumstances by analyzing a wide variety of meteorological variables, including temperature, air pressure, wind speed, and direction.
In wind turbines, condition monitoring is a crucial technological strategy to guarantee the ongoing good health and peak performance of vital parts.
A complex network of sensors that are thoughtfully inserted throughout the turbine construction form the basis of this method, gathering a wide range of real-time data on important aspects. These sensors provide a thorough picture of the turbine’s working state by keeping an eye on factors including vibrations, temperatures, rotor speed, and hydraulic pressures.
The integration of Artificial Intelligence (AI) algorithms, which carefully analyze this data to identify patterns suggestive of possible problems or departures from normal operation, is where the technological intricacy lies.
Wind Resource Assessment
An example of a technological paradigm change in determining the viability of possible wind farm locations is Remote Sensing for Wind Resource Assessment.
This complex procedure includes using remote sensing technologies to assess wind characteristics at different altitudes above the suggested location, such as LIDAR (Light Detection and Ranging) or SODAR (Sonic Detection and Ranging).
The precise interpretation of the data gathered by these distant sensors, which offers a thorough grasp of the wind’s speed, direction, and turbulence, is a technological cornerstone of this strategy.
Data Security and Privacy challenges
Data privacy protection becomes more difficult as linked systems grow and cloud computing becomes more widely used. Businesses face a difficult task in making sure that data, particularly personally identifiable information (PII), is managed appropriately and in accordance with strict privacy laws.
The right method entails putting privacy-by-design principles into practice, which integrates data protection safeguards into the system and application architecture itself. In order to protect personal identities and respect privacy requirements, this comprises anonymization methods, pseudonymization, and other privacy-enhancing technologies.
This vast data ecosystem presents a technological challenge for ensuring end-to-end security, requiring constant monitoring, threat detection, and quick reaction methods. This entails putting in place proactive steps to find and eliminate such threats, anomaly detection algorithms, and sophisticated intrusion detection systems.
Create your Custom AI tool to Improve your Wind Turbine Process with papAI
Thanks to papAI‘s innovative capabilities, businesses can now unlock the full potential of wind turbine operations and build custom AI solutions that are suited to their specific needs.
By using papAI platform, wind energy companies may make use of artificial intelligence’s transformational potential to improve and optimize a range of aspects of their turbine operations.
The creation of specialized tools that can optimize energy production plans, automate predictive maintenance, and apply adaptive control mechanisms is made easier by this adaptable AI platform.
papAI offers a flexible framework that enables organizations to drive their way towards unheard-of levels of efficiency and performance in the wind energy sector, whether it is through the improvement of problem detection algorithms or the development of customized condition monitoring systems.
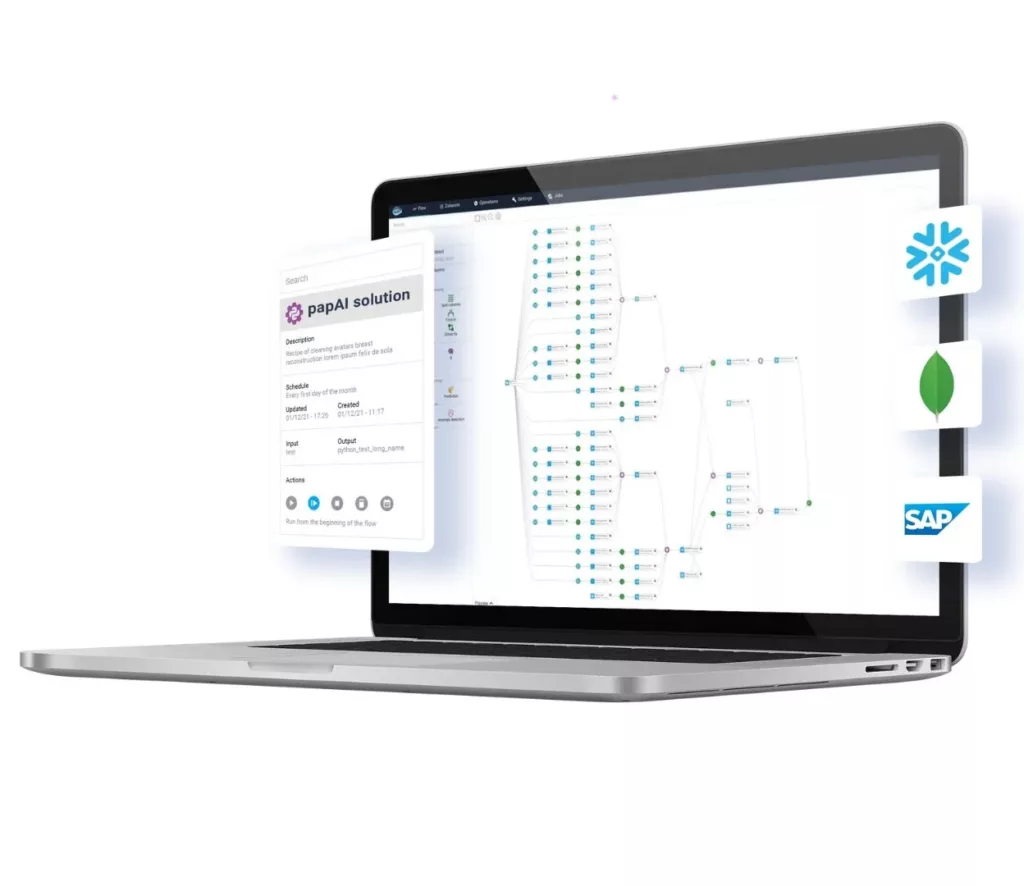
When wind turbines that could produce energy were developed in the late 19th century, it was a revolutionary step forward. Yet wind energy didn’t become widely accepted as a competitive alternative to conventional power sources until the later part of the 20th century.
According to GWEC Market Intelligence, the forecast for total wind power capacity additions during this timeframe has been revised upward by an impressive 143 gigawatts (GW), reflecting a significant 13% year-over-year (YoY) adjustment.
Leveraging AI in wind energy brings forth numerous advantages, including heightened efficiency and output optimization, informed decision-making based on data, seamless remote monitoring and control, and significant cost savings.
The applications in AI optimization for wind turbines encompass Predictive Maintenance, Fault Detection and Diagnostics, Energy Forecasting, Weather Simulation, and Condition Monitoring, alongside Wind Resource Assessment.
Interested in discovering papAI?
Our AI expert team is at your disposal for any questions
“DATATEGY EARLY CAREERS PROGRAM” With Samar CHERNI
“DATATEGY EARLY CAREERS PROGRAM” With Samar CHERNI Hello, my name...
Read MoreAI Origins: Yann LeCun
AI Origins: Yann LeCun Welcome to ”AI Origins “ series....
Read MoreThe Rise of Humanoid Robots: Progress and Challenges
The Rise of Humanoid Robots: Progress and Challenges Some humanoid...
Read More